Nov 20, 2013
by
Denny Borsboom
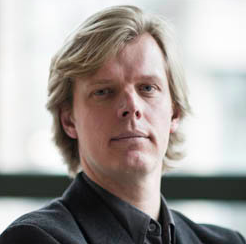
In the past few months, the Center for Open Science and its associated enterprises have gathered enormous support in the community of psychological scientists. While these developments are happy ones, in my view, they also cast a shadow over the field of psychology: clearly, many people think that the activities of the Center for Open Science, like organizing massive replication work and promoting preregistration, are necessary. That, in turn, implies that something in the current scientific order is seriously broken. I think that, apart from working towards improvements, it is useful to investigate what that something is. In this post, I want to point towards a factor that I think has received too little attention in the public debate; namely, the near absence of unambiguously formalized scientific theory in psychology.
Scientific theories are perhaps the most bizarre entities that the scientific imagination has produced. They have incredible properties that, if we weren’t so familiar with them, would do pretty well in a Harry Potter novel. For instance, scientific theories allow you to work out, on a piece of paper, what would happen to stuff in conditions that aren’t actually realized. So you can figure out whether an imaginary bridge will stand or collapse in imaginary conditions. You can do this by simply just feeding some imaginary quantities that your imaginary bridge would have (like its mass and dimensions) to a scientific theory (say, Newton’s) and out comes a prediction on what will happen. In the more impressive cases, the predictions are so good that you can actually design the entire bridge on paper, then build it according to specifications (by systematically mapping empirical objects to theoretical terms), and then the bridge will do precisely what the theory says it should do. No surprises.
That’s how they put a man on the moon and that’s how they make the computer screen you’re now looking at. It’s all done in theory before it’s done for real, and that’s what makes it possible to construct complicated but functional pieces of equipment. This is, in effect, why scientific theory makes technology possible, and therefore this is an absolutely central ingredient of the scientific enterprise which, without technology, would be much less impressive than it is.
It’s useful to take stock here, and marvel. A good scientific theory allows you infer what would happen to things in certain situations without creating the situations. Thus, scientific theories are crystal balls that actually work. For this reason, some philosophers of science have suggested that scientific theories should be interpreted as inference tickets. Once you’ve got the ticket, you get to sidestep all the tedious empirical work. Which is great, because empirical work is, well, tedious. Scientific theories are thus exquisitely suited to the needs of lazy people.
My field – psychology – unfortunately does not afford much of a lazy life. We don’t have theories that can offer predictions sufficiently precise to intervene in the world with appreciable certainty. That’s why there exists no such thing as a psychological engineer. And that’s why there are fields of theoretical physics, theoretical biology, and even theoretical economics, while there is no parallel field of theoretical psychology. It is a sad but, in my view, inescapable conclusion: we don’t have much in the way of scientific theory in psychology. For this reason, we have very few inference tickets – let alone inference tickets that work.
And that’s why psychology is so hyper-ultra-mega empirical. We never know how our interventions will pan out, because we have no theory that says how they will pan out (incidentally, that’s also why we need preregistration: in psychology, predictions are made by individual researchers rather than by standing theory, and you can’t trust people the way you can trust theory). The upshot is that, if we want to know what would happen if we did X, we have to actually do X. Because we don’t have inference tickets, we never get to take the shortcut. We always have to wade through the empirical morass. Always.
This has important consequences. For instance, as a field has less theory, it has to leave more to the data. Since you can’t learn anything from data without the armature of statistical analysis, a field without theory tends to grow a thriving statistical community. Thus, the role of statistics grows as soon as the presence of scientific theory wanes. In extreme cases, when statistics has entirely taken over, fields of inquiry can actually develop a kind of philosophical disorder: theoretical amnesia. In fields with this disorder, researchers no longer know what a theory is, which means that they can neither recognize its presence nor its absence. In such fields, for instance, a statistical model – like a factor model – can come to occupy the vacuum created by the absence of theory. I am often afraid that this is precisely what has happened with the advent of “theories” like those of general intelligence (a single factor model) and the so-called “Big Five” of personality (a five-factor model). In fact, I am afraid that this happened in many fields in psychology, where statistical models (which, in their barest null-hypothesis testing form, are misleadingly called “effects”) rule the day.
If your science thrives on experiment and statistics, but lacks the power of theory, you get peculiar problems. Most importantly, you get slow. To see why, it’s interesting to wonder how psychologists would build a bridge, if they were to use their typical methodological strategies. Probably, they would build a thousand bridges, record whether they stand or fall, and then fit a regression equation to figure out which properties are predictive of the outcome. Predictors would be chosen on the basis of statistical significance, which would introduce a multiple testing problem. In response, some of the regressors might be clustered through factor analysis, to handle the overload of predictive variables. Such analyses would probably indicate lots of structure in the data, and psychologists would likely find that the bridges’ weight, size, and elasticity loads on a single latent “strength factor”, producing the “theory” that bridges higher on the “strength factor” are less likely to fall down. Cross validation of the model would be attempted by reproducing the analysis in a new sample of a thousand bridges, to weed out chance findings. It’s likely that, after many years of empirical research, and under a great number of “context-dependent” conditions that would be poorly understood, psychologists would be able to predict a modest but significant proportion of the variance in the outcome variable. Without a doubt, it would ta ke a thousand years to establish empirically what Newton grasped in a split second, as he wrote down his F=m*a.
Because increased reliance on empirical data makes you so incredibly slow, it also makes you susceptible to fads and frauds. A good theory can be tested in an infinity of ways, many of which are directly available to the interested reader (this is what give classroom demonstrations such enormous evidential force). But if your science is entirely built on generalizations derived from specifics of tediously gathered experimental data, you can’t really test these generalizations without tediously gathering the same, or highly similar, experimental data. That’s not something that people typically like to do, and it’s certainly not what journals want to print. As a result, a field can become dominated by poorly tested generalizations. When that happens, you’re in very big trouble. The reason is that your scientific field becomes susceptible to the equivalent of what evolutionary theorists call free riders: people who capitalize on the invested honest work of others by consistently taking the moral shortcut. Free riders can come to rule a scientific field if two conditions are satisfied: (a) fame is bestowed on whoever dares to make the most adventurous claims (rather than the most defensible ones), and (b) it takes longer to falsify a bogus claim than it takes to become famous. If these conditions are satisfied, you can build your scientific career on a fad and get away with it. By the time they find out your work really doesn’t survive detailed scrutiny, you’re sitting warmly by the fire in the library of your National Academy of Sciences1.
Much of our standard methodological teachings in psychology rest on the implicit assumption that scientific fields are similar if not identical in their methodological setup. That simply isn’t true. Without theory, the scientific ball game has to be played by different rules. I think that these new rules are now being invented: without good theory, you need fast acting replication teams, you need a reproducibility project, and you need preregistered hypotheses. Thus, the current period of crisis may lead to extremely important methodological innovations – especially those that are crucial in fields that are low on theory.
Nevertheless, it would be extremely healthy if psychologists received more education in fields which do have some theories, even if they are empirically shaky ones, like you often see in economics or biology. In itself, it’s no shame that we have so little theory: psychology probably has the hardest subject matter ever studied, and to change that may very well take a scientific event of the order of Newton’s discoveries. I don’t know how to do it and I don’t think anybody else knows either. But what we can do is keep in contact with other fields, and at least try to remember what theory is and what it’s good for, so that we don’t fall into theoretical amnesia. As they say, it’s the unknown unknowns that hurt you most.
1 Caveat: I am not saying that people do this on purpose. I believe that free riders are typically unaware of the fact that they are free riders – people are very good at labeling their own actions positively, especially if the rest of the world says that they are brilliant. So, if you think this post isn’t about you, that could be entirely wrong. In fact, I cannot even be sure that this post isn’t about me.
Nov 13, 2013
by
Etienne LeBel
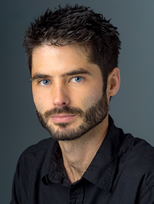
In 1959, Festinger and Carlsmith reported the results of an experiment that spawned a voluminous body of research on cognitive dissonance. In that experiment, all subjects performed a boring task. Some participants were paid $1 or $20 to tell the next subject the task was interesting and fun whereas participants in a control condition did no such thing. All participants then indicated how enjoyable they felt the task was, their desire to participate in another similar experiment, and the scientific importance of the experiment. The authors hypothesized that participants paid $1 to tell the next participant that the boring task they just completed was interesting would experience internal dissonance, which could be reduced by altering their attitudes on the three outcomes measures. A little known fact about the results of this experiment, however, is that only on one of these outcome measures did a statistically significant effect emerge across conditions. The authors reported that subjects paid $1 enjoyed the task more than those paid $20 (or the control participants), but no statistically significant differences emerged on the other two measures.
In another highly influential paper, Word, Zanna, and Cooper (1974) documented the self-fulfilling prophecies of racial stereotypes. The researchers had white subjects interview trained white and black applicants and coded for six non-verbal behaviors of immediacy (distance, forward lean, eye contact, shoulder orientation, interview length, and speech error rate). They found that that white interviewers treated black applicants with lower levels of non-verbal immediacy than white applicants. In a follow-up study involving white subjects only, applicants treated with less immediate non-verbal behaviors were judged to perform less well during the interview than applicants treated with more immediate non-verbal behaviors. A fascinating result, however, a little known fact about this paper is that only three of the six measures of non-verbal behaviors assessed in the first study (and subsequently used in the second study) were statistically significant.
What do these two examples make salient in relation to how psychologists report their results nowadays? Regular readers of prominent journals like Psychological Science and Journal of Personality and Social Psychology may see what I’m getting at: It is very rare these days to see articles in these journals wherein half or most of the reported dependent variables (DVs) fail to show statistically significant effects. Rather, one typically sees squeaky-clean looking articles where all of the DVs show statistically significant effects across all of the studies, with an occasional mention of a DV achieving “marginal significance” (Giner-Sorolla, 2012).
In this post, I want us to consider the possibility that psychologists’ reporting practices may have changed in the past 50 years. This then raises the question as to how this came about. One possibility is that as incentives became increasingly more perverse in psychology (Nosek,Spies, & Motyl, 2012), some researchers realized that they could out-compete their peers by reporting “cleaner” looking results which would appear more compelling to editors and reviewers (Giner-Sorolla, 2012). For example, decisions were made to simply not report DVs that failed to show significant differences across conditions or that only achieved “marginal significance”. Indeed, nowadays sometimes even editors or reviewers will demand that such DVs not be reported (see PsychDisclosure.org; LeBel et al., 2013). A similar logic may also have contributed to researchers’ deciding not to fully report independent variables that failed to yield statistically significant effects and not fully reporting the exclusion of outlying participants due to fear that this information may raise doubts among the editor/reviewers and hurt their chance of getting their foot in the door (i.e., at least getting a revise-and-resubmit).
An alternative explanation is that new tools and technology have given us the ability to measure a greater number of DVs, which makes it more difficult to report on all them. For example, neuroscience (e.g., EEG, fMRI) and eye-tracking methods yield multitudes of analyzable data that were not previously available. Though this is undeniably true, the internet and online article supplements gives us the ability to fully report our methods and results and use the article to draw attention to the most interesting data.
Considering the possibility that psychologists’ reporting practices have changed in the past 50 years has implications for how to construe recent calls for the need to raise reporting standards in psychology (LeBel et al., 2013; Simmons, Nelson, & Simonsohn, 2011; Simmons, Nelson, & Simonsohn, 2012). Rather than seeing these calls as rigid new requirements that might interfere with exploratory research and stifle our science, one could construe such calls as a plea to revert back to the fuller reporting of results that used to be the norm in our science. From this perspective, it should not be viewed as overly onerous or authoritarian to ask researchers to disclose all excluded observations, all tested experimental conditions, all analyzed measures, and their data collection termination rule (what I’m now calling the BASIC 4 methodological categories covered by PsychDisclosure.org and Simmons et al.’s, 2012 21-word solution). It would simply be the way our forefathers used to do it.
References
Festinger, L. & Carlsmith, J. M. (1959). Cognitive consequences of forced compliance. The Journal of Abnormal and Social Psychology, 58(2), Mar 1959, 203-210. doi: 10.1037/h0041593
Giner-Sorolla, R. (2012). Science or art? How esthetic standards grease the way through the publication bottleneck but undermine science. Perspectives on Psychological Science, 7(6), 562–571. 10.1177/1745691612457576
LeBel, E. P., Borsboom, D., Giner-Sorolla, R., Hasselman, F., Peters, K. R., Ratliff, K. A., & Smith, C. T. (2013). PsychDisclosure.org: Grassroots support for reforming reporting standards in psychology. Perspectives on Psychological Science, 8(4), 424-432. doi: 10.1177/1745691613491437
Nosek, B. A., Spies, J. R., & Motyl, M. (2012). Scientific utopia: II. Restructuring incentives and practices to promote truth over publishability. Perspectives on Psychological Science, 7, 615-631. doi: 10.1177/1745691612459058
Simmons J., Nelson L. & Simonsohn U. (2011) False-Positive Psychology: Undisclosed Flexibility in Data Collection and Analysis Allow Presenting Anything as Significant. Psychological Science, 22(11), 1359-1366.
Simmons J., Nelson L. & Simonsohn U. (2012) A 21 Word Solution Dialogue: The Official Newsletter of the Society for Personality and Social Psychology, 26(2), 4-7.
Word, C. O., Zanna, M. P., & Cooper, J. (1974). The nonverbal mediation of self-fulfilling prophecies in interracial interaction. Journal of Experimental Social Psychology, 10(2), 109–120. doi: 10.1016/0022-1031(74)90059-6
Nov 3, 2013
by
Sean Mackinnon
What is statistical power and precision?
This post is going to give you some practical tips to increase statistical power in your research. Before going there though, let’s make sure everyone is on the same page by starting with some definitions.
Statistical power is the probability that the test will reject the null hypothesis when the null hypothesis
is false. Many authors suggest a statistical power rate of at least .80, which corresponds to an 80% probability of not committing a Type II error.
Precision refers to the width of the confidence interval for an effect size. The smaller this width, the more precise your results are. For 80% power, the confidence interval width will be roughly plus or minus 70% of the population effect size (Goodman & Berlin, 1994). Studies that have low precision have a greater probability of both Type I and Type II errors (Button et al., 2013).
To get an idea of how this works, here are a few examples of the sample size required to achieve .80 power for small, medium, and large (Cohen, 1992) correlations as well as the expected confidence intervals
Population Effect Size |
Sample Size for 80% Power |
Estimated Precision |
r = .10 |
782 |
95% CI [.03, .17] |
r = .30 |
84 |
95% CI [.09, .51] |
r = .50 |
29 |
95% CI [.15, .85] |
Studies in psychology are grossly underpowered
Okay, so now you know what power is. But why should you care? Fifty years ago, Cohen (1962) estimated the statistical power to detect a medium effect size in abnormal psychology was about .48. That’s a false negative rate of 52%, which is no better than a coin-flip! The situation has improved slightly, but it’s still a serious problem today. For instance, one review suggested only 52% of articles in the applied psychology literature achieved .80 power for a medium effect size (Mone et al., 1996). This is in part because psychologists are studying small effects. One massive review of 322 meta-analyses including 8 million participants (Richard et al., 2003) suggested that the average effect size in social psychology is relatively small (r = .21). To put this into perspective, you’d need 175 participants to have .80 power for a simple correlation between two variables at this effect size. This gets even worse when we’re studying interaction effects. One review suggests that the average effect size for interaction effects is even smaller (f2 = .009), which means that sample sizes of around 875 people would be needed to achieve .80 power (Aguinis et al., 2005). Odds are, if you took the time to design a research study and collect data, you want to find a relationship if one really exists. You don’t want to "miss" something that is really there. More than this, you probably want to have a reasonably precise estimate of the effect size (it’s not that impressive to just say a relationship is positive and probably non-zero). Below, I discuss concrete strategies for improving power and precision.
What can we do to increase power?
It is well-known that increasing sample size increases statistical power and precision. Increasing the population effect size increases statistical power, but has no effect on precision (Maxwell et al., 2008). Increasing sample size improves power and precision by reducing standard error of the effect size. Take a look at this formula for the confidence interval of a linear regression coefficient (McClelland, 2000):
MSE is the mean square error, n is the sample size, Vx is the variance of X, and (1-R2) is the proportion of the variance in X not shared by any other variables in the model. Okay, hopefully you didn’t nod off there. There’s a reason I’m showing you this formula. In this formula, decreasing any value in the numerator (MSE) or increasing anything in the denominator (n, Vx, 1-R2) will decrease the standard error of the effect size, and will thus increase power and precision. This formula demonstrates that there are at least three other ways to increase statistical power aside from sample size: (a) Decreasing the mean square error; (b) increasing the variance of x; and (c) increasing the proportion of the variance in X not shared by any other predictors in the model. Below, I’ll give you a few ways to do just that.
Recommendation 1: Decrease the mean square error
Referring to the formula above, you can see that decreasing the mean square error will have about the same impact as increasing sample size. Okay. You’ve probably heard the term "mean square error" before, but the definition might be kind of fuzzy. Basically, your model makes a prediction for what the outcome variable (Y) should be, given certain values of the predictor (X). Naturally, it’s not a perfect prediction because you have measurement error, and because there are other important variables you probably didn’t measure. The mean square error is the difference between what your model predicts, and what the true values of the data actually are. So, anything that improves the quality of your measurement or accounts for potential confounding variables will reduce the mean square error, and thus improve statistical power. Let’s make this concrete. Here are three specific techniques you can use:
a) Reduce measurement error by using more reliable measures(i.e., better internal consistency, test-retest reliability, inter-rater reliability, etc.). You’ve probably read that .70 is the "rule-of-thumb" for acceptable reliability. Okay, sure. That’s publishable. But consider this: Let’s say you want to test a correlation coefficient. Assuming both measures have a reliability of .70, your observed correlation will be about 1.43 times smaller than the true population parameter (I got this using Spearman’s correlation attenuation formula). Because you have a smaller observed effect size, you end up with less statistical power. Why do this to yourself? Reduce measurement error. If you’re an experimentalist, make sure you execute your experimental manipulations exactly the same way each time, preferably by automating them. Slight variations in the manipulation (e.g., different locations, slight variations in timing) might reduce the reliability of the manipulation, and thus reduce power.
b) Control for confounding variables. With correlational research, this means including control variables that predict the outcome variable, but are relatively uncorrelated with other predictor variables. In experimental designs, this means taking great care to control for as many possible confounds as possible. In both cases, this reduces the mean square error and improves the overall predictive power of the model – and thus, improves statistical power. Be careful when adding control variables into your models though: There are diminishing returns for adding covariates. Adding a couple of good covariates is bound to improve your model, but you always have to balance predictive power against model complexity. Adding a large number of predictors can sometimes lead to overfitting (i.e., the model is just describing noise or random error) when there are too many predictors in the model relative to the sample size. So, controlling for a couple of good covariates is generally a good idea, but too many covariates will probably make your model worse, not better, especially if the sample is small.
c) Use repeated-measures designs. Repeated measures designs are where participants are measured multiple times (e.g., once a day surveys, multiple trials in an experiment, etc.). Repeated measures designs reduce the mean square error by partitioning out the variance due to individual participants. Depending on the kind of analysis you do, it can also increase the degrees of freedom for the analysis substantially. For example, you might only have 100 participants, but if you measured them once a day for 21 days, you’ll actually have 2100 data points to analyze. The data analysis can get tricky and the interpretation of the data may change, but many multilevel and structural equation models can take advantage of these designs by examining each measurement occasion (i.e., each day, each trial, etc.) as the unit of interest, instead of each individual participant. Increasing the degrees of freedom is much like increasing the sample size in terms of increasing statistical power. I’m a big fan of repeated measures designs, because they allow researchers to collect a lot of data from fewer participants.
Recommendation 2: Increase the variance of your predictor variable
Another less-known way to increase statistical power and precision is to increase the variance of your predictor variables (X). The formula listed above shows that doubling the variance of X is has the same impact on increasing statistical precision as doubling the sample size does! So it’s worth figuring this out.
a) In correlational research, use more comprehensive continuous measures. That is, there should be a large possible range of values endorsed by participants. However, the measure should also capture many different aspects of the construct of interest; artificially increasing the range of X by adding redundant items (i.e., simply re-phrasing existing items to ask the same question) will actually hurt the validity of the analysis. Also, avoid dichotomizing your measures (e.g., median splits), because this reduces the variance and typically reduces power (MacCallum et al., 2002).
b) In experimental research, unequally allocating participants to each condition can improve statistical power. For example, if you were designing an experiment with 3 conditions (let’s say low, medium, or high self-esteem). Most of us would equally assign participants to all three groups, right? Well, as it turns out, assigning participants equally across groups usually reduces statistical power. The idea behind assigning participants unequally to conditions is to maximize the variance of X for the particular kind of relationship under study -- which, according the formula I gave earlier, will increase power and precision. For example, the optimal design for a linear relationship would be 50% low, 50% high, and omit the medium condition. The optimal design for a quadratic relationship would be 25% low, 50% medium, and 25% high. The proportions vary widely depending on the design and the kind of relationship you expect, but I recommend you check out McClelland (1997) to get more information on efficient experimental designs. You might be surprised.
Recommendation 3: Make sure predictor variables are uncorrelated with each other
A final way to increase statistical power is to increase the proportion of the variance in X not shared with other variables in the model. When predictor variables are correlated with each other, this is known as colinearity. For example, depression and anxiety are positively correlated with each other; including both as simultaneous predictors (say, in multiple regression) means that statistical power will be reduced, especially if one of the two variables actually doesn’t predict the outcome variable. Lots of textbooks suggest that we should only be worried about this when colinearity is extremely high (e.g., correlations around > .70). However, studies have shown that even modest intercorrlations among predictor variables will reduce statistical power (Mason et al., 1991). Bottom line: If you can design a model where your predictor variables are relatively uncorrelated with each other, you can improve statistical power.
Conclusion
Increasing statistical power is one of the rare times where what is good for science, and what is good for your career actually coincides. It increases the accuracy and replicability of results, so it’s good for science. It also increases your likelihood of finding a statistically significant result (assuming the effect actually exists), making it more likely to get something published. You don’t need to torture your data with obsessive re-analysis until you get p < .05. Instead, put more thought into research design in order to maximize statistical power. Everyone wins, and you can use that time you used to spend sweating over p-values to do something more productive. Like volunteering with the Open Science Collaboration.
References
Aguinis, H., Beaty, J. C., Boik, R. J., & Pierce, C. A. (2005). Effect Size and Power in Assessing Moderating Effects of Categorical Variables Using Multiple Regression: A 30-Year Review. Journal of Applied Psychology, 90, 94-107. doi:10.1037/0021-9010.90.1.94
Button, K. S., Ioannidis, J. A., Mokrysz, C., Nosek, B. A., Flint, J., Robinson, E. J., & Munafò, M. R. (2013). Power failure: Why small sample size undermines the reliability of neuroscience. Nature Reviews Neuroscience, 14(5), 365-376. doi: 10.1038/nrn3475
Cohen, J. (1962). The statistical power of abnormal-social psychological research: A review. The Journal of Abnormal and Social Psychology, 65, 145-153. doi:10.1037/h0045186
Cohen, J. (1992). A power primer. Psychological Bulletin, 112, 155-159. doi:10.1037/0033-2909.112.1.155
Goodman, S. N., & Berlin, J. A. (1994). The use of predicted confidence intervals when planning experiments and the misuse of power when interpreting results. Annals of Internal Medicine, 121, 200-206.
Hansen, W. B., & Collins, L. M. (1994). Seven ways to increase power without increasing N. In L. M. Collins & L. A. Seitz (Eds.), Advances in data analysis for prevention intervention research (NIDA Research Monograph 142, NIH Publication No. 94-3599, pp. 184–195). Rockville, MD: National Institutes of Health.
MacCallum, R. C., Zhang, S., Preacher, K. J., & Rucker, D. D. (2002). On the practice of dichotomization of quantitative variables. Psychological Methods, 7, 19-40. doi:10.1037/1082-989X.7.1.19
Mason, C. H., & Perreault, W. D. (1991). Collinearity, power, and interpretation of multiple regression analysis. Journal of Marketing Research, 28, 268-280. doi:10.2307/3172863
Maxwell, S. E., Kelley, K., & Rausch, J. R. (2008). Sample size planning for statistical power and accuracy in parameter estimation. Annual Review of Psychology, 59, 537-563. doi:10.1146/annurev.psych.59.103006.093735
McClelland, G. H. (1997). Optimal design in psychological research. Psychological Methods, 2, 3-19. doi:10.1037/1082-989X.2.1.3
McClelland, G. H. (2000). Increasing statistical power without increasing sample size. American Psychologist, 55, 963-964. doi:10.1037/0003-066X.55.8.963
Mone, M. A., Mueller, G. C., & Mauland, W. (1996). The perceptions and usage of statistical power in applied psychology and management research. Personnel Psychology, 49, 103-120. doi:10.1111/j.1744-6570.1996.tb01793.x
Open Science Collaboration. (in press). The Reproducibility Project: A model of large-scale collaboration for empirical research on reproducibility. In V. Stodden, F. Leisch, & R. Peng (Eds.), Implementing Reproducible Computational Research (A Volume in The R Series). New York, NY: Taylor & Francis. doi:10.2139/ssrn.2195999
Richard, F. D., Bond, C. r., & Stokes-Zoota, J. J. (2003). One Hundred Years of Social Psychology Quantitatively Described. Review of General Psychology, 7, 331-363. doi:10.1037/1089-2680.7.4.331
Oct 25, 2013
by
Frank Farach
This is the first installment of the Open Science Toolkit, a recurring
feature that outlines practical steps individuals and organizations can
take to make science more open and reproducible.
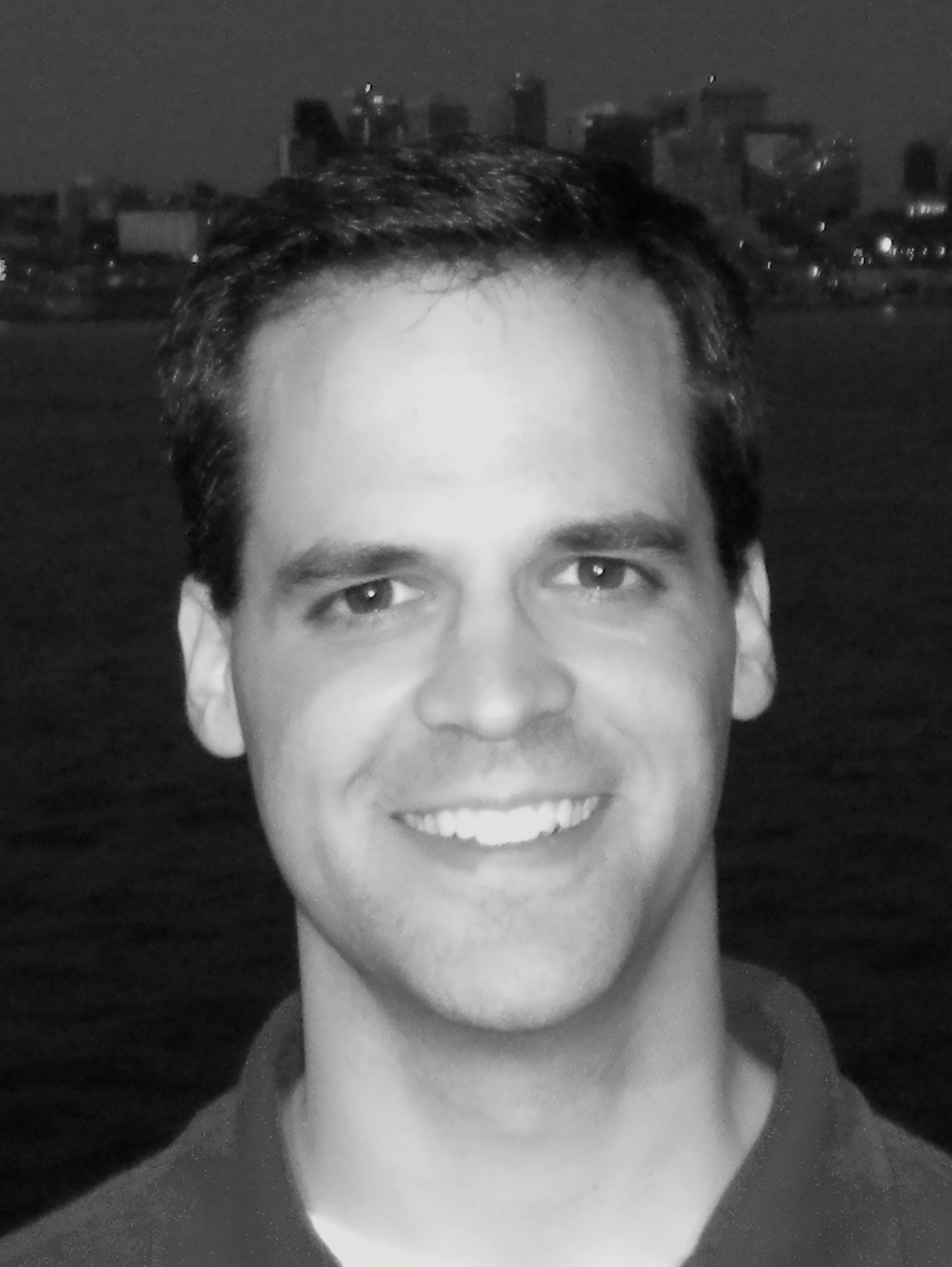
Congratulations! Your manuscript has been peer reviewed and accepted for
publication in a journal. The journal is owned by a major publisher who
wants you to know that, for $3,000, you can make your article open
access (OA) forever. Anyone in the world with access to the Internet
will have access to your article, which may be cited more often because
of its OA status. Otherwise, the journal would be happy to make your
paper available to subscribers and others willing to pay a fee.
Does this sound familiar? It sure does to me. For many years, when I
heard about Open Access (OA) to scientific research, it was always about
making an article freely available in a peer-reviewed journal -- the
so-called “gold” OA option -- often at considerable expense. I liked the
idea of making my work available to the widest possible audience, but
the costs were too prohibitive.
As it turns out, however, the “best-kept
secret” of OA is
that you can make your work OA for free by self-archiving it in an OA
repository, even if it has already been published in a non-OA journal.
Such “green” OA is possible because many
journals
have already provided blanket permission for authors to deposit their
peer-reviewed manuscript in an OA repository.
The flowchart below shows how easy it is to make your prior work OA. The
key is to make sure you follow the self-archiving policy of the journal
your work was published in, and to deposit the work in a suitable
repository.
Click to enlarge.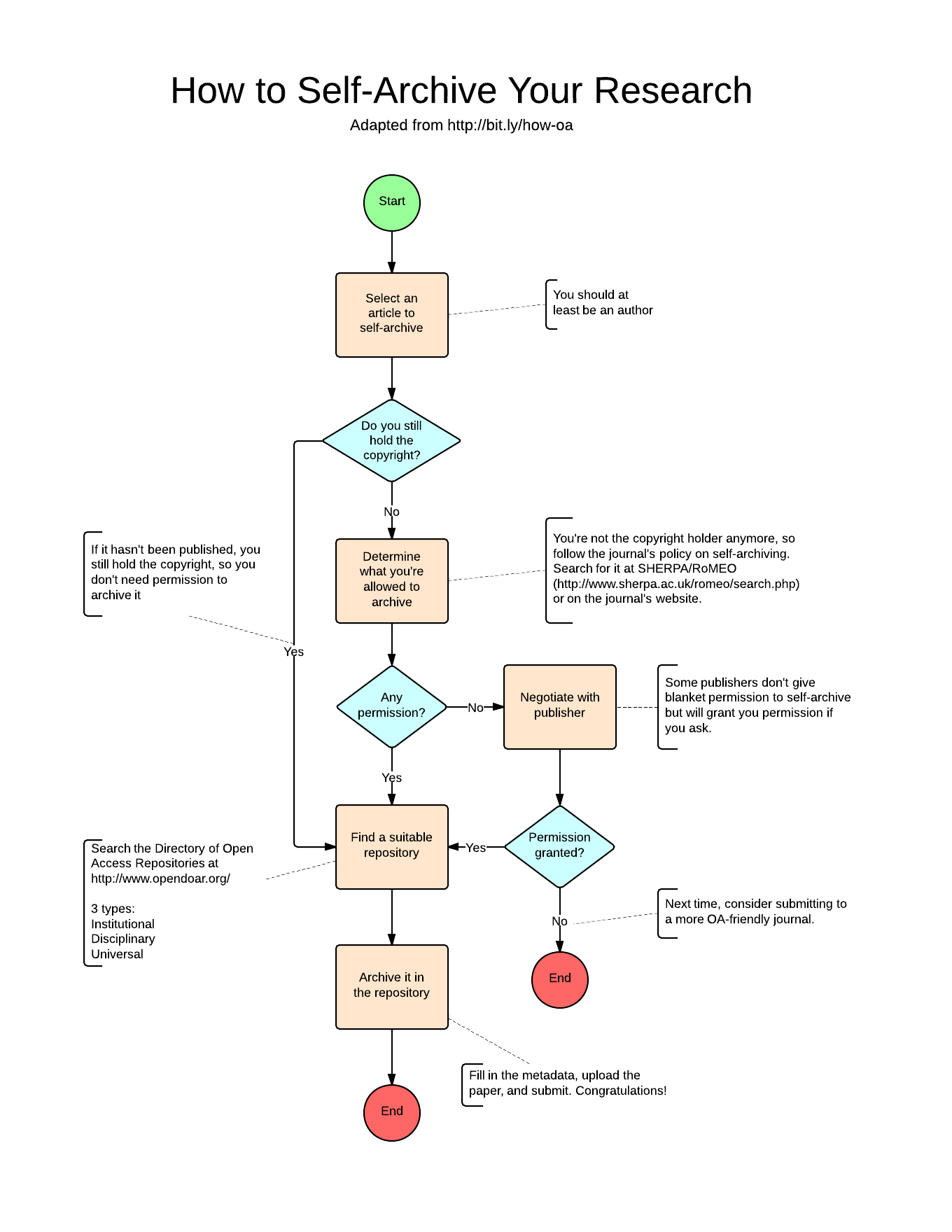
Journals typically display their self-archiving and copyright policies
on their websites, but you can also search for them in the
SHERPA/RoMEO database, which has a
nicely curated collection of policies from 1,333 publishers. The
database assigns a code to journals based on how far in the publication
process their permissions extend. It distinguishes between a pre-print,
which is the version of the manuscript before it underwent peer review,
and a post-print, the peer-reviewed version before the journal
copyedited and typeset it. Few journals allow you to self-archive their
copyedited PDF version of your article, but many let you do so for the
pre-print or post-print version. Unfortunately, some journals don’t
provide blanket permission for self-archiving, or require you to wait
for an embargo period to end before doing so. If you run into this
problem, you should contact the journal and ask for permission to
deposit the non-copyedited version of your article in an OA repository.
It has also become easy to find a suitable OA repository in which to
deposit your work. Your first stop should be the Directory of Open
Access Repositories (OpenDOAR), which
currently lists over 2,200 institutional, disciplinary, and universal
repositories. Although an article deposited in an OA repository will be
available to anyone with Internet access, repositories differ in feature
sets and policies. For example, some repositories, like
figshare, automatically assign a
CC BY license to all
publicly shared papers; others, like Open
Depot, allow you to choose a
license before making the article
public. A good OA repository will tell you how it ensures the long-term
digital preservation of your content as well as what metadata it exposes
to search engines and web services.
Once you’ve deposited your article in an OA repository, consider making
others aware of its existence. Link to it on your website, mention it on
social media, or add it to your CV.
In honor of Open Access Week, I am issuing a “Green OA Challenge” to
readers of this blog who have published at least one peer-reviewed
article. The challenge is to self-archive one of your articles in an OA
repository and link to it in the comments below. Please also feel free
to share any comments you have about the self-archiving process or about
green OA. Happy archiving!
Oct 16, 2013
Brian A. Nosek
University of Virginia, Center for Open Science
Jeffrey R. Spies
Center for Open Science
Last fall, the present first author taught a graduate class called
“Improving (Our) Science” at the University of Virginia. The class
reviewed evidence suggesting that scientific practices are not operating
ideally and are damaging the reproducibility of publishing findings. For
example, the power of an experimental design in null hypothesis
significance testing is a function of the effect size being investigated
and the size the sample to test it—power is greater when effects are
larger and samples are bigger. In the authors’ field of psychology, for
example, estimates suggest that the power of published studies to detect
an average effect size is .50 or less (Cohen, 1962; Gigerenzer &
Sedlmeier, 1989). Assuming that all of the published effects are true,
approximately 50% of published studies would reveal positive results
(i.e., p < .05 supporting the hypothesis). In reality, more than 90%
of published results are positive (Sterling, 1959; Fanelli,
2010).
How is it possible that the average study has power to detect the
average true effect 50% of the time or less and yet does so about 90% of
the time? It isn’t. Then how does this occur? One obvious contributor is
selective reporting. Positive effects are more likely than negative
effects to be submitted and accepted for publication (Greenwald, 1975).
The consequences include [1] the published literature is more likely to
exaggerate the size of true effects because with low powered designs
researchers must still leverage chance to obtain a large enough effect
size to produce a positive result; and [2] the proportion of false
positives – there isn’t actually an effect to detect – will be inflated
beyond the nominal alpha level of 5% (Ioannidis, 2005).
The class discussed this and other scientific practices that may
interfere with knowledge accumulation. Some of the relatively common
ones are described in Table 1 along with some solutions that we, and
others, identified. Problem. Solution. Easy. The class just fixed
science. Now, class members can adopt the solutions as best available
practices. Our scientific outputs will be more accurate, and significant
effects will be more reproducible. Our science will be better.
Alex Schiller, a class member and graduate student, demurred. He agreed
that the new practices would make science better, but disagreed that we
should do them all. A better solution, he argued, is to take small
steps: adopt one solution, wait for that to become standard scientific
practice, and then adopt another solution.
We know that some of our practices are deficient, we know how to improve
them, but Alex is arguing that we shouldn’t implement all the solutions?
Alex’s lapse of judgment can be forgiven—he’s just a graduate student.
However, his point isn’t a lapse. Faced with the reality of succeeding
as a scientist, Alex is right.
Table 1
Scientific practices that increase irreproducibility of
published findings, possible solutions, and barriers that prevent
adoption of those solutions
Practice |
Problem |
Possible Solution |
Barrier to Solution |
Run many low-powered studies rather than few high-powered studies |
Inflates false positive and false negative rates |
Run high-powered studies |
Non-significant effects are a threat to publishability; Risky to devote extra resources to high-powered tests that might not produce significant effects |
Report significant effects and dismiss non-significant effects as methodologically flawed |
Using outcome to evaluate method is a logical error and can inflate false positive rate |
Report all effects with rationale for why some should be ignored; let reader decide |
Non-significant and mixed effects are a threat to publishability |
Analyze during data collection, stop when significant result is obtained or continue until significant result is obtained |
Inflates false positive rate |
Define data stopping rule in advance |
Non-significant effects are a threat to publishability |
Include multiple conditions or outcome variables, report the subset that showed significant effects |
Inflates false positive rate |
Report all conditions and outcome variables |
Non-significant and mixed effects are a threat to publishability |
Try multiple analysis strategies, data exclusions, data transformations, report cleanest subset |
Inflates false positive rate |
Prespecify data analysis plan, or report all analysis strategies |
Non-significant and mixed effects are a threat to publishability |
Report discoveries as if they had resulted from confirmatory tests |
Inflates false positive rate |
Pre-specify hypotheses; Report exploratory and confirmatory analyses separately |
Many findings are discoveries, but stories are nicer and scientists seem smarter if they had thought it in advance |
Never do a direct replication |
Inflates false positive rate |
Conduct direct replications of important effects |
Incentives are focused on innovation, replications are boring; Original authors might feel embarrassed if their original finding is irreproducible |
Note: For reviews of these practices and their effects see Ioannidis,
2005; Giner-Sorolla, 2012; Greenwald, 1975; John et al.,
2012; Nosek et
al., 2012; Rosenthal,
1979; Simmons et al., 2011; Young et al., 2008
The Context
In an ideal world, scientists use the best available practices to
produce accurate, reproducible science. But, scientists don’t live in an
ideal world. Alex is creating a career for himself. To succeed, he must
publish. Papers are academic currency. They are Alex’s ticket to job
security, fame, and fortune. Well, okay, maybe just job security. But,
not everything is published, and some publications are valued more than
others. Alex can maximize his publishing success by producing particular
kinds of results. Positive effects, not negative effects (Fanelli,
2010;
Sterling, 1959). Novel effects, not verifications of prior effects (Open
Science Collaboration, 2012). Aesthetically appealing, clean results,
not results with ambiguities or inconsistencies (Giner-Sorolla, 2012).
Just look in the pages of Nature, or any other leading journal, they
are filled with articles producing positive, novel, beautiful results.
They are wonderful, exciting, and groundbreaking. Who wouldn’t want
that?
We do want that, and science advances in leaps with groundbreaking
results. The hard reality is that few results are actually
groundbreaking. And, even for important research, the results are often
far from beautiful. There are confusing contradictions, apparent
exceptions, and things that just don’t make sense. To those in the
laboratory, this is no surprise. Being at the frontiers of knowledge is
hard. We don’t quite know what we are looking at. That’s why we are
studying it. Or, as Einstein said, “If we knew what we were doing, it
wouldn’t be called research”.
But, those outside the laboratory get a different impression. When the
research becomes a published article, much of the muck goes away. The
published articles are like the pictures of this commentary’s authors at
the top of this page. Those pictures are about as good as we can look.
You should see the discards. Those with insider access know, for
example, that we each own three shirts with buttons and have highly
variable shaving habits. Published articles present the best-dressed,
clean-shaven versions of the actual work.
Just as with people, when you replicate effects yourself to see them in
person, they may not be as beautiful as they appeared in print. The
published version often looks much better than reality. The effect is
hard to get, dependent on a multitude of unmentioned limiting
conditions, or entirely irreproducible (Begley & Ellis, 2012; Prinz et
al., 2011).
The Problem
It is not surprising that effects are presented in their best light.
Career advancement depends on publishing success. More beautiful looking
results are easier to publish and more likely to earn rewards
(Giner-Sorolla, 2012). Individual incentives align for maximizing
publishability, even at the expense of accuracy (Nosek et al.,
2012).
Consider three hypothetical papers shown in Table 2. For all three, the
researchers identified an important problem and had an idea for a novel
solution. Paper A is a natural beauty. Two well planned studies showed
effects supporting the idea. Paper B and Paper C were conducted with
identical study designs. Paper B is natural, but not beautiful; Paper
C is a manufactured beauty. Both Paper B and Paper C were based on 3
studies. One study for each showed clear support for the idea. A second
study was a mixed success for Paper B, but “worked” for Paper C after
increasing the sample size a bit and analyzing the data a few different
ways. A third study did not work for either. Paper B reported the
failure with an explanation for why the methodology might be to blame,
rather than the idea being incorrect. The authors of Paper C generated
the same methodological explanation, categorized the study as a pilot,
and did not report it at all. Also, Paper C described the final sample
sizes and analysis strategies, but did not mention that extra data was
collected after initial analysis, or that alternative analysis
strategies had been tried and dismissed.
Table 2
Summary of research practices for three hypothetical papers
Step |
Paper |
Paper B |
Paper C |
Data collection |
Conducted two studies |
Conducted three studies |
Conducted three studies |
Data analysis |
Analyzed data after completing data collection following a pre-specified analysis plan |
Analyzed data after completing data collection following a pre-specified analysis plan |
Analyzed during data collection and collected more data to get to significance in one case. Selected from multiple analysis strategies for all studies. |
Result reporting |
Reported the results of the planned analyses for both studies |
Reported the results of the planned analyses for all studies |
Reported results of final analyses only. Did not report one study that did not reach significance. |
Final paper |
Two studies demonstrating clear support for idea |
One study demonstrating clear support for idea, one mixed, one not at all |
Two studies demonstrating clear support for idea |
Paper A is clearly better than Paper B. Paper A should be
published in a more prestigious outlet and generate more attention and
accolade. Paper C looks like Paper A, but in reality it is
like Paper B. The actual evidence is more circumspect than the
apparent evidence. Based on the report, however, no one can tell the
difference between Paper A and Paper C.
Two possibilities would minimize the negative impact of publishing
manufactured beauties like Paper C. First, if replication were
standard practice, then manufactured effects would be identified
rapidly. However, direct replication is very uncommon (Open Science
Collaboration, 2012). Once an effect is the literature, there is little
systematic ethic to self-correct. Rather than be weeded out, false
effects persist or just slowly fade away. Second, scientists could just
avoid doing the practices that lead to Paper C making this
illustration an irrelevant hypothetical. Unfortunately, a growing body
of evidence suggests that these practices occur, and some are even
common (e.g., John et al., 2012).
To avoid the practices that produce Paper C, the scientist must be
aware of and confront a conflict-of-interest—what is best for science
versus what is best for me. Scientists have inordinate opportunity to
pursue flexible decision-making in design and analysis, and there is
minimal accountability for those practices. Further, humans’ prodigious
motivated reasoning capacities provide a way to decide that the outcomes
that look best for us also has the most compelling rationale (Kunda,
1990). So, we may convince ourselves that the best course of action for
us was the best course of action period. It is very difficult to stop
doing suspect practices when we have thoroughly convinced ourselves that
we are not doing them.
The Solution
Alex needs to publish to succeed. The practices in Table 1 are to the
scientist, what steroids are to the athlete. They amplify the likelihood
of success in a competitive marketplace. If others are using, and Alex
decides to rely on his natural performance, then he will disadvantage
his career prospects. Alex wants to do the best science he can and be
successful for doing it. In short, he is the same as every other
scientist we know, ourselves included. Alex shouldn’t have to make a
choice between doing the best science and being successful—these should
be the same thing.
Is Alex stuck? Must he wait for institutional regulation, audits, and
the science police to fix the system? In a regulatory world, practices
are enforced and he need not worry that he’s committing career suicide
by following them. Many scientists are wary of a strong regulatory
environment in science, particularly for the possibility of stifling
innovation. Some of the best ideas start with barely any evidence at
all, and restrictive regulations on confidence in outputs could
discourage taking risks on new ideas. Nonetheless, funders, governments,
and other stakeholders are taking notice of the problematic incentive
structures in science. If we don’t solve the problem ourselves,
regulators may solve them for us.
Luckily, Alex has an alternative. The practices in Table 1 may be
widespread, but the solutions are also well known and endorsed as good
practice (Fuchs et al., 2012). That is, scientists easily understand the
differences between Papers A, B, and C – if they have full access to how
the findings were produced. As a consequence, the only way to be
rewarded for natural achievements over manufactured ones is to make the
process of obtaining the results transparent. Using the best available
practices privately will improve science but hurt careers. Using the
best available practices publicly will improve science while
simultaneously improving the reputation of the scientist. With openness,
success can be influenced by the results and by how they were
obtained.
Conclusion
The present incentives for publishing are focused on the one thing that
we scientists are absolutely, positively not supposed to control - the
results of the investigation. Scientists have complete control over the
design, procedures, and execution of the study. The results are what
they are.
A better science will emerge when the incentives for achievement align
with the things that scientists can (and should) control with their
wits, effort, and creativity. With results, beauty is contingent on what
is known about their origin. Obfuscation of methodology can make ugly
results appear beautiful. With methodology, if it looks beautiful, it is
beautiful. The beauty of methodology is revealed by openness.
Most scientific results have warts. Evidence is halting, uncertain,
incomplete, confusing, and messy. It is that way because scientists are
working on hard problems. Exposing it will accelerate finding solutions
to clean it up. Instead of trying to make results look beautiful when
they are not, the inner beauty of science can be made apparent. Whatever
the results, the inner beauty—strong design, brilliant reasoning,
careful analysis—is what counts. With openness, we won’t stop aiming for
A papers. But, when we get them, it will be clear that we earned them.
References
Begley, C. G., & Ellis, L. M. (2012). Raise standards for preclinical
cancer research. Nature, 483, 531-533.
Cohen , J. (1962). The statistical power of abnormal-social
psychological research: A review. Journal of Abnormal and Social
Psychology, 65, 145-153.
Fanelli, D. (2010). "Positive" results increase down the hierarchy of
the sciences. PLoS ONE, 5(4), e10068.
doi:10.1371/journal.pone.0010068
Fuchs H., Jenny, M., & Fiedler, S. (2012). Psychologists are open to
change, yet wary of rules. Perspectives on Psychological Science, 7,
634-637. doi:10.1177/1745691612459521
Sedlmeier, P., & Gigerenzer, G. (1989). Do studies of statistical power
have an effect on the power of studies? Psychological Bulletin, 105,
309-316.
Giner-Sorolla, R. (2012). Science or art? How esthetic standards grease
the way through the publication bottleneck but undermine science.
Perspectives on Psychological Science.
Greenwald, A. G. (1975). Consequences of prejudice against the null
hypothesis. Psychological Bulletin, 82, 1-20.
Ioannidis, J. P. A. (2005). Why most published research findings are
false. PLoS Medicine, 2, e124.
John, L., Loewenstein, G., & Prelec, D. (2012). Measuring the prevalence
of questionable research practices with incentives for truth-telling.
Psychological Science, 23, 524-532.
doi:10.1177/0956797611430953
Kunda, Z. (1990). The case for motivated reasoning. Psychological
Bulletin, 108, 480-498. doi:10.1037/0033-2909.108.3.480
Nosek, B. A., Spies, J. R., & Motyl, M. (2012). Scientific utopia: II.
Restructuring incentives and practices to promote truth over
publishability. Perspectives on Psychological Science, 7,615-631.
doi:10.1177/1745691612459058
Open Science Collaboration. (2012). An open, large-scale, collaborative
effort to estimate the reproducibility of psychological science.
Perspectives on Psychological Science, 7, 657-660.
doi:10.1177/1745691612462588
Prinz, F., Schlange, T. & Asadullah, K. (2011). Believe it or not: how
much can we rely on published data on potential drug targets? Nature
Reviews Drug Discovery, 10, 712-713.
Rosenthal, R. (1979). The file drawer problem and tolerance for null
results. Psychological Bulletin, 86, 638-641.
doi:10.1037/0033-2909.86.3.638
Simmons, J. P., Nelson, L. D., & Simonsohn, U. (2011). False-positive
psychology: Undisclosed flexibility in data collection and analysis
allows presenting anything as significant. Psychological Science, 22,
1359-1366.
Sterling, T. D. (1959). Publication decisions and their possible effects
on inferences drawn from tests of significance - or vice versa. Journal
of the American Statistical Association, 54, 30-34.
Young, N. S., Ioannidis, J. P. A., & Al-Ubaydli, O. (2008). Why current
publication practices may distort science. PLoS Medicine, 5,
1418-1422.
Oct 10, 2013
by
Jon Grahe, Pacific Lutheran University
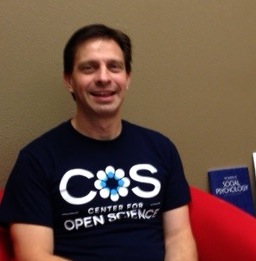
As a research methods instructor, I encourage my students to conduct “authentic” research projects. For now, consider authentic undergraduate research experiences as student projects at any level where the findings might result in a conference presentation or a publishable paper. This included getting IRB approval and attempts to present our findings at regional conferences, maybe including a journal submission. Eventually, I participated in opportunities for my students to contribute to big science by joining in two crowd-sourcing projects organized by Alan Reifman. The first was published in Teaching of Psychology (School Spirit Study Group, 2004) as an example for others to follow. The data included samples from 22 research methods classes who measured indices representative of the group name. Classes evaluated the data from their own school and the researchers collapsed the data to look at generalization across institutions. The second collaborative project included surveys collected by students in 10 research methods classes. The topic was primarily focused on emerging adulthood and political attitudes, but included many other psychological constructs. Later, I note the current opportunities for Emerging Adulthood theorists to use these data for a special issue of the journal, Emerging Adulthood. These two projects notwithstanding, there have been few open calls for instructors to participate in big science. Instructors who want to include authentic research experiences do so by completing all their own legwork as characterized by Frank and Saxe (2012) or as displayed by many of the poster presentations that you see at Regional Conferences.
However, that state of affairs has changed. Though instructors are likely to continue engaging in authentic research in their classrooms, they don’t have to develop the projects on their own anymore. I am very excited about the recent opportunities that allow students to contribute to “big science” by acting as crowd-sourcing experimenters. In full disclosure, I acknowledge my direct involvement in developing three of the following projects. However, I will save you a description of my own pilot test called the Collective Undergraduate Research Project (Grahe, 2010). Instead, I will briefly review recent “open invitation projects”, those where any qualified researcher can contribute. The first to emerge (Psych File Drawer, Reproducibility Project) were focused on PhD level researchers. However, since August 2012 there have been three research projects that specifically invite students to help generate data either as experimenters or as coders. More are likely to emerge soon as theorists grasp the idea that others can help them collect data.
This is a great time to be a research psychologist. These projects provide real opportunities for students or other researchers at any expertise level to get involved in not only authentic, but transformative research opportunities. The Council of Undergraduate Research recently published an edited volume (Karukstis & Hensel, 2010) dedicated to fostering transformative research for undergraduates. According to Wikipedia, “Transformative research is a term that became increasingly common within the science policy community in the 2000s for research that shifts or breaks existing scientific paradigms.” I consider open invitation projects transformative because they change the way that we view minimally acceptable standards for research. Each one is intended to change the basic premise of collaboration by bridging not only institutions, but also the chasm of acquaintance. Any qualified researcher can participate in data collection and authorship. Now, when I introduce research opportunities to my students, the ideas are grand. Here are research projects with grand ideas that invite contributions.
Many Labs Project - The Many Labs Team’s original invitation to contributors, sent out in February 2013 asked contributors to join their “wide-scale replication project {that was} conditionally accepted for publication in the special issue of Social Psychology.” They made a follow-up invitation in July reminding us of their Oct. 1st, 2013 deadline for data collection. This deadline limits future contributors, but it is a great example of a crowd-sourcing project. Their goal is to replicate 12 effects using the Project Implicit infrastructure. As is typical of these projects, contributors meeting a minimum goal (N > 80 cases in this instance) will be listed as coauthors in future publications. As they stated in their July post, “The project and accepted proposal has been registered on the Open Science Framework and can be found at this link: http://www.openscienceframework.org/project/WX7Ck/.” Richard Klein (project coordinator) reported that their project includes 18 different researchers at 15 distinct labs, with a plan for 38 labs contributing data before the deadline. This project is nearing the completion deadline and so new contributions are limited, but the project represents an important exemplar of potential projects.
Reproducibility Project – As stated on their invitation to new contributors on the Open Science Framework page, "Our primary goal is to conduct high quality replications of studies from three 2008 psychology journals and then compare our results to the original studies." As of right now, Johanna Cohoon from the Center for Open Science states, “We have 136 replication authors who come from 59 different institutions, with an additional 19 acknowledged replication researchers (155 replication researchers total). We also have an additional 40 researchers who are not involved in a replication that have earned authorship through coding/vetting 10 or more articles.” In short, this is a large collaboration and is welcoming more committed researchers. Though this project needs advanced researchers, it is possible for faculty to work closely with students who might wish to contribute.
PsychFileDrawer Project – This is less a collaborative effort between researchers than a compendium of replications, as stated by the project organizers: “PsychFileDrawer.org is a tool designed to address the File Drawer Problem as it pertains to psychological research: the distortion in the scientific literature that results from the failure to publish non-replications." It is collaborative in the sense that they host a “top 20” list of studies that the viewers want to see replicated. However, any researcher with a replication is invited to contribute the data. Further, although this was not initially targeted toward students, there is a new feature that allows contributors to identify a sample as a class project and the FAQ page asks instructors to comment on, “…the level and type of instructor supervision, and instructor’s degree of confidence in the fidelity with which the experimental protocol was implemented.”
Emerging Adulthood, Political Decisions, and More (2004) project – Alan Reifman is an early proponent of collaborative undergraduate research projects. After successfully guiding the School Spirit Study Group, he called again to research methods instructors to collectively conduct a survey that included two emerging adulthood scales, some political attitudes and intentions, and other scales that contributors wanted to add to the paper and pencil survey. By the time the survey was finalized, it was over 10 pages long and included hundreds of individual items measuring dozens of distinct psychological constructs on 12 scales. In retrospect, the survey was too long and the invitation to add on measures might have caused of the attrition of committed contributors that occurred. However, the final sample included over 1300 cases from 11 distinct institutions across the US. A list of contributors and initial findings can be found at Alan Reifman’s Emerging Adulthood Page. This project suffered from the amorphous structure of the collaboration. In short, no one instructor was interested in all the constructs. To resolve this situation where wonderfully rich data sit unanalyzed, the contributors are inviting emerging adulthood theorists to analyze the data and submit their work for a special issue of Emerging Adulthood. Details for how to request the data are available on the project’s OSF page. The deadline for a submission is July, 2014.
International Situations Project (ISP) – David Funder and Esther Guillaume-Hanes from the University of California—Riverside have organized a coalition of international researchers (19 and counting) to complete an internet protocol. As they describe on their about page, “Participants will describe situations by writing a brief description of the situation they experienced the previous day at 7 pm. They will identify situational characteristics uing the Riverside Situation Q-sort (RSQ) which includes 89 items that participants place into one of nine categories ranging from not at all characteristic to very characteristics. They then identify the behaviors they displayed using the Riverside Behavioral Q-Sort (RBQ) which includes 68 items using the same sorting procedure. The UC-Riverside researchers are taking primary responsibility for writing research reports. They have papers in print and others in preparation where all contributors who provide more than 80 cases are listed as authors. In Fall 2012, Psi Chi and Psi Beta encouraged their members to replicate the ISP in the US to create a series of local samples yielding 11 samples with 5 more committed contributors (Grahe, Guillaume-Hanes, & Rudmann, 2014). Currently, contributors are invited to complete either this project or their subsequent Personality project which includes completing this protocol, then completing the California Adult Q-Sort two weeks later. Interested researchers should contact Esther Guillaume directly at eguil002@ucr.edu.
Collaborative Replications and Education Project (CREP) – This project has recently started inviting contributions and is explicitly designed for undergraduates. Instructors who guide student research projects are encouraged to share the available studies list with their students. Hans IJzerman and Mark Brandt reviewed the top three cited empirical articles in the top journals in nine sub disciplines and rated them for feasibility to be completed by undergraduates selecting nine studies that were the most feasible. The project provides small ($200-$500) CREP research awards for completed projects (sponsored by Psi Chi and the Center for Open Science). Contributors will be encouraged/supported in writing and submitting reports for publication by the project coordinators. Anyone interested in participating should contact Jon Grahe (graheje@plu.edu).
Archival Project – This Center for Open Science project is also specifically designed as a crowd-sourcing opportunity for students. When it completes the beta-testing phase, the Archival Project will be publicly advertised. Unlike all the projects reviewed thus far, this project asks contributors to serve as coders rather than act as experimenters. It is a companion project to the OSC Reproducibility Project in that the target articles are from the same three Journals from the first three months of 2008. This project has a low bar for entry as training can take little time (particularly with the now available online tutorial) and coders can code as few as a single article and still make a real contribution. However, this project also has a system of honors as they state on their “getting involved” page: “Contributors who provide five or more accurate codings will be listed as part of the collective authorship.” This project was designed with the expectation that instructors will find the opportunity pedagogically useful and that they will employ it as a course exercise. Alternatively, students in organized clubs (such as Psi Chi) are invited to participate to increase their own methodological competence while simultaneously accumulating evidence of their contributions to an authentic research project. Finally, graduate students are invited to participate without faculty supervision. Interested parties should contact Johanna Cohoon (johannacohoon@gmail.com) for more information.
Future Opportunities – While this is intended to be an exhaustive list of open invitation projects, the field is not static and this list is likely to grow. What is exciting is that we now have ample opportunities to participate in “big science” with relatively small contributions. When developed with care, these projects follow Grahe et al. (2012)’s recommendation to take advantage of the magnitude of research being conducted each year by psychology students. The bar for entry varies in these projects from relatively intensive (e.g. Reproducibility Project, CREP) to relatively easy (e.g. Archival Project, ISP), providing opportunities for individuals with varying resources, from graduate students and PhD level researchers capable of completing high quality replications to students and instructors who seek opportunities in the classroom. Beyond the basic opportunity to participate in transformative research, these projects provide exemplars for how future collaborative projects should be designed and managed.
This is surely an incomplete list of current or potential examples of crowd-sourcing research. Please share other examples as comments below. Further consider pointing out strengths, weaknesses, opportunities or threats that could emerge from collaborative research. Finally, any public statements about intentions to participate in this type of open science initiative are welcome.
References
Frank, M. C., & Saxe, R. (2012). Teaching replication. Perspectives On Psychological Science, 7(6), 600-604. doi:10.1177/1745691612460686
Grahe. J. E., Gullaume-Hanes, E., & Rudmann, J. (2014). Students collaborate to advance science: The International Situations Project. Council for Undergraduate Research Quarterly
Grahe, J. E., Reifman, A., Hermann, A. D., Walker, M., Oleson, K. C., Nario-Redmond, M., & Wiebe, R. P. (2012). Harnessing the undiscovered resource of student research projects. Perspectives On Psychological Science, 7(6), 605-607. doi:10.1177/1745691612459057
Karukstis, K. K., & Hensel, N. (2010) Transformative research at predominately undergraduate institutions.” Council of Undergraduate Research. Washington DC., USA.
Oct 4, 2013
by
Åse Innes-Ker
Before Open Science there was Open Access (OA) — a movement driven by the
desire to make published research publicly accessible (after all, the public
usually had paid for it), rather than hidden behind paywalls.
Open Access is, by now, its very own strong movement — follow for example Björn
Brembs if you want to know what is happening there — and
there are now nice Open Access journals, like PLOS and
Frontiers, which are
peer-reviewed and reputable. Some of these journals charge an article
processing fee for OA articles, but in many cases funders have introduced or
are developing provisions to cover these costs. (In fact, the big private
funder in Sweden INSISTS on it.)
But, as always, situations where there is money involved and targets who are
desperate (please please please publish my baby so I won’t perish) breed mimics
and cuckoos and charlatans, ready to game the new playing field to their
advantage. This is probably just a feature of the human condition (see
Triver’s “Folly of Fools”).
There are lists of potentially predatory Open Access journals — I have linked
some in on my private blog (Åse Fixes Science)
here
and
here.
Reputation counts. Buyers beware!
Demonstrating the challenges of this new marketplace, John Bohannon published
in Science (a decidedly not Open Access journal) a sting operation in which he
spoofed Open Access journals to test their peer-review system. The papers were
machine generated nonsense — one may recall the Sokal Hoax from the previous
Science Wars. One may also recall the classic Ceci paper from 1982, which made
the rounds again earlier this year (and I blogged about that one too - on my
other blog).
Crucially, all of Bohannon’s papers contained fatal flaws that a decent
peer-reviewer should catch. The big news? Lots of them did not (though PLOS
did). Here’s the Science article with
its commentary stream,
and a commentary from Retraction Watch).
This is, of course, interesting — and it is generating buzz. But, it is also
generating some negative reaction. For one, Bohannon did not include the
regular non-OA journals in his test, so the experiment lacks a control group,
which means we can make no comparison and draw no firm inferences from the
data. The reason he states (it is quoted on the Retraction Watch site) is the
very long turnaround times for regular journals, which can be months, even a
year (or longer, as I’ve heard). I kinda buy it, but this is really what is
angering the Open Access crowd, who sees this letter as an attempt to
implicate Open Access itself as the source of the problem. And, apart from
Bohannon not including regular journals in his test, Science published what he
wrote without peer reviewing it.
My initial take? I think it is important to test these things — to uncover the
flaws in the system, and also to uncover the cuckoos and the mimics and the
gamers. Of course, the problems in peer review are not solely on the shoulders
of Open Access — Diederik Stapel, and other frauds, published almost
exclusively in paywalled journals (including Science). The status of peer
review warrants its own scrutiny.
But, I think the Open Access advocates have a really important point to
make. As noted on Retraction Watch, Bohannon's study didn't include
non-OA journals, so it's unclear whether the peer-review problems he
identified in OA journals are unique to their OA status.
I’ll end by linking in some of the commentary that I have seen so far — and, of
course, we’re happy to hear your comments.
Oct 2, 2013
by
Denny Borsboom
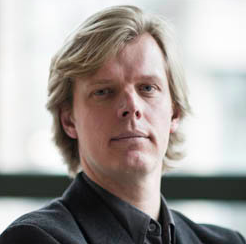
People used to smoke on airplanes. It's hard to imagine, but it's true. In less
than twenty years, smoking on airplanes has grown so unacceptable that it has
become difficult to see how people ever condoned it in the first place.
Psychological scientists used to refuse to share their data. It's not so hard to
imagine, and it's still partly true. However, my guess is that a few years from
now, data-secrecy will be as unimaginable as smoking on an airplane is today.
We've already come a long way. When in 2005 Jelte Wicherts, Dylan Molenaar,
Judith Kats, and I asked 141 psychological scientists to send us their raw data
to verify their analyses, many of them told us to get lost - even though, at
the time of publishing the research, they had signed an agreement to share
their data upon request. "I don't have time for this," one famous psychologist
said bluntly, as if living up to a written agreement is a hobby rather than a
moral responsibility. Many psychologists responded in the same way. If they
responded at all, that is.
Like Diederik Stapel.
I remember that Judith Kats, the student in our group who prepared the emails
asking researchers to make data available, stood in my office. She explained to
me how researchers had responded to our emails. Although many researchers
had refused to share data, some of our Dutch colleagues had done so in an
extremely colloquial, if not downright condescending way. Judith asked me how
she should respond. Should she once again inform our colleagues that they had
signed an APA agreement, and that they were in violation of a moral code?
I said no.
It's one of the very few things in my scientific career that I regret. Had we
pushed our colleagues to the limit, perhaps we would have been able to identify
Stapel's criminal practices years earlier. As his autobiography shows, Stapel
counterfeited his data in an unbelievably clumsy way, and I am convinced that
we would have easily identified his data as fake.
I had many reasons for saying no, which seemed legitimate at the time, but in
hindsight I think my behavior was a sign of adaptation to a defective research
culture. I had simply grown accustomed to the fact that, when I entered an
elevator, conversations regarding statistical analyses would fall silent. I took
it as a fact of life that, after we methodologists had explained students how to
analyze data in a responsible way, some of our colleagues would take it upon
themselves to show students how scientific data analysis really worked (today,
these practices are known as p-hacking). We all lived in a scientific version of
The Matrix, in which the reality of research was hidden from all - except those
who had the doubtful honor of being initiated. There was the science that people
reported and there was the science that people did.
In Groningen University, where Stapel used to work, he was known as The Lord
of the Data, because he never let anyone near his SPSS files. He pulled results
out of thin air, throwing them around as presents to his co-workers, and when
anybody asked him to show the underlying data files, he simply didn't respond.
Very few people saw this as problematic, because, hey, these were his data, and
why should Stapel share his data with outsiders?
That was the moral order of scientific psychology. Data are private property.
Nosy colleagues asking for data? Just chase them away, like you chase coyotes
from your farm. That is why researchers had no problem whatsoever denying
access to their data, and that is why several people saw the data-sharing request
itself as unethical. "Why don't you trust us?," I recall one researcher saying in a
suspicious tone of voice.
It is unbelievable how quickly things have changed.
In the wake of the Stapel case, the community of psychological scientists
committed to openness, data-sharing, and methodological transparency quickly
reached a critical mass. The Open Science Framework allows researchers to
archive all of their research materials, including stimuli, analysis code, and
data, to make them public by simply pressing a button. The new Journal of Open
Psychology Data offers an outlet
specifically designed to publish datasets, thereby giving these the status of a
publication. PsychDisclosure.org asks researchers to document decisions
regarding, e.g., sample size determination and variable selection, that were
left unmentioned in publications; most researchers provide this information
without hesitation - some actually do so voluntarily. The journal Psychological
Science will likely implement requirements for this type information in the
submission process. Data-archiving possibilities are growing like crazy. Major
funding institutes require data-archiving or are preparing regulations that do.
In the Reproducibility Project, hundreds of studies are being replicated in a
concerted effort. As a major symbol of these developments, we now have the
Center for Open Science, which facilitates the massive grassroots effort to
open up the scientific regime in psychology.
If you had told me that any of this would happen back in 2005, I would have
laughed you away, just as I would have laughed you away in 1990, had you told
me that the future would involve such bizarre elements as smoke-free airplanes.
The moral order of research in psychology has changed. It has changed for the
better, and I hope it has changed for good.
by
OSC
Welcome to the blog of the Open Science Collaboration! We are a loose network of researchers, professionals, citizen scientists, and others with an interest in open science, metascience, and good scientific practices. We’ll be writing about:
- Open science topics like reproducibility, transparency in methods and analyses, and changing editorial and publication practices
- Updates on open science initiatives like the Reproducibility Project and opportunities to get involved in new projects like the Archival Project
- Interviews and opinions from researchers, developers, publishers, and citizen scientists working to make science more transparent, rigorous, or reproducible.
We hope that the blog will stimulate open discussion and help improve the way science is done!
If you'd like to suggest a topic for a post, propose a guest post or column, or get involved with moderation, promotion, or technical development, we would love to hear from you! Email us at oscbloggers@gmail.com or tweet @OSCbloggers.
The OSC is an open collaboration - anyone is welcome to join, contribute to discussions, or develop a project. The OSC blog is supported by the Center for Open Science, a non-profit organization dedicated to increasing openness, integrity and reproducibility of scientific research.