Oct 7, 2014
by
Stacy Konkiel
This article was originally posted on the Impactstory blog.
Yesterday, we announced three winners in the Center for Open Science’s random drawing to win a year’s subscription to Impactstory for users that connected their Impactstory profile to their Open Science Framework (OSF) profile: Leonardo Candela (OSF, Impactstory), Rebecca Dore (OSF, Impactstory), and Calvin Lai (OSF, Impactstory). Congrats, all!
We know our users would be interested to hear from other researchers practicing Open Science, especially how and why they use the tools they use. So, we emailed our winners who graciously agreed to share their experiences using the OSF (a platform that supports project management with collaborators and project sharing with the public) and Impactstory (a webapp that helps researchers discover and share the impacts of all their research outputs). Read on!
What's your research focus?
Leonardo: I’m a computer science researcher. My research interests include Data Infrastructures, Virtual Research Environments, Data Publication, Open Science, Digital Library Management Systems and Architectures, Digital Libraries Models, Distributed Information Retrieval, and Grid and Cloud Computing.
Rebecca: I am a PhD student in Developmental Psychology. Broadly, my research focuses on children’s experiences in pretense, fiction and fantasy. How do children understand these experiences? How might these experiences affect children's behaviors, beliefs and abilities?
Calvin: I'm a doctoral student in Social Psychology studying how to change unconscious or automatic biases. In their most insidious forms, unconscious biases lead to discrepancies between what people value (e.g., egalitarianism) and how people act (e.g., discriminating based on race). My interest is in understanding how to change these unconscious thoughts so that they're aligned with our conscious values and behavior.
How do you use the Open Science Framework in the course of your research?
Leonardo: Rather than an end user of the system for supporting my research tasks, I’m interested in analysing and comparing the facilities offered by such an environment and the concept of Virtual Research Environments.
Rebecca: At this stage, I use the OSF to keep all of the information about my various projects in one place and to easily make that information available to my collaborators--it is much more efficient to stay organized than constantly exchanging and keeping track of emails. I use the wiki feature to keep notes on what decisions were made and when and store files with drafts of materials and writing related to each project. Version control of everything is very convenient.
Calvin: For me, the Open Science Framework (OSF) encompasses all aspects of the research process - from study inception to publication. I use the OSF as a staging ground in the early stages for plotting out potential study designs and analysis plans. I will then register my study shortly before data collection to gain the advantage of pre-registered confirmatory testing. After data collection, I will often refer back to the OSF as a reminder of what I did and as a guide for analyses and manuscript-writing. Finally, after publication, I use the OSF as a repository for public access to my data and study materials.
What's your favorite Impactstory feature? Why?
Leonardo: I really appreciate the effort Impactstory is posing on collecting metrics on the impact my research products have on the web. I like its integration with ORCID and the recently supported “Key profile metrics” since it gives a nice overview of a researcher impact.
Rebecca: I had never heard of ImpactStory before this promotion, and it has been really neat to start testing out. It took me 2 minutes to copy my publication DOIs into the system, and I got really useful information that shows the reach of my work that I hadn't considered before, for example shares on Twitter and where the reach of each article falls relative to other psychology publications. I'm on the job market this year and can see this being potentially useful as supplementary information on my CV.
Calvin: Citation metrics can only tell us so much about the reach of a particular publication. For me, Impactstory's alternative metrics have been important for figuring out where else my publications are having impact across the internet. It has been particularly valuable for pointing out connections that my research is making that I wasn't aware of before.
Thanks to all our users who participated in the drawing by connecting their OSF and Impactstory profiles! Both of our organizations are proud and excited to be working to support the needs of researchers practicing Open Science, and thereby changing science for the better.
To learn more about our open source non-profits, visit the Impactstory and Open Science Framework websites.
Sep 9, 2014
by
Konrad Hinsen
Recently, Shauna Gordon-McKeon wrote about the meaning of replicability on this blog, concentrating on examples from psychology. In this post, I summarize for comparison the situation in computational science. These two fields may well be at opposite ends of the spectrum as far as replication and replicability are concerned, so the comparison should be of interest for establishing terminology that is also suitable for other domains of science. For a more detailed discussion of the issues specific to computational science, see this post on my personal blog.
The general steps in conducting a scientific study are the same in all fields:
-
Design: define in detail what needs to be done in order to obtain useful insight into a scientific problem. This includes a detailed description of required equipment, experimental samples, and procedures to be applied.
-
Execution: do whatever the design requires to be done.
-
Interpretation: draw conclusions from whatever results were obtained.
The details of the execution phase vary enormously from one discipline to another. In psychology, the "experimental sample" is typically a group of volunteers, which need to be recruited, and the "equipment" includes the people interacting with the volunteers and the tools they use, but also the conditions in which the experiment takes place. In physics or chemistry, for which the terms "sample" and "equipment" are most appropriate, both are highly specific to an experiment and acquiring them (by buying or producing) is often the hard part of the work. In computational science, there are no samples at all, and once the procedure is sufficiently well defined, its execution is essentially left to a computer, which is a very standardized form of equipment. Of course what I have given here are caricatures, as reality is usually much more complicated. Even the three steps I have listed are hardly ever done one after the other, as problems discovered during execution lead to a revision of the design. But for explaining concepts and establishing terminology, such caricatures are actually quite useful.
Broadly speaking, the term "replication" refers to taking an existing study design and repeating the execution phase. The motivation for doing this is mainly verification: the scientists who designed and executed the study initially may have made mistakes that went unnoticed, forgotten to mention an important aspect of their design in their publication, or at the extreme have cheated by making up or manipulating data.
What varies enormously across scientific disciplines is the effort or cost associated with replication. A literal replication (as defined in Shauna's post) of a psychology experiment requires recruiting another group of volunteers, respecting their characteristics as defined by the original design, and investing a lot of researchers' time to repeat the experimental procedure. A literal replication of a computational study that was designed to be replicable involves minimal human effort and an amount of computer time that is in most cases not important. On the other hand, the benefit obtained from a literal replication varies as well. The more human intervention is involved in a replication, the more chances for human error there are, and the more important it is to verify the results. The variability of the “sample” is also important: repeating an experiment with human volunteers is likely to yield different outcomes even if done with exactly the same subjects, and similar problems apply in principle with other living subjects, even as small as bacteria. In contrast, re-running a computer program is much less useful, as it can only discover rare defects in computer hardware and system software.
These differences lead to different attitudes toward replication. In psychology, as Shauna describes, literal replication is expensive and can detect only some kinds of potential problems, which are not necessarily expected to be the most frequent or important ones. This makes a less rigid approach, which Shauna calls "direct replication", more attractive: the initial design is not repeated literally, but in spirit. Details of the protocol are modified in a way that, according to the state of knowledge of the field, should not make a difference. This makes replication cheaper to implement (because the replicators can use materials and methods they are more familiar with), and covers a wider range of possible problems. On the other hand, when such an approach leads to results that are in contradiction with the original study, more work must be invested to figure out the cause of the difference.
In computational science, literal replication is cheap but at first sight seems to yield almost no benefit. The point of my original blog post was to show that this is not true: replication proves replicability, i.e. it proves that the published description of the study design is in fact sufficiently complete and detailed to make replication possible. To see why this is important, we have to look at the specificities of computation in science, and at the current habits that make most published studies impossible to replicate.
A computational study consists essentially in running a sequence of computer programs, providing each one with the input data it requires, which is usually in part obtained from the output of programs run earlier. The order in which the programs are run is very important, and the amount of input data that must be provided is often large. Typically, changing the order of execution or a single number in the input data leads to different results that are not obviously wrong. It is therefore common that mistakes go unnoticed when individual computational steps require manual intervention. And that is still the rule rather than the exception in computational science. The most common cause for non-replicability is that the scientists do not keep a complete and accurate log of what they actually did, because keeping such a log is a very laborious, time-consuming, and completely uninteresting task. There is also a lack of standards and conventions for recording and publishing such a log, making the task quite difficult as well. For these reasons, replicable computational studies remain the exception to this day. There is of course no excuse for this: it’s a moral obligation for scientists to be as accurate as humanly and technologically possible about documenting their work. While today’s insufficient technology can be partly blamed, most computational scientists (myself included) could do much better than they do. It is really a case of bad habits that we have acquired as a community.
The good news is that people are becoming aware of the problem (see for example this status report in Nature) and working on solutions. Early adopters report consistently that the additional initial effort for ensuring replicability quickly pays off over the duration of a study, even before it gets published. As with any new development, potential adopters are faced with a bewildering choice of technologies and recommended practices. I'll mention my own technology in passing, which makes computations replicable by construction. More generally, interested readers might want to look at this book, a Coursera course, two special issues of CiSE magazine (January 2009 and July 2012), and a discussion forum where you can ask questions.
An interesting way to summarize the differences across disciplines concerning replication and reproducibility is to look at the major “sources of variation” in the execution phase of a scientific study. At one end of the spectrum, we have uncontrollable and even undescribable variation in the behavior of the sample or the equipment. This is an important problem in biology or psychology, i.e. disciplines studying phenomena that we do not yet understand very well. To a lesser degree, it exists in all experimental sciences, because we never have full control over our equipment or the environmental conditions. Nevertheless, in technically more mature disciplines studying simpler phenomena, e.g. physics or chemistry, one is more likely to blame human error for discrepancies between two measurements that are supposed to be identical. Replication of someone else's published results is therefore attempted only for spectacularly surprising findings (remember cold fusion?), but in-house replication is very common when testing new scientific equipment. At the other end of the spectrum, there is the zero-variation situation of computational science, where study design uniquely determines the outcome, meaning that any difference showing up in a replication indicates a mistake, whose source can in principle be found and eliminated. Variation due to human intervention (e.g. in entering data) is considered a fault in the design, as a computational study should ideally not require any human intervention, and where it does, everything should be recorded.
Aug 22, 2014
by
Alexander Wais
The Berkeley Initiative for Transparency in the Social Sciences (BITSS) will be holding its 3rd annual conference at UC Berkeley on December 11-12, 2014. The goal of the meeting is to bring together leaders from academia, scholarly publishing, and policy which are committed to strengthening the standards of rigor across social science disciplines.
A select number of papers elaborating new tools and strategies to increase the transparency of research will be presented and discussed. Topics for papers include, but are not limited to:
- Pre-registration and the use of pre-analysis plans;
- Disclosure and transparent reporting;
- Replicability and reproducibility;
- Data sharing;
- Methods for detecting and reducing publication bias or data mining.
Papers or long abstracts must be submitted by Friday, October 10th (midnight Pacific time) through CEGA’s Submission Platform. Travel funds will be provided for presenters. Submission can be of completed papers or works in progress.
The 2014 BITSS Conference is sponsored by the Alfred P. Sloan Foundation and the Laura and John Arnold Foundation.
Aug 7, 2014
by
Shauna Gordon-McKeon
If I said, “Researcher A replicated researcher B’s work”, what would you take me to mean?
There are many possible interpretations. I could mean that A had repeated precisely the methods of researcher B, and obtained similar results. Or I could be saying that A had repeated precisely the methods of researcher B, and obtained very different results. I could be saying that A had repeated only those methods which were theorized to influence the results. I could mean that A had devised new methods which were meant to explore the same phenomenon. Or I could mean that researcher B had copied everything down to the last detail.
We do have terms for these different interpretations. A replication of precise methods is a direct replication, while a replication which uses new methods but gets at the same phenomenon is a conceptual replication. Once a replication has been completed, you can look at the results and call it a “successful replication” if the results are the same, and a “failed replication” if the results are different.
Unfortunately, these terms are not always used, and the result is that recent debates over replication have become not only heated, but confused.
Take, for instance, nobel laureate Daniel Kahneman’s open letter to the scientific community, A New Etiquette for Replication. He writes:
“Even rumors of a failed replication cause immediate reputational damage by raising a suspicion of negligence (if not worse). The hypothesis that the failure is due to a flawed replication comes less readily to mind – except for authors and their supporters, who often feel wronged.”
Here he uses the common phrasing, “failed replication”, to indicate a replication where different results were obtained. The cause of those different results is unknown, and he suggests that one option is that the methods used in the direct replication were not correct, which he calls a “flawed replication”. What, then, is the term for a replication where the methods are known to be correct but different results were still found?
Further on in his letter, Kahneman adds:
“In the myth of perfect science, the method section of a research report always includes enough detail to permit a direct replication. Unfortunately, this seemingly reasonable demand is rarely satisfied in psychology, because behavior is easily affected by seemingly irrelevant factors.”
We take “direct replication” to mean copying the original researcher’s methods. As Kahneman points out, perfect copying is impossible. When a factor that once seemed irrelevant may have influenced the results, is that a “flawed replication”, or simply no longer a “direct replication”? How can we distinguish between replications which copy as much of the methods as possible, and those which copy only those elements of the methods which the original author hypothesizes should influence the result?
This terminology is not only imprecise, it differs from what others use. In their Registered Reports: A Method to Increase the Credibility of Published Results, Brian Nosek and Daniel Lakens write:
“There is no such thing as an exact replication. Any replication will differ in innumerable ways from the original. A direct replication is the attempt to duplicate the conditions and procedure that existing theory and evidence anticipate as necessary for obtaining the effect (Open Science Collaboration, 2012, 2013; Schmidt, 2009). Successful replication bolsters evidence that all of the sample, setting, and procedural differences presumed to be irrelevant are, in fact, irrelevant.”
This statement contains an admirably clear definition of “direct replication”, which the authors use here to mean a replication copying only those elements of the methods considered relevant. This is distinct from Kahneman’s usage of the term “direct replication”. Kahneman, instead, may be conflating “direct replication” with “literal replication”, a much less common term meaning “the precise duplication of the specific design and results of a previous study” (Heiman, 2002).
Nosek and Lakens also use the term “successful replication” in a way which implies that not only were the results replicated, the methods were as well, as they take the replication’s success to be a commentary on the methods. However, even “successful replications” may not successfully replicate methods, as pointed out by Simone Schnall in her critique of the special issue edited by Nosek and Lakens:
Various errors in several of the replications (e.g., in the “Many Labs” paper) became only apparent once original authors were allowed to give feedback. Errors were uncovered even for successfully replicated findings.
Whether or not there were methodological errors in these particular cases, the possibility of such errors even when results are replicated remains a possibility, one which is elided by the terminology of “successful replication”. This is not merely a point of semantics, as "successful replications" may be checked less carefully for methodological errors than “failed replications”.
There are many other examples of researchers using replication terminology in ways that are not maximally clear. So far I have only quoted from social psychologists. When we attempt to speak across disciplines we face even greater potential for confusion.
As such, I propose:
1) That we resurrect the term “literal replication”, meaning “the precise duplication of the specific design of a previous study” rather than overload the term “direct replication”. Direct replication can then mean only the duplication of those methods deemed to be relevant. Of course, a perfect literal replication is impossible, but using this terminology implies that duplication of as much of the previous study as possible is the goal.
2) That we retire the phrases “failed replication” and “successful replication”, which do not distinguish between procedure and results. In their place, we can use “replication with different results” and “flawed replication” for the former, and “replication with similar results” and “sound replication” for the latter.
Thus, a replication attempt where the goal was to precisely duplicate materials and where this was successfully done, but different results were found, would be a sound literal replication with different results. An attempt only to duplicate elements of the design hypothesized to be relevant, leading to some methodological questions, yet where similar results were found, would be a flawed direct replication with similar results.
These terms may seem unnecessarily wordy, and indeed may not always be needed, but I encourage everyone to use them when precision is important, for instance in published articles or in debates with those who disagree with you. I know that from now on, when I hear someone use the bare term “replication”, I will ask, “What kind?”
Thanks to JP de Ruiter, Etienne LeBel, and Sheila Miguez for their feedback on this post.
Jul 30, 2014
by
Sebastiaan Mathôt
A little more than three years ago I started working on OpenSesame, a free program for the easy development of experiments, mostly oriented at psychologists and neuroscientists. The first version of OpenSesame was the result of a weekend-long hacking sprint. By now, OpenSesame has grown into a substantial project, with a small team of core developers, tens of occasional contributors, and about 2500 active users.
Because of my work on OpenSesame, I've become increasingly interested in open-source software in general. How is it used? Who makes it? Who is crazy enough to invest time in developing a program, only to give it away for free? Well ... quite a few people, because open source is everywhere. Browsers like Firefox and Chrome. Operating systems like Ubuntu and Android. Programming languages like Python and R. Media players like VLC. These are all examples of open-source programs that many people use on a daily basis.
But what about specialized scientific software? More specifically: Which programs do experimental psychologists and neuroscientists use? Although this varies from person to person, a number of expensive, closed-source programs come to mind first: E-Prime, SPSS, MATLAB, Presentation, Brainvoyager, etc. Le psychonomist moyen is not really into open source.
In principle, there are open-source alternatives to all of the above programs. Think of PsychoPy, R, Python, or FSL. But I can imagine the frown on the reader's face: Come on, really? These freebies are not nearly as good as 'the real thing', are they? But this, although true to some extent, merely raises another question: Why doesn't the scientific community invest more effort in the development of open-source alternatives? Why do we keep accepting inconvenient licenses (no SPSS license at home?), high costs ($995 for E-Prime 2 professional), and scripts written in proprietary languages that cannot easily be shared between labs. This last point has become particularly relevant with the recent focus on replication and transparency. How do you perform a direct replication of an experiment if you do not have the required software? And what does transparency even mean if we cannot run each other's scripts?
Despite widespread skepticism, I suspect that most scientists feel that open source is ideologically preferable over proprietary scientific software. But open source suffers from an image problem. For example, a widely shared misconception is that open-source software is buggy, whereas proprietary software is solid and reliable. But even though quality is subjective--and due to cognitive dissonance strongly biased in favor of expensive software!--this belief is not consistent with reality: Reports have shown that open-source software contains about half as many errors per line of code as proprietary software.
Another misconception is that developing (in-house) open-source software is expensive and inefficient. This is essentially a prisoners dilemma. Of course, for an individual organization it is often more expensive to develop software than to purchase a commercial license. But what if scientific organizations would work together to develop the software that they all need: You write this for me, I write this for you? Would open source still be inefficient then?
Let's consider this by first comparing a few commercial packages: E-Prime, Presentation, and Inquisit. These are all programs for developing experiments. Yet the wheel has been re-invented for each program. All overlapping functionality has been re-designed and re-implemented anew, because vendors of proprietary software dislike few things as much as sharing code and ideas. (This is made painfully clear by numerous patent wars.) Now, let's compare a few open-source programs: Expyriment, OpenSesame, and PsychoPy. These too are all programs for developing experiments. And these too have overlapping functionality. But you can use these programs together. Moreover, they build on each other's functionality, because open-source licenses allow developers to modify and re-use each other's code. The point that I'm trying to make is not that open-source programs are better than their proprietary counterparts. Everyone can decide that for him or herself. The crucial point is that the development process of open-source software is collaborative and therefore efficient. Certainly in theory, but often in practice as well.
So it is clear that open-source software has many advantages, also--maybe even especially so--for science. Therefore, development of open-source software should be encouraged. How could universities and other academic organizations contribute to this?
A necessary first step is to acknowledge that software needs time to mature. There are plenty of young researchers, technically skilled and brimming with enthusiasm, who start a software project. Typically, this is software that they developed for their own research, and subsequently made freely available. If you are lucky, your boss allows this type of frivolous fun, as long the 'real' work doesn't suffer. And maybe you can even get a paper out of it, for example in Behavior Research Methods, Journal of Neuroscience Methods, or Frontiers in Neuroinformatics. But it is often forgotten that software needs to be maintained. Bugs need to be fixed. Changes in computers and operating systems require software updates. Unmaintained software spoils like an open carton of milk.
And this is where things get awkward, because universities don't like maintenance. Developing new software is one thing. That's innovation, and somewhat resembles doing research. But maintaining software after the initial development stage is over is not interesting at all. You cannot write papers about maintenance, and maintenance does not make an attractive grant proposal. Therefore, a lot of software ends up 'abandonware', unmaintained ghost pages on development sites like GitHub, SourceForge, or Google Code.
Ideally, universities would encourage maintenance of open-source scientific software. The message should be: Once you start something, go through with it. They should recognize that the development of high-quality software requires stamina. This would be an attitude change, and would require that universities get over their publication fetish. Because the value of a program is not in the papers that have been written about it, but in the scientists that use it. Open-source scientific software has a very concrete and self-evident impact for which developers should be rewarded. Without incentives, they won't make the high-quality software that we all need!
In other words, developers could use a bit of encouragement and support, and this is currently lacking. I recently attended the APS convention, where I met Jeffrey Spies, one of the founders of the Center for Open Science (COS). As readers of this blog probably know, the COS is an American organization that (among many other things) facilitates development of open-source scientific software. They provide advice, support promising projects, and build networks. (Social, digital, and a mix of both, like this blog!) A related organization that focuses more specifically on software development is the Mozilla Science Lab (MSL). I think that the COS and MSL do great work, and provide models that could be adopted by other organizations. For example, I currently work for the CNRS, the French organization for fundamental research. The CNRS is very large, and could easily provide sustained support for the development of high-quality open-source projects. And the European Research Council could easily do so as well. However, these large research organization do not appear to recognize the importance of software development. They prefer to invest all of their budget in individual research projects, rather than invest a small part of it in the development and maintenance of the software that these research projects need.
In summary, a little systematic support would do wonders for the quality and availability of open-source scientific software. Investing in the future, is that not what science is about?
A Dutch version of this article initially appeared in De Psychonoom, the magazine of the Dutch psychonomic society. This article has been translated and updated for the OSC blog.
Jul 16, 2014
by
Sara Bowman, Mallory Kidwell, and Erin Braswell
Scientific domains vary by the tools and instruments used, the way data are collected and managed, and even how results are analyzed and presented. As advocates of open science practices, it’s important that we understand the common obstacles to scientific workflow across many domains. The COS team visits scientists in their labs and out in the field to discuss and experience their research processes first-hand. We experience the day-to-day of researchers and do our own investigating. We find where data loss occurs, where there are inefficiencies in workflow, and what interferes with reproducibility. These field trips inspire new tools and features for the Open Science Framework to support openness and reproducibility across scientific domains.
Last week, the team visited the Monticello Department of Archaeology to dig a little deeper (bad pun) into the workflow of archaeologists, as well as learn about the Digital Archaeological Archive of Comparative Slavery (DAACS). Derek Wheeler, Research Archaeologist at Monticello, gave us a nice overview of how the Archaeology Department surveys land for artifacts. Shovel test pits, approximately 1 foot square, are dug every 40 feet on center as deep as anyone has dug in the past (i.e., down to undisturbed clay). If artifacts are found, the shovel test pits are dug every 20 feet on center. At Monticello, artifacts are primarily man-made items like nails, bricks or pottery. The first 300 acres surveyed contained 12,000 shovel test pits -- and that’s just 10% of the total planned survey area. That’s a whole lot of holes, and even more data.
Fraser Neiman, Director of Archaeology at Monticello, describes the work being done to excavate on Mulberry Row - the industrial hub of Jefferson’s agricultural industry.
At the Mulberry Row excavation site, Fraser Neiman, Director of Archaeology, explained the meticulous and painstaking process of excavating quadrats, small plots of land isolated for study. Within a quadrat, there exist contexts - stratigraphic units. Any artifacts found within a context are carefully recorded on a context sheet - what the artifact is, its location within the quadrat, along with information about the fill (dirt, clay, etc.) in the context. The fill itself is screened to pull out smaller artifacts the eye may not catch. All of the excavation and data collection at the Mulberry Row Reassessment is conducted following the standards of the Digital Archaeological Archive of Comparative Slavery (DAACS). Standards developed by DAACS help archaeologists in the Chesapeake region to generate, report, and compare data from 20 different sites across the region in a systematic way. Without these standards, archiving and comparing artifacts from different sites would be extremely difficult.
Researchers make careful measurements at the Monticello Mulberry Row excavation site, while recording data on a context sheet.
The artifacts, often sherds, are collected by context and taken to the lab for washing, labeling, analysis and storage. After washing, every sherd within a particular context is labeled with the same number and stored together. All of the data from the context sheets, as well as photos of the quadrants and sherds, are carefully input into DAACS following the standards set out in the DAACS Cataloging Manual. There is an enormous amount of manual labor associated with preparing and curating each artifact. Jillian Galle, Project Manager of DAACS, described the extensive training users must undergo in order to deposit their data in the archive to ensure the standards outlined by the Cataloging Manual are kept. This regimented process ensures the quality and consistency of the data- and thus its utility. The result is a publicly available dataset of the history of Monticello for researchers of all kinds to examine this important site in America’s history.
These sherds have been washed and numbered to denote their context.
Our trip to Monticello Archaeology was eye-opening, as none of us had any practical experience with archaeological research or data. The impressive DAACS protocols and standards represent an important aspect of all scientific research - the ability to accurately capture large amounts of data in a systematic, thoughtful way - and then share it freely with others.
Jul 10, 2014
by
Tom Stafford
Jason Mitchell's essay 'On the emptiness of failed replications' is notable for being against the current effort to publish replication attempts. Commentary on the essay that I saw was pretty negative (e.g. "awe-inspiringly clueless", “defensive pseudo-scientific, anti-Bayesian academic ass-covering”, "Do you get points in social psychology for publicly declaring you have no idea how science works?").
Although I reject his premises, and disagree with his conclusion, I don't think Mitchell's arguments are incomprehensibly mad. This seems to put me in a minority, so I thought I'd try and explain the value in what he's saying. I'd like to walk through his essay assuming he is a thoughtful rational person. Why would a smart guy come to the views he has? What is he really trying to say, and what are his assumptions about the world of psychology that might, perhaps, illuminate our own assumptions?
Experiments as artefacts, not samples
First off, key to Mitchell's argument is a view that experiments are complex artefacts, in the construction of which errors are very likely. Effects, in this view, are hard won, eventually teased out via a difficult process of refinement and validation. The value of replication is self-evident to anyone who thinks statistically: sampling error and publication bias will produce lots of false positives, you improve your estimate of the true effect by independent samples (= replications). Mitchell seems to be saying that the experiments are so complex that replications by other labs aren't independent samples of the same effect. Although they are called replications there are, he claims, most likely to be botched, and so informative of nothing more than the incompetence of the replicators.
When teaching our students many of us will have deployed the saying "The plural of anecdote is not data". What we mean by this is that many weak observations - of ghosts, aliens or psychic powers - do not combine multiplicatively to make strong evidence in favour of these phenomena. If I've read him right, Mitchell is saying the same thing about replication experiments - many weak experiments are uninformative about real effects.
Tacit practical knowledge
Part of Mitchell's argument rests on the importance of tacit knowledge in running experiments (see his section "The problem with recipe-following"). We all know that tacit knowledge about experimental procedures exists in science. Mitchell puts a heavy weight on the importance of this. This is a position which presumably would have lots of sympathy from Daniel Kahneman, who suggested that all replication attempts should involve the original authors.
There's a tension here between how science should be and how it is. Obviously our job is to make things explicit, to explain how to successfully run experiments so that anyone can run them but the truth is, full explanations aren't always possible. Sure, anyone can try and replicate based on a methods section, but - says Mitchell - you will probably be wasting your time generating noise rather than data, and shouldn't be allowed to commit this to the scientific record.
Most of us would be comfortable with the idea that if a non-psychologist ran our experiments they might make some serious errors (one thinks of the hash some physical scientists made of psi-experiments, failing completely to account for things like demand effects, for example). Mitchell's line of thought here seems to take this one step further, you can't run a social psychologist's experiments without special training in social psychology. Or even, maybe, you can't successfully run another lab's experiment without training from that lab.
I think happen to think he's wrong on this, and that he neglects to mention the harm of assuming that successful experiments have a "special sauce" which cannot be easily communicated (it seems to be a road to elitism and mysticism to me, completely contrary to the goals science should have). Nonetheless, there's definitely some truth to the idea, and I think it is useful to consider the errors we will make if we assume the contrary, that methods sections are complete records and no special background is required to run experiments.
Innuendo
Mitchell makes the claim that targeting an effect for replication amounts to the innuendo that the effects under inspection are unreliable, which is a slur on the scientists who originally published them. Isn't this correct? Several people on twitter admitted, or tacitly admitted, that their prior beliefs were that many of these effects aren't real. There is something disingenuous about claiming, on the one hand, that all effects should be replicated, but, on the other, targeting particular effects for attention. If you bought Mitchell's view that experiments are delicate artefacts which render most replications uninformative, you can see how the result is a situation which isn't just uninformative but actively harmful to the hard-working psychologists whose work is impugned. Even if you don't buy that view, you might think that selection of which effects should be the focus of something like the Many Labs project is an active decision made by a small number of people, and which targets particular individuals. How this processes works out in practice deserves careful consideration, even if everyone agrees that it is a Good Thing overall.
Caveats
There are a number of issues in Mitchell's essay I haven't touched on - this isn't meant to be a complete treatment, just an explanation of some of the reasonable arguments I think he makes. Even if I disagree with them, I think they are reasonable; they aren't as obviously wrong as some have suggested and should be countered rather than dismissed.
Stepping back, my take on the 'replication crisis' in psychology is that it really isn't about replication. Instead, this is what digital disruption looks like in a culture organised around scholarly kudos rather than profit. We now have the software tools to coordinate data collection, share methods and data, analyse data, and interact with non-psychologists, both directly and via the media, in unprecedented ways and at an unprecedented rate. Established scholarly communities are threatened as "the way things are done" is challenged. Witness John Bargh's incredulous reaction to having his work challenged (and note that this was 'a replicate and explain via alternate mechanism' type study that Mitchell says is a valid way of doing replication). Witness the recent complaint of medical researcher Jonathan S. Nguyen-Van-Tam when a journalist included critique of his analysis technique in a report on his work. These guys obviously believe in a set of rules concerning academic publishing which many of us aren't fully aware of or believe no longer apply.
By looking at other disrupted industries, such as music or publishing, we can discern morals for both sides. Those who can see the value in the old way of doing things, like Mitchell, need to articulate that value and fast. There's no way of going back, but we need to salvage the good things about tight-knit, slow moving, scholarly communities. The moral for the progressives is that we shouldn't let the romance of change blind us to the way that the same old evils will reassert themselves in new forms, by hiding behind a facade of being new, improved and more equitable.
Jul 9, 2014
by
Sean Mackinnon
Jason Mitchell recently wrote an article entitled “On the Emptiness of Failed Replications.”. In this article, Dr. Mitchell takes an unconventional and extremely strong stance against replication, arguing that: “… studies that produce null results -- including preregistered studies -- should not be published.” The crux of the argument seems to be that "scientists who get p > .05 are just incompetent." It completely ignores the possibility that a positive result could also (maybe even equally) be due to experimenter error. Dr. Mitchell also appears to ignore the possibility of simply getting a false positive (which is expected to happen under the null in 5% of cases).
More importantly, it ignores issues of effect size and treats the outcome of research as a dichotomous "success or fail.” The advantages of examining effect sizes over simple directional hypotheses using null hypothesis significance testing are beyond the scope of this short post, but you might check out Sullivan and Feinn (2012) as an open-access starting point. Generally speaking, the problem is that sampling variation means that some experiments will find null results even when the experimenter does everything right. As an illustration, below is 1000 simulated correlations, assuming that r = .30 in the population, and a sample size of 100 (I used a monte carlo method).
In this picture, the units of analysis are individual correlations obtained in 1 of 1000 hypothetical research studies. The x-axis is the value of the correlation coefficient found, and the y-axis is the number of studies reporting that value. The red line is the critical value for significant results at p < .05 assuming a sample size of 100. As you can see from this picture, the majority of studies are supportive of an effect that is greater than zero. However (simply due to chance) all the studies to the left of the red line turned out non-significant. If we suppressed all the null results (i.e., all those unlucky scientists to the left of the red line) as Dr. Mitchell suggests, then our estimate of the effect size in the population would be inaccurate; specifically, it would appear to be larger than it really is, because certain aspects of random variation (i.e., null results) are being selectively suppressed. Without the minority of null findings (in addition to the majority of positive findings) the overall estimate of the effect cannot be correctly estimated.
The situation is even more grim if there really is no effect in the population.
In this case, a small proportion of studies will produce false positives, with a roughly equal chance of an effect in either direction. If we fail to report null results, false positives may be reified as substantive effects. The reversal of signs across repeated studies might be a warning sign that the effect doesn’t really exist, but without replication, a single false positive could define a field if it happens (by chance) to be in line with prior theory.
With this in mind, I also disagree that replications are “publicly impugning the scientific integrity of their colleagues.” Some people feel threatened or attacked by replication. The ideas we produce as scientists are close to our hearts, and we tend to get defensive when they’re challenged. If we focus on effect sizes, rather than the “success or fail” logic of null hypothesis significance testing, then I don’t believe that “failed” replications damage the integrity of the original author, but rather simply suggests that we should modulate the estimate of the effect size downwards. In this framework, replication is less about “proving someone wrong” and more about centering on the magnitude of an effect size.
Something that is often missed in discussion of replication is that the very nature of randomness inherent in the statistical procedures scientists use means that any individual study (even if perfectly conducted) will probably generate an effect size that is a bit larger or smaller than it is in the population. It is only through repeated experiments that we are able to center on an accurate estimate of the effect size. This issue is independent of researcher competence, and means that even the most competent researchers will come to the wrong conclusions occasionally because of the statistical procedures and cutoffs we’ve chosen to rely on. With this in mind, people should be aware that a failed replication does not necessarily mean that one of the two researchers is incorrect or incompetent – instead, it is assumed (until further evidence is collected) that the best estimate is a weighted average of the effect size from each research study.
For some more commentary from other bloggers, you might check out the following links:
"Are replication efforts pointless?" by Richard Tomsett
"Being as wrong as can be on the so-called replication crisis of science" by drugmonkey at Scientopia
"Are replication efforts useless?" by Jan Moren
"Jason Mitchell’s essay" by Chris Said
"#MethodsWeDontReport – brief thought on Jason Mitchell versus the replicators" by Micah Allen
"On 'On the emptiness of failed replications'" by Neuroskeptic
Jul 2, 2014
by
Ryne Sherman
This article was originally posted in the author's personal blog.
Imagine you have a two group between-S study with N=30 in each group. You compute a two-sample t-test and the result is p = .09, not statistically significant with an effect size r = .17. Unbeknownst to you there is really no relationship between the IV and the DV. But, because you believe there is a relationship (you decided to run the study after all!), you think maybe adding five more subjects to each condition will help clarify things. So now you have N=35 in each group and you compute your t-test again. Now p = .04 with r = .21.
If you are reading this blog you might recognize what happened here as an instance of p-hacking. This particular form (testing periodically as you increase N) of p-hacking was one of the many data analytic flexibility issues exposed by Simmons, Nelson, and Simonshon (2011). But what are the real consequences of p-hacking? How often will p-hacking turn a null result into a positive result? What is the impact of p-hacking on effect size?
These were the kinds of questions that I had. So I wrote a little R function that simulates this type of p-hacking. The function – called phack – is designed to be flexible, although right now it only works for two-group between-S designs. The user is allowed to input and manipulate the following factors (argument name in parentheses):
- Initial Sample Size (initialN): The initial sample size (for each group) one had in mind when beginning the study (default = 30).
- Hack Rate (hackrate): The number of subjects to add to each group if the p-value is not statistically significant before testing again (default = 5).
- Population Means (grp1M, grp2M): The population means (Mu) for each group (default 0 for both).
- Population SDs (grp1SD, grp2SD): The population standard deviations (Sigmas) for each group (default = 1 for both).
- Maximum Sample Size (maxN): You weren’t really going to run the study forever right? This is the sample size (for each group) at which you will give up the endeavor and go run another study (default = 200).
- Type I Error Rate (alpha): The value (or lower) at which you will declare a result statistically significant (default = .05).
- Hypothesis Direction (alternative): Did your study have a directional hypothesis? Two-group studies often do (i.e., this group will have a higher mean than that group). You can choose from “greater” (Group 1 mean is higher), “less” (Group 2 mean is higher), or “two.sided” (any difference at all will work for me, thank you very much!). The default is “greater.”
- Display p-curve graph (graph)?: The function will output a figure displaying the p-curve for the results based on the initial study and the results for just those studies that (eventually) reached statistical significance (default = TRUE). More on this below.
- How many simulations do you want (sims). The number of times you want to simulate your p-hacking experiment.
To make this concrete, consider the following R code:
res <- phack(initialN=30, hackrate=5, grp1M=0, grp2M=0, grp1SD=1,
grp2SD=1, maxN=200, alpha=.05, alternative="greater", graph=TRUE, sims=1000)
This says you have planned a two-group study with N=30 (initialN=30) in each group. You are going to compute your t-test on that initial sample. If that is not statistically significant you are going to add 5 more (hackrate=5) to each group and repeat that process until it is statistically significant or you reach 200 subjects in each group (maxN=200). You have set the population Ms to both be 0 (grp1M=0; grp2M=0) with SDs of 1 (grp1SD=1; grp2SD=1). You have set your nominal alpha level to .05 (alpha=.05), specified a direction hypothesis where group 1 should be higher than group 2 (alternative=“greater”), and asked for graphical output (graph=TRUE). Finally, you have requested to run this simulation 1000 times (sims=1000).
So what happens if we run this experiment?1 So we can get the same thing, I have set the random seed in the code below.
source("http://rynesherman.com/phack.r") # read in the p-hack function
set.seed(3)
res <- phack(initialN=30, hackrate=5, grp1M=0, grp2M=0, grp1SD=1, grp2SD=1,
maxN=200, alpha=.05, alternative="greater", graph=TRUE, sims=1000)
The following output appears in R:
Proportion of Original Samples Statistically Significant = 0.054
Proportion of Samples Statistically Significant After Hacking = 0.196
Probability of Stopping Before Reaching Significance = 0.805
Average Number of Hacks Before Significant/Stopping = 28.871
Average N Added Before Significant/Stopping = 144.355
Average Total N 174.355
Estimated r without hacking 0
Estimated r with hacking 0.03
Estimated r with hacking 0.19 (non-significant results not included)
The first line tells us how many (out of the 1000 simulations) of the originally planned (N=30 in each group) studies had a p-value that was .05 or less. Because there was no true effect (grp1M = grp2M) this at just about the nominal rate of .05. But what if we had used our p-hacking scheme (testing every 5 subjects per condition until significant or N=200)? That result is in the next line. It shows that just about 20% of the time we would have gotten a statistically significant result. So this type of hacking has inflated our Type I error rate from 5% to 20%. How often would we have given up (i.e., N=200) before reaching statistical significance? That is about 80% of the time. We also averaged 28.87 “hacks” before reaching significance/stopping, averaged having to add N=144 (per condition) before significance/stopping, and had an average total N of 174 (per condition) before significance/stopping.
What about effect sizes? Naturally the estimated effect size (r) was .00 if we just used our original N=30 in each group design. If we include the results of all 1000 completed simulations that effect size averages out to be r = .03. Most importantly, if we exclude those studies that never reached statistical significance, our average effect size r = .19.
This is pretty telling. But there is more. We also get this nice picture:
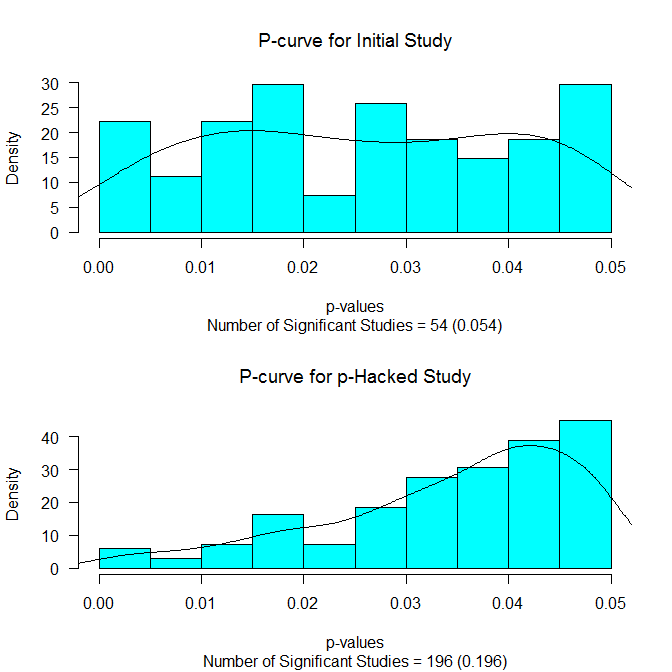
It shows the distribution of the p-values below .05 for the initial study (upper panel) and for those p-values below .05 for those reaching statistical significance. The p-curves (see Simonsohn, Nelson, & Simmons, 2013) are also drawn on. If there is really no effect, we should see a flat p-curve (as we do in the upper panel). And if there is no effect and p-hacking has occurred, we should see a p-curve that slopes up towards the critical value (as we do in the lower panel).
Finally, the function provides us with more detailed output that is summarized above. We can get a glimpse of this by running the following code:
This generates the following output:
Initial.p Hackcount Final.p NAdded Initial.r Final.r
0.86410908 34 0.45176972 170 -0.14422580 0.006078565
0.28870264 34 0.56397332 170 0.07339944 -0.008077691
0.69915219 27 0.04164525 135 -0.06878039 0.095492249
0.84974744 34 0.30702946 170 -0.13594941 0.025289555
0.28048754 34 0.87849707 170 0.07656582 -0.058508736
0.07712726 34 0.58909693 170 0.18669338 -0.011296131
The object res contains the key results from each simulation including the p-value for the initial study (Initial.p), the number of times we had to hack (Hackcount), the p-value for the last study run (Final.p), the total N added to each condition (NAdded), the effect size r for the initial study (Initial.r), and the effect size r for the last study run (Final.r).
So what can we do with this? I see lots of possibilities and quite frankly I don’t have the time or energy to do them. Here are some quick ideas:
- What would happen if there were a true effect?
- What would happen if there were a true (but small) effect?
- What would happen if we checked for significance after each subject (hackrate=1)?
- What would happen if the maxN were lower?
- What would happen if the initial sample size was larger/smaller?
- What happens if we set the alpha = .10?
- What happens if we try various combinations of these things?
I’ll admit I have tried out a few of these ideas myself, but I haven’t really done anything systematic. I just thought other people might find this function interesting and fun to play with.
1 By the way, all of these arguments are set to their default, so you can do the same thing by simply running:
Jun 25, 2014
by
EJ Wagenmakers
James Randi, magician and scientific skeptic, has compared those who believe in the paranormal to “unsinkable rubber ducks”: after a particular claim has been thoroughly debunked, the ducks submerge, only to resurface again a little later to put forward similar claims.
In light of this analogy, it comes as no surprise that Bem and colleagues have produced a new paper claiming that people can look into the future. The paper is titled “Feeling the Future: A Meta-Analysis of 90 Experiments on the Anomalous Anticipation of Random Future Events” and it is authored by Bem, Tressoldi, Rabeyron, and Duggan.
Several of my colleagues have browsed Bem's meta-analysis and have asked for my opinion. Surely, they say, the statistical evidence is overwhelming, regardless of whether you compute a p-value or a Bayes factor. Have you not changed your opinion? This is a legitimate question, one which I will try and answer below by showing you my review of an earlier version of the Bem et al. manuscript.
I agree with the proponents of precognition on one crucial point: their work is important and should not be ignored. In my opinion, the work on precognition shows in dramatic fashion that our current methods for quantifying empirical knowledge are insufficiently strict. If Bem and colleagues can use a meta-analysis to demonstrate the presence of precognition, what should we conclude from a meta-analysis on other, more plausible phenomena?
Disclaimer: the authors have revised their manuscript since I reviewed it, and they are likely to revise their manuscript again in the future. However, my main worries call into question the validity of the enterprise as a whole.
To keep this blog post self-contained, I have added annotations in italics to provide context for those who have not read the Bem et al. manuscript in detail.
My review of Bem, Tressoldi, Rabeyron, and Duggan
Read more...