Jan 22, 2015
by
Erica Baranski
Excitement in the air
My generation – the 20-something, millennial generation — has led technological advancement that has had an unprecedented impact on business, medicine, politics, and even social interactions. Technology is in a constant state of advancement and if you’re plugged in, you can easily get swept away. There is an overwhelming feeling that we are living in an age where ‘it’s all happening’, and in ways it has never happened before.
I’ve been thinking about this state of mind in the context of the current “reproducibility crisis” (Pashler & Wagenmakers, 2012). I recently asked a few (more established) social psychologists if this feeling is based on actual innovation and change or if it’s just a product being young in the field. They emphatically agreed that now is different than any other time in psychology and things really are changing; the air of excitement is real.
Crowdsourcing science
In the midst of the reproducibility crisis, crowdsourcing science has emerged as a means to produce transparent science. With each large-scale, crowdsourcing project, most notably, the Reproducibility Project - Psychology and Cancer Biology, Many Labs 2 and 3, and CREP, the benefits of such methodology are made clear. Sharing the scientific process with other researchers from different institutions enables those outside the primary lab to reap the rewards of the project. The many contributors of these projects have, in their own right, some sense of ownership of the project. For the Reproducibility Project – Psychology (RP-P), collaborators not only are helping the lead researchers answer meta-scientific questions, but are also involved in the excitement that the project has created in the field.
As a contributor to the RP-P, I feel directly involved in this huge open-science movement. This involvement is one of the reasons the reproducibility conversation has had so much momentum over the last couple of years. Crowdsourcing science in conjunction with projects explicitly devoted to improve the integrity and transparency of science will keep the spark alive.
The lab I work in at the University of California – Riverside is utilizing crowdsourcing to spread the benefits of collecting rich and big data. The International Situations Project and International Personality Project are cross-cultural studies in which collaborators from 19 and 13 countries, respectively, have managed the data collection process from a combined 7,454 participants worldwide. We’ve also partnered with Psi Chi and Psi Beta to crowdsource undergraduates and collect data from 13 colleges and universities across the US (Grahe, Guillaume, & Rudman, 2013). Generally, we’re interested in how people experience a situation and how behavior and personality informs, and is informed by, these experiences. To do this, we had our collaborators ask their participants to log on to the study’s website, answer the question “What were you doing at 7:00 pm the previous night?” and then evaluate the situation, their behavior and their personality using Q-sort methodology. The comparison of a large number of cultures along common personality variables is sure to yield important scientific "news" regardless of what is found. With these projects we are aiming to quantify the differences in personality and situations among cultures. Though this research is exploratory in nature, we expect to gain insight into the degree to which behavior, situations, and personality vary both between and within cultures.
In line with the crowdsource methodology’s mission, our many (33+) collaborators have the opportunity to analyze the wealth of data they helped collect to answer important psychological questions. Indeed, a few have already made waves in the field in doing so (Rauthmann et al., 2014). Each ISP/IPP contributor feels (like I do as an RP-P contributor) well-deserved ownership for the project, and shares in the feeling of excitement associated with the project’s analytical and theoretical possibility.
The future of crowdsourced science
Recently, I have had the unique opportunity to use open-source software to expand these projects. Over this past summer I worked as a developer intern at the Center for Open Science (COS) in Charlottesville, VA. COS is a non-profit whose mission is to improve the integrity, openness and reproducibility across all scientific disciplines. To support this mission, COS has developed an online tool called the Open Science Framework (OSF) to enable scientists to maintain productive collaborations, publicly display their methods and data, and encourage the growth of open-science conversation. My experience led me to two major intellectual accomplishments: understanding how to build online tools that make crowdsourcing projects possible, and instilling a new passion in me that will inform all of my future projects. I have begun to appreciate how tools like the OSF and methods like crowd-sourcing can aid in pushing the reproducibility movement forward.
Despite the use of crowdsourcing methods in both projects, the Reproducibility Project and the International Situation (and Personality) Project have distinct scientific goals and it is this distinction that illuminates the scope of scientific endeavors crowdsourcing and technology like the Open Science Framework can facilitate. For example, RP-P utilizes various labs’ resources around the world in order to generalize answers to meta-scientific questions. ISP, on the other hand, recruits its US and international researchers to establish strong scientific connections and lend unique theoretical insights on the massive amount of data collected. Both projects use crowd-sourced data. Both projects intend to strengthen the generalizability power with use of these data. Both projects use the Open-Science Framework to display the methods, materials, and data to encourage the openness of the projects. Yet their research goals exist on two different, yet equally important scientific planes.
The future of crowdsourced science is a bright one. While metascientific projects like RP-P and Many Labs 2 and 3 have illuminated the many benefits crowdsourcing has on science, the projects going on in my lab use this method to involve many scientists in theoretically novel projects. And we do so cross-culturally. The International Situations and Personality Projects offer a unique opportunity to the open-science community to reap the rewards of a project with so much potential for further questions.
It’s all happening
Although I appreciate that some of the excitement around psychological research is a product of being young and new in the field, there is an undeniable sense that things are changing in meaningful ways. Conducting research that uses open-source technology and crowdsourcing methodology will ensure that I am directly involved. It’s important to harness this excitement and continue to establish innovative ways to utilize these crowdsourcing methods. To involve many in the scientific process – no matter what the end goal is – and utilize technology that is constantly pushing forward and carrying scientific integrity and value along with it.
References
Pashler, H., & Wagenmakers, E. J. (2012). Editors’ Introduction to the Special Section on Replicability in Psychological Science A Crisis of Confidence?.Perspectives on Psychological Science, 7(6), 528-530.
Grahe, J. E., Guillaume, E., & Rudmanm, J (2013). Students collaborate to advance science: The International Situations Project. CUR Quarterly-Online, 34 (2), 4-9.
Rauthmann, J. F., Gallardo-Pujol, D., Guillaume, E. M., Todd, E., Nave, C. S., Sherman, R. A., & Funder, D. C. (2014). The Situational Eight DIAMONDS: A taxonomy of major dimensions of situation characteristics.
Nov 26, 2014
by
Jon Grahe
Do you follow me on Twitter, are we Facebook friends? If so, you might know that my pictures almost always include me in a COS t-shirt. That is unless I’m promoting Little Jerry’s, my local burgers and breakfast joint that I also provide free endorsements for. This past summer, I traveled to Psi Chi and Council for Undergraduate Research meetings plus a family visit for three weeks. I only had 6 shirts in my carryon luggage, and there were four colors of COS t-shirts. I still wear my COS t-shirts at least weekly, and on any occasion where a new audience may have a chance to ask me about COS and various aspects of open science, and I try to make sure I post a picture of it on Facebook and Twitter. In the scientific revolution, I like to think of myself as a bard; but my colleagues might be more likely to label me as a clown.
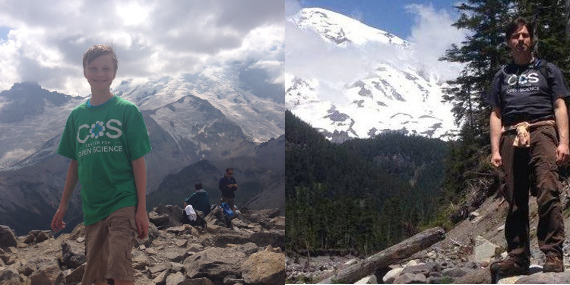
My mother-in-law is starting to think that something is wrong with me. What do I get out of it? Maybe you are starting to wonder too; more likely you don’t really care. However, I think you should, and I welcome competition from more popular, more creative people to take the title as “most variously photographed COS t-shirt wearer” in 2015. I think the challengers should also identify a better title for the winner. So I challenge you to become more active, personal advertisers of the COS specifically and open science generally.
Reasons to Highlight the COS
The COS is about a movement, not a person or a product; though they have products I often try to sell. More accurately, I should describe their products as give-aways. Their primary give-away, the OSF website (which makes scientific workflow easier and more transparent or kept private) and is ever improving. Their other products (Open Science Badges, opportunities to contribute to meta-science projects, the OSC Blog) are similarly valuable and worth investigating. Throughout the fury about whether replication science is valuable or not, I predict that the COS will maintain a focus on methods of improving science and encouraging the positive revolution of science. I like the vision of a Scientific Utopia posited by Brian Nosek and colleagues. What role will you play in achieving that phase of our science?
Conversations From T-shirts
When considering between your COS t-shirt or other clothing options, or when considering what to talk about with your friends, family, and social network, consider the following. Social promotion of the COS benefits other sciences more than psychology. Although I have introduced some peers and students to the COS by bringing them to the COS booth at conferences last year, I think my local market is saturated. Someone should study the social psychology population about their understanding of the scientific revolution and the practices that are being employed. So much of it is happening around us. However, it is other scientists, administrators, and young people in the general public that I most frequently engage in conversation about the t-shirts and why I wear them all the time.
In these brief conversations, I talk about Psychology’s Crisis of Confidence and the importance and potential of Replication Science. I tell them about Ioannidis and that the problems extend to anywhere there is publication bias and entrenched methodology and status quo. I explain solutions offered by Open Science and the COS. My own personal agenda also includes a monologue about the potential contribution of undergraduates, to improving scientific discourse. Depending on the audience and the conversation, I may also talk about the dark side of open science. I explain my frustration with the status quo and the resistance I have witnessed to Replication Science. If they get interested, I suggest they look for phrases like “replication bully” or “replication science” and review the public discourse of the leaders in psychology. I tell them that while bullying is a potential problem, I think that the claim is inverted in this case. I invite them to draw their own conclusions and I encourage them to embrace open science where they can.
Most recently, I got a miniscule amount of Twitter activity wearing one of my COS t-shirts in a costume as a Replication Bully. I loved the costume. I included Open Science Badges as facepaint and drew them on my shield. I fashioned a nasty staff and joined my family medieval fantasy theme. Throughout the night, I probably explained that costume 25 times to peers at one event and then a host of younger people at another. Only a few prior students understood.
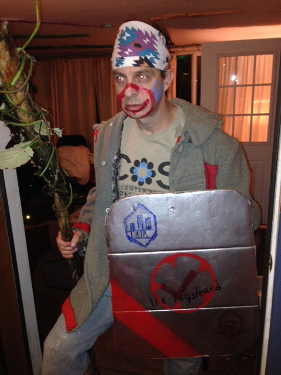
Many party goers ignored it completely, and some asked questions. On Twitter, I made some pithy statements about open science and the evils of the Replication Bully. What I hope is that some of the witnesses go visit the COS or talk about Open Science with their peers. Though this conversation is nauseating with each other, students and the general public seem to be interested and can help reach across scientific disciplines. Consider the disciplines impacted at your host institutions.
Halloween demands villains, and I think the Replication Bully is a villain. Bullying should be avoided, and the replication bully should not be welcome in open science circles. However, as a caricature of this discussion which still continues, it can help identify what should be avoided. Dr. Schnall suggests some parameters that should be avoided, and there are likely others. I therefore invite conversation when I wear my t-shirts, and allow the listener to draw conclusions. I want to keep the replication bully away and identifying these characteristics that are not tolerable is critical to Scientific Utopia. I explain how mistakes can be made and were made, and how they could and should be fixed. I have no shortage of opinions. At the core is the need to focus on open science principles. My experience with the COS sponsored crowd-sourcing science projects is that they always strive to achieve these principles.
Is the COS a valuable contribution to our field? Go visit their website and follow their work. There is no hurry, unless you want to miss out on an exciting part of the scientific revolution. The Reproducibility Project for all its limitations due to wide scope and challenging feasibility was a thoughtful precursor to the various Many Labs Projects. As I edit the undergraduate version of crowdsourcing science in psychology (see the Collaborative Replications and Education Project), I follow their lead. I try to maintain a similar level of quality, but it isn’t easy. They were nimble until just before data collection began, and responded to feedback and answer questions. They are also well-resourced both in their crowd of collaborators, and their collaboration with the COS. The excitement that I felt while working on the methodology summaries this summer as they prepared the pre-registered report was positive and refreshing and inviting. I wished I could do more, but there are only so many hours in a day. As I look to the future, I expect these projects will only continue to diversify. I hope they continue to answer interesting and important questions in our field.
But I think that discussion is critical and if there are criticisms of the Many Labs 1, 2, or 3 methodology, it should be reported. I predict that it will likely be addressed in Many Labs 4, 5, ..., k. I welcome the Many Labs teams into my lab, and my classroom, and I welcome their vibrant introduction of openness and persistence into our science. For the CREP, the study selection resulted from identifying studies based on impact factor and feasibility for undergraduates to complete, but we recently started soliciting requests for favorite or classic studies. How does study selection impact bias of effect estimates? I bet some of those replication science hordes (a revolutionary population filled with normally distributed personalities and interpersonal styles no doubt) will identify methods of testing this and suggesting further tests and corrections.
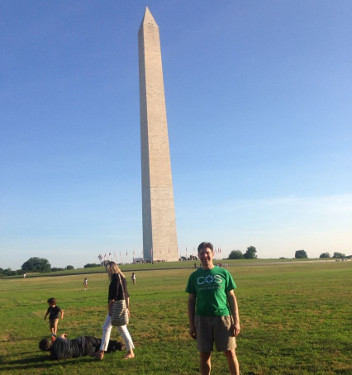
When I planned my Halloween costume, I thought about dressing as a hero and imitating some replication science icons, but it is a time for horror so I chose a villain. Thanksgiving is coming; this blog is not likely to be live by then, but if I have the opportunity, I’ll pose with a COS tshirt and try to represent Thanks. I’ll tweet it and post it on FB. I’ll hope that it is ingenious enough to peek some interest in the COS and meta-science projects generally and the CREP indirectly. My next opportunity is the holiday season, and then conference season will ensue. In each case, I will expose as many people as possible to open science, either through personal connection or via social media. My goal will be trying to visually connect my renewed excitement in our field with the organization that will most likely bring about the improvement many of us have craved for decades at least.
Why not embrace replication science opportunities? I saturate my social network with images of Open Science and invite them to ponder that question. While I am not a social media star, some of you might be. Pose in your COS tshirt, show the love, increase the impact and help brighten rather than darken our sciences’ future. I would like to see more interesting poses and locations than my limited imagination brings.
If you prefer to demonstrate your effort by working hard and quietly advancing open science principles, so be it. If you engage in replication bullying, I will distance myself from you. If you are fun and lively and fancy yourself a bard, but might sometimes act like a clown, expand the conversation about open science beyond psychology. As with other social movements, change will come slowly and it is likely the next generation that will see the largest gains from the current revolution in methods and scientific values. In the meantime, I’ll go put my t-shirts in the laundry and think about what they may look like on a turkey.
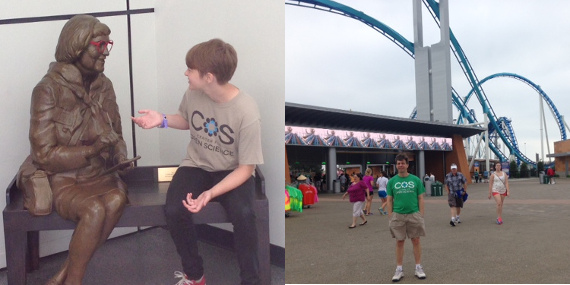
Nov 24, 2014
by
Robert Diener
The Noba Project is an open psychology education initiative of the Diener Education Fund. Noba was envisioned as an open and free substitute to traditional intro-to-psychology materials that cost anywhere from $100 to $200 for a single book.
The primary goals of Noba are:
- To reduce financial burden on students by providing access to free educational content
- To provide instructors with a platform to customize educational content to better suit their curriculum
- To present free, high-quality material written by a collection of experts and authorities in the field of psychology
The Noba Project consists of a website (www.nobaproject.com) where instructors, students, or independent learners can find a large and growing collection of learning modules written by well-known instructors and researchers. The website allows users to mix and match those modules into any order they like and publish a custom textbook that is preserved in each user's personal “Library” on the Noba website and that can be downloaded and distributed in PDF format as well.
Noba has also curated the various modules into a number of “Ready-Made” textbooks that instructors can use as-is or edit to suit their needs. One example is the Noba book “Discover Psychology”, which was built to match the scope and sequence of standard Intro-to-Psychology courses. All Noba materials are licensed under the Creative Commons CC BY-NC-SA license. Users can freely use, redistribute, re-mix, and re-purpose the Noba content.
The Diener Education Fund is co-founded by Drs. Ed and Carol Diener. Ed is the Joseph Smiley Distinguished Professor of Psychology (Emeritus) at the University of Illinois. Carol Diener is the former director of the Mental Health Worker and the Juvenile Justice Programs at the University of Illinois. Both Ed and Carol are award-winning university teachers.
Is there a role for undergraduates and graduate students to contribute?
We love students and want to engage them. This is why we created the Noba Video Award. Each year we award 10 thousand dollars for high quality short videos related to a specific Noba content area. Last year it was "memory" and we received more than 50 submissions from around the world. This year our topic is "social influence" and we hope to receive even more submissions. We believe that this presents an opportunity for students to engage in their learning in a more creative and active way. We also display top videos and integrate winning videos into our actual content modules. We also invite graduate and undergraduate students to write guest newsletter and blog posts for us. This is an opportunity for students to have their voice heard by their peers around the world.
Another way we’ve included grad students is that when Noba first started, at the beginning of the writing process we asked our well-known authors if they had deserving graduate students that they wanted to work with and many of them were very receptive to that suggestion. A good number of our modules have graduate students as second authors. It meant a lot to us to be able to help give these younger researchers a chance to be involved in the writing and publication process. In the future as we produce new modules for Noba we'll encourage the same kind of collaboration.
How often are the modules updated/edited?
Because of our digital format we are able to make updates large and small as needed. On the smaller side we make regular updates to modules including new links that may recently have become available, or dropping in new examples where we think they might be helpful. We invite feedback from our users and we often get small recommendations for illustrations, figures, subtopics, etc. We try to make these types of revisions very quickly.
The idea of making larger changes that keep pace with the research is a very difficult issue. The truth is most textbooks provide a broad overview and simply do not have room to dive deeply in new developments. Traditionally, textbook publishers have dealt with this by updating pop culture references, graphics, and adding brief mentions of newer research. Again, because of our digital format, we are lucky to be able to make major revisions in multiple ways. First, we can create whole new modules without concern for overall book length or publishing costs. For example, we have a whole module on the “Biochemistry of Love." This is a niche topic that draws heavily from contemporary research and yet we can easily include it. We also make major changes by writing whole new modules that represent content at the 100/200 or the 300/400 level. For example, David Lubinski wrote a very advanced module on "Intellectual abilities, Interest, and Mastery." This will be particularly interesting to students who want more challenge, sophistication, and depth. For more introductory level material, on the other hand, we have a basic unit on "Intelligence" that is comparable to what you would find in traditional intro psych textbooks.
With regards to updating our editorial strategy is this: if we receive feedback about suggestions for small changes we try to revise immediately. If there are exciting breakthroughs we try to make mention of these emerging studies or new directions within our existing modules. Finally, for areas for which there is a cascade of new findings we entertain the idea of creating all new modules.
What subfields of psychology is NOBA interested in exploring next?
This is an excellent question. If our resources were unlimited we would build out complete collections for clinical, developmental, biological, statistical and social psychology. In reality, we have to make careful decisions about what we invest in. We don't want to develop too much new material without first evaluating the strength of our current material. Ideas we have discussed recently include: developing more interactive learning for the modules we currently have, developing more material regarding actual careers in psychology, and developing more in-depth material in cognitive/neuroscience and social/personality.
One of the most exciting developments for us was the realization that no matter how good the content is many instructors will be slow to adopt it because they need a suite of materials to help them teach it. As a result we invested heavily in creating power point presentations, test bank items, and a comprehensive instructor manual. All available free of charge, of course!
To what extent can Noba contribute to open science/teaching in other countries? The major textbooks we get tend to have european/international editions where at least the anecdotes are adjusted so that non-american students could relate to them better.
We are aware that we Americans can be-- well-- American centric in our views. We have attempted to side step that problem by using examples that are more global in nature, reporting measurements using the metric system, and inviting non-American authors to help with everything from our test bank items to our modules. At the same time some of our authors have probably used examples that in some cases are more recognizable to North American students.
What we would love to see happen is for users outside the US to create new versions of Noba content to better suit their local context and then put that material back into the Creative Commons for others to use and perhaps build on again. That was one of the most important reasons for choosing an open CC license for Noba that allows derivatives. It would be impossible for us to customize Noba to suit the most specific needs of users everywhere. But local instructors do know what will work best for their students and are free to take Noba as the foundation and then iterate to make a better learning experience that reflects their local situation. And we encourage instructors in the US to do this as well. We trust instructors to make good choices about ways to add on to Noba to benefit their students wherever they are.
In the end, Noba can be thought of as more than a text-book substitute. It represents a trend toward open educational resources. While the primary aim of Noba is to save students money and remove obstacles to education there is a secondary aim as well. By using the virtues of the digital medium and by paying attention to the latest research on teaching and learning we have the opportunity to fundamentally reimagine what instructional materials are.
Nov 19, 2014
by
Gustav Nilsonne
The replication drive in psychology continues to inspire debate. Simone Schnall has recently published a set of views which I think has potential to helpfully advance a conversation about replication research. In her discussion, one analogy struck me as particularly interesting: that to clinical trials. Schnall suggests that crowdsourced replications of clinical trials would be absurd. Surely, we can’t have patients organizing themselves into trials spontaneously, buying over-the-counter drugs, and reporting their outcomes online?
Possibly not. But we can have patients and doctors working together to do it, and for a wide range of clinical questions. Ideally, if there is uncertainty about what treatment would be best for me, my physician should key in my information into a computer and receive an immediate offer to randomize me to either of several reasonable treatment options. My health outcomes would then be automatically transferred into a clinical outcomes registry. Once enough patients have been randomized and results are conclusive, we will know which treatment is best, and then we can move on to the next question and keep randomizing. What I have just described is a randomised registry clinical trial. These trials can and will be implemented step by step into health systems that are able to monitor outcomes well.
Randomized registry trials can be used not only to test new treatments. They can also replicate clinical trials cheaply and easily. And we sorely need it. Think about it: Randomized controlled trials, in spite of ever-present challenges and limitations, tend to provide reasonably good evidence for the effect of a drug among the patients in the trial. But are you like those patients? Maybe the trial was done 20 years ago. Maybe the trial included only men, and you are a woman. Maybe you have another disease besides the one for which you are taking this drug. To catch moderating factors like these we need another trial. There is no end. To keep improving, we must keep randomizing, keep replicating, and keep extending our clinical questions.
Thus, randomized registry trials can be thought of in a sense as crowdsourced replications. They can be used for direct replications to improve estimates of treatment effects when evidence is inconclusive. They can also be used to verify the external validity of concluded clinical trials, i.e. to test whether a treatment really works in other people, in other places, and in this day and age. Compared to regular clinical trials, they can be a cheap and effective way to improve the evidence base for shared decision making.
Schnall advances two main counterarguments. First, she worries that patients’ confidence in drugs may be undermined. In my opinion, that would be likely to happen mostly if confidence was too high in the first place. If we show that another treatment option has stronger benefits, we will have done the patients a great service. They can then discuss their treatment options with their physicians again, and benefit from a new incremental increase in survival, quality of life, and/or decrease in costs. Here is a direct analogy to basic research. If we can succeed in undermining confidence in results that are not true, then the scientific community and the general public will benefit.
Schnall also suggests that pharmaceutical companies will not like to have their results replicated, because it may harm their reputation if the replication fails. I hope it is true that pharmaceutical companies’ concern for their reputation is a factor that helps to keep them from making unsupported claims about their products. But if they did so anyway, patients and physicians have a right to know. It is well known that industry-sponsored clinical trials have considerable publication bias, leading to inflated effect estimates. Patients of flesh and blood are harmed every day as a result. Pharmaceutical companies can improve their reputation by publishing all their trial data. Again, the analogy to basic research holds. Publication bias and other questionable practices distort the literature to some extent in every field. We researchers can protect our reputations by practicing science openly.
Clinical trials are an interesting model for other kinds of research. The lesson is not to replicate less, nor to avoid crowdsourcing. On the contrary, the lesson is to always keep measuring effects and to continuously update cumulative meta-analytic estimates. These are a few thoughts, which I offer in the hope of contributing to a constructive conversation about replication research.
Gustav Nilsonne, MD, PhD is a researcher at Stockholm University and Karolinska Institutet. You can reach him at gustav.nilsonne@ki.se
Nov 12, 2014
by
Denny Borsboom
This train won't stop anytime soon.
That's what I kept thinking during the two-day sessions in Charlottesville, where a diverse array of scientific stakeholders worked hard to reach agreement on new journal standards for open and transparent scientific reporting. The aspired standards are intended to specify practices for authors, reviewers, and editors to follow in order to achieve higher levels of openness than currently exist. The leading idea is that a journal, funding agency, or professional organization, could take these standards off-the-shelf and adopt them in their policy. So that when, say, The Journal for Previously Hard To Get Data starts to turn to a more open data practice, they don't have to puzzle on how to implement this, but may instead just copy the data-sharing guideline out of the new standards and post it on their website.
The organizers1 of the sessions, which were presided by Brian Nosek of the Center for Open Science, had approached methodologists, funding agencies, journal editors, and representatives of professional organizations to achieve a broad set of perspectives on what open science means and how it should be institutionalized. As a result, the meeting felt almost like a political summit. It included high officials from professional organizations like the American Psychological Association (APA) and the Association for Psychological Science (APS), programme directors from the National Institutes of Health (NIH) and the National Science Foundation (NSF), editors of a wide variety of psychological, political, economic, and general science journals (including Science and Nature), and a loose collection of open science enthusiasts and methodologists (that would be me).
The organizers had placed their bets adventurously. When you have such a broad array of stakeholders, you've got a lot of different interests, which are not necessarily easy to align – it would have been much easier to achieve this kind of task with a group of, say, progressive methodologists. But of course if the produced standards succeed, and are immediately endorsed by important journals, funders, and professional organizations, then the resulting impact is such that it might change the scientific landscape forever. The organizers were clearly going for the big fish. And although one can never be sure with this sort of thing, I think the fish went for the bait, even though it isn't caught just yet.
Before the meeting, subcommittees had been tasked to come up with white papers and proposed standards on five topics: Open standards for data-sharing, reporting of analyses, reporting on research design, replication, and preregistration. During the meeting, these standards were discussed, revised, discussed, and revised again. I don't want to go into the details of exactly what the standards will contain, as they are still being revised at this time, but I think the committees have succeeded in formulating a menu of standards that are digestible for both progressive and somewhat more conservative stakeholders. If so, in the near future we can expect broad changes to take place on these five topics.
For me, one of the most interesting features of the meeting was that it involved such diverse perspectives. For instance, when you talk about data-sharing, what exactly are the data you want people to share? In psychology, we're typically just talking about a 100kB spreadsheet, but what if the data involve Terabytes of neural data? And what about anthropological research, in which the data may involve actual physical artifacts? The definition of data is an issue that seems trivial from a monodisciplinary perspective, but that might well explode in your face if it is transported to the interdisciplinary realm. Similarly, halfway through the meeting, the people involved in clinical trials turned out to have a totally different understanding of preregistration as compared to the psychologists in the room. It was fascinating to see how fields slice up their research reality differently, and how they wrestle with different issues under the same header (and with the same issue under different ones).
Despite these differences, however, I felt that we all did have a clear target on the horizon, and I am confident that the new standards will be endorsed by many, if not all, of the stakeholders present at the meeting. Of course, it is a great advantage that leading journals like Science and Nature endorse higher standards op openness, and that funders like NIH and NSF are moving too. I sometimes have to pinch myself to make sure I am not dreaming, but there is real evidence that, after all these years, actual change is finally taking place: see NIH's endorsement of open science in preclinical research, the Royal Society's new guidelines which make open data mandatory, and the joint editorial on these issues that was simultaneously published by Science and Nature earlier this week. This effectively means that we live in a new world already.
Perhaps the most important thing about this meeting was that it proves how important openness and transparency have become. There are very few topics that could command the agendas of so many leaders in the field to align, so that they can all get together at the same time and place, to spend two days sweating on a set of journal standards. Today, open science is such a topic.
This train won't stop anytime soon.
1 The meeting was organized by Brian Nosek (Center for Open Science), Edward Miguel (University of Berkeley), Donald Green (Columbia University), and Marcia McNutt (Editor-in-Chief of Science Magazine); funded by the Laura and John Arnold Foundation; and sponsored by the Center Open Science, the Berkeley Initiative for Transparency in the Social Sciences, and Science Magazine.
Nov 5, 2014
by
Sean P. Mackinnon
This year, I’ve been teaching a lot more than usual. With that extra teaching comes a lot more grading – and students with concerns about grades. With all the talk about grade inflation lately, I’ve been thinking about HOW grades come to be inflated. While there are certainly political pressures from governments and institutions to produce “successful” students that contribute to grade inflation, I’m thinking about these problems like a psychologist -- a data analyst, really. I’ve learned a lot by working in the open science movement about the psychological processes that underlie questionable research practices and effect size inflation. In this post, I want to draw parallels between grade inflation at universities, and effect size inflation in scientific research. To some extent, I think there are similar psychological processes that contribute to both problems.
Grades have monetary value
High university grades have pragmatic, monetary value to students. At the low end, avoiding failures means not having to pay extra tuition to re-take a course. On the other end, high university grades increase the odds that students will receive scholarships to pay for their education and provides increased access to graduate-level education – which for many individuals, means a higher-paid, more prestigious job. It makes sense then that students will act in their best interest to improve their own grades, while actively avoiding situations that will negatively impact their grades.
Measurement of Academic Success Contains Error
University grades are a psychological construct that (theoretically) measure a student’s capacity for critical thought, knowledge of the subject matter, and analytical skill. Like any psychological construct, teachers need to operationalize this construct with specific measures – in university, that usually means essays, exams, quizzes and assignments. Classical test theory suggests that all measurement is imperfect:
True Score = Measured Score + Error
So, whenever we grade a student’s exam or essay, it approximates their true level of competence with a certain degree of inaccuracy. Maybe you add up the final grade incorrectly, create a poor multiple choice question, or are just in a bad mood when you graded that essay. All of this is error.
Another assumption underlying many statistics is that the residuals are normally distributed. So, sometimes you give students grades that are too high, and sometimes you give grades that are too low, but on average these will tend to cancel each other out in terms of the average grades for your class (assuming randomly distributed errors).
Stacking the deck to get an advantage
The thing is (despite these statistical truisms) most students think that getting a lower grade than they deserve is terribly unfair. Students will tend to work in their own self-interest to get better grades – and the tangible rewards that go along with good grades. Through a few consistently applied tactics, students positively bias the error terms – and in doing so, tend to inflate grades overall. There are at least three primary ways students might do this.
Contesting grades: When students receive a grade that is lower than they deserve – which will happen occasionally due to measurement error in the grading process – students will often try to convince their professor to change that grade. However, I have yet to have a student argue that they should receive a lower grade even though it’s probable that I’ve made those kinds of errors too.
Dropping classes: When students figure they are going to fail a course, then tend to drop the course so it won’t count against their GPA. Thus, the worst instances of performance can be wiped from their record. Of course, students drop classes for all kinds of reasons – however, I think it’s reasonable to say that poor grades increase the odds a student will drop a class (in fact it’s the #1 reason students give for withdrawing from courses in one survey).
Taking easy classes: It’s easier to get a good grade in some classes than others. Sometimes it’s because the material is easier, while other times it’s because the student has prior experience (e.g., a French immersion student taking introductory French in university). While I don’t have hard evidence to prove this, I think most teachers were students themselves long enough to understand that plenty of students are thinking about this when selecting classes.
Because students selectively contest poor grades, and drop courses more frequently when their performance is poor, and actively search for classes where it is easier to get a good grade, this produce a selective positive bias in overall grades.
The parallel with research effect sizes
I think there are clear parallels between grade inflation and inflated effect sizes in science. Like students and good grades, statistically significant results have a pragmatic, monetary value for scientists. Statistically significant results are much more likely to be published in scientific journals. Publications are the currency in academia – numerous, high-impact publications are required to win grants, tenure, and sometimes even to keep your job. It’s not surprising then that scientists will go to great lengths to get statistically significant results to publish in journals – sometimes creating “manufactured beauties” in the process.
In a previous post, I talked about how sampling variation can lead some researchers to find null results, even when the experimenter has done everything right. Imagine you get grant money to run an experiment. It’s well-grounded in theory, and you even go to great lengths to ensure you have 95% power. You collect the data and run the experiment and eagerly check your results … only to find that your hypotheses were not supported. With 95% power, 1 in 20 research studies will end up like this (assuming a frequentist approach which, for better or worse, is dominant in psychology right now). This is a frightening fact of our line of work – there is an element of random chance that permeates statistics. Psychologically speaking, this feels dreadfully unfair. You did good scientific work, but because of outside pressures to prioritize “statistically significant” work with ps < .05, your research will encounter significant challenges getting published. With this in mind it makes sense why many scientists engage in behaviours to “game” this potentially unfair system – the rationale is not that different from those of our students trying to make their way through university with good grades.
Like many in the OSC, I think that major structural changes are needed at the level of the scientific journals to incentivize good research practices, rather than simply incentivizing novel, statistically significant results. When I look at the issues using grades as an analogy, it seems to me that asking scientists to change their questionable research practices without changing the underlying structural problems is a lot like asking students to simply accept a bad grade -- good for the credibility of the institution, but bad for the individual.
Thinking about these two issues together has been an interesting thought experiment both in empathizing with student concerns, and with understanding precisely what it is about the current climate of publishing that feels so unfair sometimes. Like my students, I’d like to believe that hard work should equate to career success – however, the unfortunate truth of science is that there is a bit of luck involved. Even if I disagree with questionable research practices, think I can understand why many people do it. Probability can be cruel.
Oct 30, 2014
by
Ingmar Visser
Why reproducible reporting?
You are running an experiment when a conference deadline comes along; you quickly put together an abstract with 'preliminary findings' showing this or that effect. When preparing the talk for the conference, typically months later, you find a different p-value, F-value, t-value or whatever your favorite statistic was; it may still be significant or as expected, but different regardless; this nags you.
You are preparing a manuscript with multiple experiments, a dozen or so tables and figures and many statistical results such as t-tests, ANOVA's et cetera. During manuscript preparation you decide on using another exclusion criterion for your response time data as well as your participants, all for good reasons. Then, the new semester starts and you are overloaded with teaching duties, and you are only able to start working on your manuscript weeks later. Did you already update figure 5 with the new exclusion criteria? Did you already re-run the t-test on page 17 concerning the response times?
Unfortunately, I myself and many others are familiar with such experiences of not being able to reproduce results. Here, reproducibility refers not to the aspect of experimental results being reproducible. Reproducibility of experimental results has been written about frequently (see discussion here), and serious effort is now put into testing the reproducibility of common results (eg in the reproducibililty project), as well as improving standards when it comes to experimentation (eg by pre-registration, see discussion here). Rather, this post focuses on reproducibility of preparing the data, statistical analyses, producing figures and tables, and reporting of results in a journal manuscript.
During my own training as a psychologist, not much attention was given in the curriculum to standards of bookkeeping, lab-journals, and reporting of results. Standards that are in place focus on the stylistic aspects of reporting, such as that the F in reporting an F-value should be italicized, rather than upright. The APA reporting guidelines concern mostly matters of form and style, such as the exquisitely detailed guidelines for producing references. While such uniformity of manuscripts in form and style are highly relevant when it comes to printing material in journals, those guidelines have not much to say about the contents of what is reported and about how to maintain the reproducibility of analyses.
What is reproducible reporting?
When it comes to reproducible reporting, the goal is to have a workflow from (raw) data files to (pre-)print manuscript in such a way that every step along the way is reproducible, by others, including your future self -- for example, by others who have an interest in the results for the purposes of replication or quality control. It may be useful to have someone particular in mind such as Jelte Wicherts or Uri Simonsohn, both famous for tracking down errors (and worse) in published research -- hence, the goal is to make your work, say, Wicherts-proof. Typically, there are at least three phases involved in (re-)producing reports from data:
-
transforming raw data files to a format that admits statistical analysis
-
making figures and tables, and producing analytical results from the data
-
putting it all together into a report, either a journal manuscript, a presentation for a conference, or a website
To accomplish reproducibility in reporting, the following are important desiderata for our toolchain:
-
Scripting Using a scripting language (as opposed a point-and-click interface) for the statistical analyses, such as R, has the important advantage that analyses can easily be reproduced using the script. Popular statistical analysis packages, such as SPSS, also admit of the possibility of saving scripts of analyses. However, it is not necessary to do so, and hence one can easily omit doing so as it is not the standard way of operating.
-
One tool instead of many R (and possibly other similar statistical packages) can be used to perform all the analytical steps from reading raw data files to analysing data, and producing (camera-ready) figures and tables; again, this is a major advantage over using a combination of text-file editors (to reformat raw data), a spreadsheet program, to clean the raw data, SPSS or otherwise to do the analyses, and a graphical program to produce figures.
-
High quality output in multiple formats A toolchain to produce scientific output should produce high quality output, preferably suitable for immediate publication; that is, it should produce camera-ready copy. It should also produce outputs in different formats, that is, analysis scripts and text should be re-usable to produce either journal manuscripts, web pages or conference presentations. Other important requirements are i) portability (anyone using your source, in plain text format, should be able to reproduce your manuscript, whether they are working on Mac, Linux, Windows or otherwise), ii) flexibility (seamless integration of references and production of the reference list, automatic table of contents, indexing), iii) scalability (the tool should work similarly for small journal manuscripts and multi-volume books), iv) the separation of form and content (scientific reporting should be concerned with content, formatting issues should be left to journals, website maintainers et cetera).
-
Maintain a single source Possibly the most important requirement for reproducible reporting is that the three phases of getting from raw data to pre-print manuscript are kept in a single file; data preprocessing, data analysis, and the text making up a manuscript should be tightly coupled in a single place. A workflow that only comprises a single file and where analytical results are automatically inserted into the text of a manuscript prevents common errors such as forgetting to update tables and figures (did I update this figure with the new exclusion criteria or not?), and, most importantly, simply making typing errors in copying results (the latter is arguably quite a common source of errors and hard to detect, especially so when the results are in favour of your hypotheses).
Fortunately, many tools are available these days that satisfy these requirements to help ensure reproducibility of each of the phases of reporting scientific results. In particular, data pre-processing and data analysis can be made reproducible using R, an open source script based statistics package, which currently has close to 6000 add-on packages to do analyses ranging from simple t-tests to multi-level irt models, and from analysing two-by-two tables to intricate Markov models for complex panel designs.
As for the third requirement, high quality output, LaTeX is the tool of choice. LaTeX has been the de-facto standard for high-quality typesetting in the sciences since its inception in 1986 by Leslie Lamport. LaTeX belongs to the family of markup languages, together with HTML, Markdown, XML, et cetera; the key characteristic of markup languages is the separation of form and content. Writing in LaTeX means assigning semantic labels to parts of your text, such as title, author, section, et cetera. Rather than deciding that a title should be type-set in 12-point Times New Roman, as an author I just want to indicate what the title is. Journals, websites and other media can then decide what their preferred format is for title's in their medium and use the source LaTeX file to produce say PDF, for a journal, or HTML, for a website, output. Separation of form and content is precisely the feature that allows this flexibility in output formats.
The superior typographical quality of LaTeX produced output is nicely illustrated here, showing improved hyphenation, spacing, standard use of ligatures and others. Besides typographical quality, portability, automatic indexing, producing tables of contents, citations and referencing, and scalability were built into the design of LaTeX. See here, for a -- balanced -- discussion of the benefits of LaTeX over other word-processors.
The real benefit of these tools -- R and LaTeX -- comes in when they are used in combination. Several tools are available to combine R with LaTeX in a single document and the main advantage of this combination is that all three phases scientific report production are combined in a single document, fulfilling the fourth requirement. In the following I provide some minor examples of using R/LaTeX.
How to get started with reproducible reporting?
The main tools required for combining statistical analyses in R with LaTeX are Sweave and knitr. Below are the main portals for getting the required -- free! open-source! -- software, as well as some references to appropriate introductory guides.
-
Getting started with R: the R home page, for downloads of the program, add-on packages and manuals; as for the latter, R for psychologists, is particularly useful with worked examples of many standard analyses.
-
Getting started with LaTeX: the LaTeX-homepage for downloads, introductory guides and much much more; here's a cheat sheet that helps in avoiding having to read introductory material.
-
Getting started with Sweave, or alternatively knitr; both provide minimal examples to produce reproducible reports.
If using these programs through their command-line interfaces seems overwhelming to start with, Rstudio provides a nice graphical user interface for R, as well as having options to produce PDF's from Sweave and/or knitr files.
Needless to say, Google is your friend for finding more examples, troubleshooting et cetera.
Minimal R, LaTeX and Sweave example
A minimal example of using R and LaTeX using Sweave can be downloaded here: reproducible-reporting.zip.
To get it working do the following:
- Install Rstudio
- Install LaTeX
- Open the .Rnw file in Rstudio after unpacking and run Compile pdf
Some Markdown examples
Similar to the combination of LaTeX, R in Sweave, Markdown combines simple markup and R code to produce HTML pages as done in this blog. The examples below illustrate some of the possibilities.
To get the flavour, start with loading some data and use 'head(sleep)' to see what's in it:
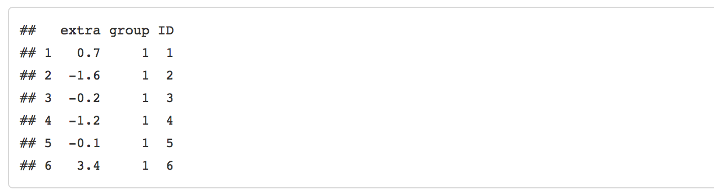
This data gives the 'case' values for application of two types of drug and its influence on hours of sleep. The following plots the means and standard deviations of the two types of treatments:
plot(extra~group,data=sleep)
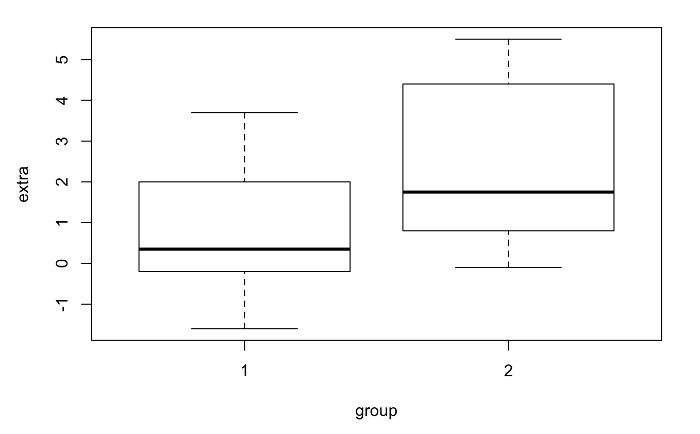
This data set is the one used by Student to introduce his t-test, so it's only fitting to do such a test on these data here:
t.test(extra~group,data=sleep,paired=TRUE)
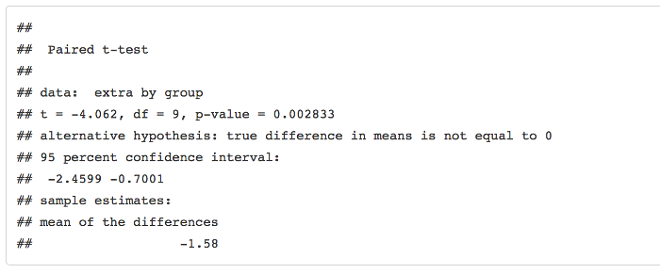
There is much more to say about reproducibility in reporting and statistical analysis than a blog can contain. There are several recent books on the topic for further reading:
Gandrud, C. (2013). Reproducible research with R and RStudio. CRC Press.
Stodden, V., Leisch, F., & Peng, R. D. (Eds.). (2014). Implementing Reproducible Research. CRC Press.
Xie, Yihui. Dynamic Documents with R and knitr. CRC Press, 2013.
... and an online course here.
There is a world to win in acceptance of reproducible research practices, and reproducible reporting should be part and parcel of that effort. Such acceptance not only requires investment on the side of scientists and authors but also on the side of journals, in that they should accept LaTeX/R formatted manuscripts. Many psychometrics and mathematical psychology journals do accept LaTeX, as well as Elsevier, who provide explicit instructions for submitting manuscripts in LaTeX, and so does Frontiers, see here. Change in journal policy can be brought about by (associate) editors: if you have such a position make sure that your journal subscribes to open-source, reproducible reporting standards as this will also help improve the standards of reviewing and eventually the journal itself. As a reviewer, one may request to see the data and analysis files to aid in judging adequacy of reported results. At least some journals, such as the Journal of Statistical Software, require the source and analysis files to be part of every submission; in fact, JSS, accepts LaTeX submissions only, and this should become the standard throughout psychology and other social sciences as well.
Oct 24, 2014
by
Shauna Gordon-McKeon, Sheila Miguez
In celebration of Open Access Week, we'd like to share two pieces of writing from open access advocates who faced or are facing persecution for their efforts towards sharing knowledge.
The first is a letter from Diego A. Gómez Hoyos. Gomez is a Colombian graduate student studying biodiversity who is facing up to eight years in prison for sharing a research article. He writes:
The use of FLOSS was my first approach to the open source world. Many times I could not access ecological or statistical software, nor geographical information systems, despite my active interest in using them to make my first steps in research and conservation. As a student, it was impossible for me to cover the costs of the main commercial tools. Today, I value access to free software such as The R project and QGis project, which keep us away from proprietary software when one does not have the budget for researching.
But it was definitely since facing a criminal prosecution for sharing information on the Internet for academic purposes, for ignoring the rigidity of copyright law, that my commitment to support initiatives promoting open access and to learn more about ethical, political, and economic foundations has been strengthened.
I am beginning my career with the conviction that access to knowledge is a global right. The first articles I have published in journals have been under Creative Commons licenses. I use free or open software for analyzing. I also do my job from a social perspective as part of my commitment and as retribution for having access to public education in both Colombia and Costa Rica.
From the situation I face, I highlight the support I have received from so many people in Colombia and worldwide. Particularly, I thank the valuable support of institutions working for our freedom in the digital world. Among them I would like to acknowledge those institutions that have joined the campaign called “Let’s stand together to promote open access worldwide”: EFF, Fundación Karisma, Creative Commons, Internet Archive, Knowledge Ecology International, Open Access Button, Derechos Digitales, Open Coalition, Open Knowledge, The Right to Research Coalition, Open Media, Fight for the Future, USENIX, Public Knowledge and all individuals that have supported the campaign.
If open access was the default choice for publishing scientific research results, the impact of these results would increase and cases like mine would not exist. There would be no doubt that the right thing is to circulate this knowledge, so that it should serve everyone.
Thank you all for your support.
Diego A. Gómez Hoyos
The second document we’re sharing today is the Guerilla Open Access Manifesto, written by the late Aaron Swartz in 2008:
Information is power. But like all power, there are those who want to keep it for themselves. The world's entire scientific and cultural heritage, published over centuries in books and journals, is increasingly being digitized and locked up by a handful of private corporations. Want to read the papers featuring the most famous results of the sciences? You'll need to send enormous amounts to publishers like Reed Elsevier.
There are those struggling to change this. The Open Access Movement has fought valiantly to ensure that scientists do not sign their copyrights away but instead ensure their work is published on the Internet, under terms that allow anyone to access it. But even under the best scenarios, their work will only apply to things published in the future. Everything up until now will have been lost.
That is too high a price to pay. Forcing academics to pay money to read the work of their colleagues? Scanning entire libraries but only allowing the folks at Google to read them? Providing scientific articles to those at elite universities in the First World, but not to
children in the Global South? It's outrageous and unacceptable.
"I agree," many say, "but what can we do? The companies hold the copyrights, they make enormous amounts of money by charging for access, and it's perfectly legal — there's nothing we can do to stop them." But there is something we can, something that's already being done: we can fight back.
Those with access to these resources — students, librarians, scientists — you have been given a privilege. You get to feed at this banquet of knowledge while the rest of the world is locked out. But you need not — indeed, morally, you cannot — keep this privilege for yourselves. You have a duty to share it with the world. And you have: trading passwords with colleagues, filling download requests for friends.
Meanwhile, those who have been locked out are not standing idly by. You have been sneaking through holes and climbing over fences, liberating the information locked up by the publishers and sharing them with your friends.
But all of this action goes on in the dark, hidden underground. It's called stealing or piracy, as if sharing a wealth of knowledge were the moral equivalent of plundering a ship and murdering its crew. But sharing isn't immoral — it's a moral imperative. Only those blinded by greed would refuse to let a friend make a copy.
Large corporations, of course, are blinded by greed. The laws under which they operate require it — their shareholders would revolt at anything less. And the politicians they have bought off back them, passing laws giving them the exclusive power to decide who can make copies.
There is no justice in following unjust laws. It's time to come into the light and, in the grand tradition of civil disobedience, declare our opposition to this private theft of public culture.
We need to take information, wherever it is stored, make our copies and share them with the world. We need to take stuff that's out of copyright and add it to the archive. We need to buy secret databases and put them on the Web. We need to download scientific journals and upload them to file sharing networks. We need to fight for Guerilla Open Access.
With enough of us, around the world, we'll not just send a strong message opposing the privatization of knowledge — we'll make it a thing of the past. Will you join us?
Aaron Swartz
July 2008, Eremo, Italy
In the past few years, the open access movement has gained momentum as the benefits it provides to individual researchers and to the scientific community as a whole have started to manifest. But even if open access lacked these benefits, we would still be morally obligated to advocate for it, because access to knowledge is a human right.
To learn more about open access efforts, visit the Open Access Week website.
Oct 22, 2014
by
Aaron Goetz
Academic publishing dogma holds that peer reviewers (aka referees) should be anonymous. In the vast majority of cases, however, there are more costs than benefits to reviewer anonymity. Here, I make the case that reviewer identity and written reviews themselves should become publicly accessible information. Until then, reviewers should sign their reviews, as this practice can increase rigor, expose biases, encourage goodwill, and could serve as an honest signal of review quality and integrity.
Why reviewer anonymity solves nothing
The story goes that anonymity frees the reviewer from any reputational costs associated with providing a negative review. Without the cloak of invisibility, reviewers who provided devastating critiques would then become the target of attacks from those debased authors. Vengeful authors could sabotage the reviewer’s ability to get publications, grants, and tenure.
It’s imaginable that these vengeful authors who have the clout to sabotage another’s career might exist, but I’m willing to bet that few careers have been injured or sidelined due primarily to a bullying senior scientist. It’s difficult to say whether the absence of these horror stories is due to a lack of vengeful saboteurs or a lack of named reviewers. If you’re aware of rumored or confirmed instances of a scorned author who exacted revenge, please let me know in the comments section below.
Let’s appreciate that our default is to be onymous1. Without hiding behind anonymity, we manage to navigate our careers, which often includes being critical and negative. We openly criticize others’ work in commentaries, at conferences, in post-publication reviews, and on Facebook and Twitter. Editorships are not the kiss of death, even though their names appear at the bottom of rejection letters. Sure, editors typically have tenure and so you might think that there are no costs to their onymous criticism. But they also still attempt to publish and get grant funding, and their criticism, in the form of rejection letters, probably doesn’t hinder this. Moreover, for every enemy you make by publicly criticizing their work, in the form of post-publication reviews for example, you probably recruit an ally. Can’t newfound allies influence your ability to get publications, grants, and tenure just as much as adversaries?
JP de Ruiter, who wrote an excellent piece also questioning anonymous peer review, offered a simple workaround to the problem of the fearful young scientist criticizing the senior scientist: “Reviewers with tenure always sign their reviews.” This is great, but my fear is that most reviews are written by untenured scientists, so the problems associated with reviewer anonymity will remain with this rule in place. My advice to the untenured and those on, or soon to be on, the job market would be the same: sign all reviews. Even negative reviews that recommend rejection should be signed. Needless to say, negative reviews need to be written very carefully. Drawing attention to flaws, fatal or otherwise, can be done with tact. Speaking tentatively will take the sting out of any criticism. In the review that the author (or public) sees, you can suggest a more appropriate analysis or alterative explanation, but in the private comments to the editor, you can emphasize how devastating these shortcomings are. Keep in mind that reviewers do not need to communicate their recommendation (i.e., accept, revise, reject) in the review that the authors see. In fact, most editors prefer the recommendation be kept separate from the review. This allows them more freedom with their decision. Also, newly minted PhDs and postdocs should keep in mind that there are practices and laws in place so that a scorned search committee member cannot make a unilateral decision.
A second worry is that, without anonymity, reviewers would have to worry about being critical of a known colleague’s (and sometimes a friend’s) work. With anonymity, they’re free to criticize any manuscript and maintain favorable relationships with the authors. But if you’re worried about hurting a colleague’s feelings by delivering an honest and critical review, then you shouldn’t be a reviewer. Recuse yourself. Or maybe you shouldn’t infantilize your colleagues. They’ve probably learned to keep personal and professional relationships separate, and they would surely prefer an honest and constructive review, even if it was accompanied by some short-lived emotional pangs.
A third worry about reviewer transparency might be that it could produce credulous or timid reviews. I don’t see this as a serious threat. Even in the light of onymity, reviewers will still demand good evidence. Identified reviewers will still provide the occasional dismissive, sarcastic, and insulting review. I’m certain of this because of my history of providing brutal, onymous reviews and because of those few that I’ve received. Even with my name signed at the bottom, I’ve written some things that I would find difficult to say to the author’s face. I’m not inappropriate, but I can be frank.
Moreover, the concern that identifying reviewers will lead to overly effusive reviews is alleviated when we appreciate the reputational costs associated with providing uncritical reviews. No one wants their name in the acknowledgements of a worthless paper.
Five benefits of reviewer transparency
1) Encourage goodwill. Obviously, reviewer transparency can curb misbehavior. We’re all well aware that it’s easy to be nasty when anonymity reduces the associated risks. The vileness of many YouTube comments is an obvious example. de Ruiter argues that anonymous peer review not only has the unintended consequence of removing good science from the literature, but it also removes good scientists. I can attest to this, too. One of my former graduate students, having just gone through the peer review process, questioned his future in academia. He expressed that he didn’t want the fate of his career to hinge on the whims of three random people who are loaded with biases and can behave badly without consequence.
2) Get credit. Currently, we don't get credit for reviewing. If it's not related to your research, it's not worth your time to write a review, let alone write a high-quality review. "Opportunity costs!" screams the economist. But if we make reviews archivable, then we can receive credit, and we should be more likely to review. Some retention, tenure, and promotion committees would likely count these archived reviews as forms of scholarship and productivity. Altmetrics—quantitative measures of a researcher’s impact, other than journal impact factor—are becoming more and more popular, and unless journal impact factors are completely revamped, which is unlikely to happen anytime soon, we’ll all be hearing a lot more about altmetrics in the future. Digital-born journals are in a good position to overhaul the peer review process to make it transparent and archivable. F1000Research and PeerJ, for example, have laudable open peer review models.
The flip side of this “getting credit” benefit is that we’ll be able to see who’s free-riding. In a correspondence piece in Nature, Dan Graur argued that those scientists who publish the most are least likely to serve as reviewers. “The biggest consumers of peer review seem to contribute the least to the process,” he wrote. This inverse correlation was not found, however, in a proper analysis of four ecology journals over an 8-year period, but the ratio of researchers’ reviews to submissions could be journal or discipline specific. Bottom line: free-riding could be a problem, and reviewer onymity could help to reduce it.
A journal’s prestige comes primarily from the quality of papers it publishes. And the quality of papers rests largely on the shoulders of the editors and peer reviewers. It follows, then, that the prestige of a journal is owed to its editors and reviewers. Editors get acknowledged. Their names are easily found in the colophon of a print journal and on the journal’s website, but not so for the reviewers’ names. Some journals publish an annual acknowledgment of manuscript reviewers, but because it’s divorced from any content—e.g., you don’t know who reviewed what and how often—it’s largely worthless and probably ignored. Given that the dissemination (and progress?) of science depends on free labor provided by reviewers, they should get credit for doing it. Admittedly, this would introduce complexities, such as including the recommendations of the reviewers. I’d appreciate if I were acknowledged as a reviewer in each paper I review, but only if my recommendation accompanied my name: “Aaron Goetz recommended rejection.” A reviewer’s name, without her accompanying recommendation, in the acknowledgements of a published paper would look like an endorsement, and I know I’m not the only one to recommend rejection to a paper that was subsequently published. Even better, it would not be difficult to link associated reviews to the paper.
3) Accountability. You’ve surely opened up the pages of your discipline’s flagship journal, saw a laughable article, and wondered who let this nonsense get published. Picking the low-hanging fruit, who reviewed Bem’s precognition paper? Some reviewers of that paper, not Eric-Jan Wagenmakers, should be held accountable for wasting researcher resources. Try not to calculate how much time and effort was spent on these projects that set the record straight.
Another benefit that comes from shining light on reviewers would be the ability to recognize unsuitable reviewers and conflicts of interest. I bet a nontrivial number of people have reviewed their former students’ or former advisor’s work. I also have a hunch that a few topics within psychology owe their existence to a small network of researchers who are continually selected as the reviewers for these papers. As a hypothetical example, wouldn’t it be important to know that the majority of work on terror management theory was reviewed by Greenberg, Solomon, Pyszczynski, and their students? Although I don’t think that G, S, P, and their students conducted the majority of reviews of the hundreds of terror management papers, I am highly skeptical of TMT for theoretical reasons. But I digress.
Some colleagues have confessed that, when reviewing a manuscript that has the potential to steal their thunder or undermine their work, they were more critical, were more likely to recommend rejection, and took significantly longer to return their review. This is toxic and is “damaging science” in de Ruiter’s words.
And for those senior researchers who delegate reviews to graduate students, onymity could alleviate the associated bad practices. Senior researchers will either be forced to write their own reviews or engage in more pedagogy so that their students’ reviews meet basic standards of quality.
4) Clarification. Authors would be able to ask reviewers to clarify their comments and suggestions, even if official correspondence between the two is severed due to the manuscript’s rejection. I’ve certainly received comments that I didn’t know quite what to do with. I once got “The authors should consider whether these perceptual shifts are commercial.” Huh? Commercial? Of course, a potential danger is that authors and reviewers could open a back-channel dialog that excludes the editor. I imagine that some editors will read potential danger, while some will read potential benefit. If you’re the former, an explicit “Authors and reviewers should refrain from communicating with one another about the manuscript throughout the review process” would probably do the trick.
5) Increased quality. This is the primary benefit of review transparency. I know that I’m not the only reviewer who has, at some point, written a hasty or careless review. Currently, there are no costs to reviewers who provide careless or poor-quality reviews, but there are serious costs associated with careless reviews, the primary being impeding scientific progress and wasting researcher resources. If we tie reputational consequences to reviews, then review quality increases. This practice might also increase review time, but that’s a cost we should be willing to incur to increase quality and accountability, expose biases, give credit where it’s due, and encourage goodwill.
There’s actually some empirical evidence suggesting that signed reviews are of higher quality than unsigned reviews. Reviewers for the British Journal of Psychiatry were randomly assigned to signed and unsigned groups and provided real reviews for manuscripts, per business as usual. The researchers then measured the quality of reviews and compared them. By most measures, review quality was modestly but statistically better among the signed reviews. These data, however, aren’t as relevant to my argument, because reviewer identity was only revealed to authors of the manuscripts rather than the entire scientific community. Any differences noted between the signed and unsigned groups are likely conservative estimates of what would happen if reviewers’ names and recommendations were publically attached to papers where reputational costs and benefits could be incurred. Another study also examining the effect of reviewer anonymity on review quality did not find any differences between the signed and unsigned groups, but this study suffers from the same limitation as the first: reviewer identity was only revealed to the authors of the manuscripts and did not appear in the subsequent publishing of accepted manuscripts.
Signed reviews could become what evolutionary scientists call honest signals. Honest signals—sometimes referred to as hard-to-fake signals, costly signals, or Zahavian signals—refer to traits or behaviors that are metabolically and energetically costly or dangerous to produce, maintain, or express. We all know the peacock’s tail as the standard example. A peacock’s tail honestly signals low parasite load. Only healthy, high quality males can afford to produce large, bright tail feathers. And many of us learned that the stotting of many antelope species is best understood as an honest signal of quality.
Much in same way that large, bright tail feathers honestly signal health, signed reviews can honestly signal review quality and integrity. Only a reviewer who writes a high quality review and is confident that the review is high quality can afford to sign her name at the bottom of her review. And only a reviewer who is confident that her critical review is fair and warranted can afford sign her name.
It’s easy to write a subpar review; it probably happens every day. It’s not easy, however, to write a subpar review if your name is attached to it. Our desire to maintain our reputation is strong. To illustrate this, I challenge you to tweet or update your Facebook status to this: “CBS is hands down the best network. I could watch it all day.”
Conclusion
I recently reread an onymous review I received from a colleague I deeply respect. His review did not pull any punches, and parts of it would probably be considered abrasive by those who haven’t developed a thick skin. When I received it, I recognized that to give my paper such a review—to dig up those obscure references, to run those analyses, to identify all of the strengths and weaknesses, and to entertain those alternative explanations—he had to get intimate with it. He had to let it marinade in his thoughts before he savored each paragraph, citation, and analysis. Although he ultimately devoured it, it was deeply rewarding to have someone else care that much about my work. And it fills me with gratitude to know who it was that give up their weekend, their time on their own work, or their time with their friends and family. Anything that increases rigor, exposes biases, aids scientific progress, and promotes gratitude and goodwill should at least be considered. And beyond mere consideration, journals should think seriously about examining the differences between signed and unsigned reviews and between public and private reviews. Editors have all they need to examine the differences between signed and unsigned reviews, and editors open to testing an open reviewer system that links reviews to published papers can contact me and others at the Open Science Collaboration.
Footnotes
1 Yes, you’re recognizing onymous as the back-formation of anonymous. Onymous is synonymous with named, identified, or signed.
2 JP de Ruiter, whom I mention few times throughout, wrote a piece that argued the same basic point that I’m trying to here: anonymous peer review is toxic and it should be fixed. Andrew Sabisky alerted me to de Ruiter’s post, and it inspired me to finish writing this piece. Many thanks to both. I encourage you to read de Ruiter’s post. Also, Kristy MacLeod wrote a great post about her decision to sign a review, and her decision to sign seems to be rooted in honest signaling. I recommend you read it, too.
3 Geoffrey Miller wrote and signed the review I referenced in the last paragraph.
4 Special thanks go to Jessica Ayers, Kayla Causey, JP de Ruiter, Jon Grahe, and Gorge Romero who gave comments on an earlier draft of this post. All errors are my own, of course.
Oct 14, 2014
by
Alex Holcombe
Science is sick. How will we know when it's been cured?
Meta-analysis quantitatively combines the evidence from multiple experiments, across different papers and laboratories. It's the best way we have to determine the upshot of a spate of studies.
Published studies of psi (telepathy, psychokinesis, and other parapsychological phenomena) have been submitted to meta-analysis. The verdict of these meta-analyses is that the evidence for the existence of psi is close to overwhelming. Bosch, Steinkamp, & Boller (2006, Psychological Bulletin), for example, meta-analyzed studies of the ability of participants to affect the output of random number generators. These experiments stemmed from an older tradition in which participants attempted to influence a throw of dice to yield a particular target number. As for the old dice experiments, many of the studies found that the number spat out by the random number generator was more often the target number that the participant was gunning for than one would expect by chance. In their heroic effort, Bosch et al. combined the results of 380 published experiments, and calculated that if in fact psychokinesis does not exist, the probability of finding the evidence published was less than one in a thousand (for one of their measures, z = 3.67). In other words, it is extremely unlikely that so much evidence in favor of psychokinesis would have resulted if psychokinesis actually does not exist.
Like many others, I suspect that this evidence stems not from the existence of psi, but rather from various biases in the way science today is typically conducted.
"Publication bias" refers to the tendency for a study to be published if it is interesting, while boring results rarely make it out of the lab. "P-hacking" - equally insidious - is the tendency of scientists to try many different statistical analyses until they find a statistically significant result. If you try enough analyses or tests, you're nearly guaranteed to find a statistically significant although spurious result. But despite scientists' suspicion that the seemingly-overwhelming evidence for psi is a result of publication bias and p-hacking, there is no way to prove this, or to establish it beyond a reasonable doubt (we shouldn't expect proof, as that may be a higher standard than is feasible for empirical studies of a probabilistic phenomenon).
Fortunately these issues have received plenty of attention, and new measures are being adopted (albeit slowly) to address them. Researchers have been encouraged to publicly announce (simply by posting on a website) a single, specific statistical analysis plan prior to collecting data. This can eliminate p-hacking. Other positive steps, like sharing of code and data, helps other scientists to evaluate the evidence more deeply, to spot signs of p-hacking as well as inappropriate analyses and simple errors. In the case of a recent study of psi by Tressoldi et al., Sam Schwartzkopf has been able to wade into the arcane details of the study, revealing possible problems. But even if the Tressoldi et al. study is shown to be seriously flawed, Sam's efforts won't overturn all the previous evidence for psi, nor will it combat publication bias in future studies. We need a combination of measures to address the maladies that afflict science.
OK, so let's say that preregistration, open science, and other measures are implemented, and together fully remedy the unhealthy traditions that hold back efforts to know the truth. How will we know science has been cured?
A Psi Test for the health of science might be the answer. According to the Psi Test, until it can be concluded that psi does not exist using the same meta-analysis standards as are applied to any other phenomenon in the biomedical or psychological literature, science has not yet been cured.
Do we really need to eliminate publication bias to pass the Psi Test, or can meta-analyses deal with it? Funnel plots can provide evidence for publication bias. But given that most areas of science are rife with publication bias, if we use publication bias to overturn the evidence for psi, to be consistent we'd end up disbelieving countless more-legitimate phenomena. And my reading of medicine’s standard meta-analysis guide, by the Cochrane Collaboration, is that in Cochrane reviews, evidence for publication bias raises concerns but is not used to overturn the verdict indicated by the evidence.
Of course, instead of concluding that science is sick, we might instead conclude that psi actually exists. But I think this is not the case - mainly because of what I hear from physicists. And I think if psi did exist, there’d likely be even more overwhelming evidence for it by now than we have. Still, I want us to be able to dismiss psi using the same meta-analysis techniques we use for the run-of-the-mill. Others have made similar points.
The Psi Test for the health of science, even if valid, won't tell us right away that science has been fixed. But in retrospect we’ll know. After the year science is cured, when taking psi studies published that year and after, applying the standard meta-analysis technique will result in the conclusion that psi does not exist.
Below, I consider two objections to this Psi Test.
Objection 1: some say that we already can conclude that psi does not exist, based on Bayesian evaluation of the psi proposition. To evaluate the evidence from psi studies, a Bayesian first assigns a probability that psi exists, prior to seeing the studies' data. Most physicists and neuroscientists would say that our knowledge of how the brain works and of physical law very strongly suggests that psychokinesis is impossible. To overturn this Bayesian prior, one would need much stronger evidence than even the one-in-a-thousand chance derived from psi studies that I mentioned above. I agree; it's one reason I don't believe in psi. However, it's pretty hard to pin down in quantitative fashion and partially explains why Bayesian analysis hasn’t taken over the scientific literature more rapidly. Also, there may be expert physicists out there that think some sort of quantum interaction could underlie psi, and it's hard to know how to quantitatively combine the opinions of dissenters with the majority.
Rather than relying on a Bayesian argument (although Bayesian analysis is still useful, even with a neutral prior), I'd prefer that our future scientific practice, involving preregistration, unbiased publishing, replication protocols, and so on reach the point where if hundreds of experiments on a topic are available, they should be fairly definitive. Do you think we will get there?
Objection 2: Some will say that science can never eliminate publication bias. While publication bias is reduced by the advent of journals like PLoS ONE that accept null results, and by the growing number of journals that accept papers prior to the data being collected, it may forever remain a significant problem. But there are further steps one could take: in open notebook science, all data is posted on the net as soon as it is collected, eliminating all opportunity for publication bias. But open notebook science might never become standard practice, and publication bias may remain strong enough that substantial doubt will persist for many scientific issues. In that case, the only solution may be a pre-registered, confirmatory large-scale replication of an experiment, similar to what we are doing at Perspectives on Psychological Science (I'm an associate editor for the new Registered Replication Report article track). Will science always need that to pass the psi test?