Apr 2, 2015
by
See below
On March 19th and 20th, the Center for Open Science hosted a small meeting in Charlottesville, VA, convened by COS and co-organized by Kaitlin Thaney Mozilla Science Lab and Titus Brown (UC Davis). People working across the open science ecosystem attended, including publishers, infrastructure non-profits, public policy experts, community builders, and academics.
Open Science has emerged into the mainstream, primarily due to concerted efforts from various individuals, institutions, and initiatives. This small, focused gathering brought together several of those community leaders. The purpose of the meeting was to define common goals, discuss common challenges, and coordinate on common efforts.
We had good discussions about several issues at the intersection of technology and social hacking including badging, improving standards for scientific APIs, and developing shared infrastructure. We also talked about coordination challenges due to the rapid growth of the open science community. At least three collaborative projects emerged from the meeting as concrete outcomes to combat the coordination challenges.
A repeated theme was how to make the value proposition of open science more explicit. Why should scientists become more open, and why should institutions and funders support open science? We agreed that incentives in science are misaligned with practices, and we identified particular pain points and opportunities to nudge incentives. We focused on providing information about the benefits of open science to researchers, funders, and administrators, and emphasized reasons aligned with each stakeholders' interests. We also discussed industry interest in "open", both in making good use of open data, and also in participating in the open ecosystem. One of the collaborative projects emerging from the meeting is a paper or papers to answer the question “Why go open?” for researchers.
Many groups are providing training for tools, statistics, or workflows that could improve openness and reproducibility. We discussed methods of coordinating training activities, such as a training "decision tree" defining potential entry points and next steps for researchers. For example, Center for Open Science offers statistics consulting, rOpenSci offers training on tools, and Software Carpentry, Data Carpentry, and Mozilla Science Lab offer training on workflows. A federation of training services could be mutually reinforcing and bolster collective effectiveness, and facilitate sustainable funding models.
The challenge of supporting training efforts was linked to the larger challenge of funding the so-called "glue" - the technical infrastructure that is only noticed when it fails to function. One such collaboration is the SHARE project, a partnership between the Association of Research Libraries, its academic association partners, and the Center for Open Science. There is little glory in training and infrastructure, but both are essential elements for providing knowledge to enable change, and tools to enact change.
Another repeated theme was the "open science bubble". Many participants felt that they were failing to reach people outside of the open science community. Training in data science and software development was recognized as one way to introduce people to open science. For example, data integration and techniques for reproducible computational analysis naturally connect to discussions of data availability and open source. Re-branding was also discussed as a solution - rather than "post preprints!", say "get more citations!" Another important realization was that researchers who engage with open practices need not, and indeed may not want to, self-identify as "open scientists" per se. The identity and behavior need not be the same.
A number of concrete actions and collaborative activities emerged at the end, including a more coordinated effort around badging, collaboration on API connections between services and producing an article on best practices for scientific APIs, and the writing of an opinion paper outlining the value proposition of open science for researchers. While several proposals were advanced for "next meetings" such as hackathons, no decision has yet been reached. But, a more important decision was clear - the open science community is emerging, strong, and ready to work in concert to help the daily scientific practice live up to core scientific values.
People
Tal Yarkoni, University of Texas at Austin
Kara Woo, NCEAS
Tyler Walters, Virginia Tech and SHARE
Andrew Updegrove, Gesmer Updegrove and ConsortiumInfo.org
Kaitlin Thaney, Mozilla Science Lab
Jeffrey Spies, Center for Open Science
Courtney Soderberg, Center for Open Science
Elliott Shore, Association of Research Libraries
Andrew Sallans, Center for Open Science
Karthik Ram, rOpenSci and Berkeley Institute for Data Science
Min Ragan-Kelley, IPython and UC Berkeley
Brian Nosek, Center for Open Science and University of Virginia
Erin C, McKiernan, Wilfrid Laurier University
Jennifer Lin, PLOS
Amye Kenall, BioMed Central
Mark Hahnel, figshare
C. Titus Brown, UC Davis
Sara D. Bowman, Center for Open Science
Mar 6, 2015
by
Andrew A. Nelson
In following the recent cascade of online commentary and published literature surrounding the open science debate, I've noticed various attempts to situate the undergraduate role within this movement. One position, held by many, is best communicated in the following passages:
"Replications of new research should be performed by students as part of their coursework in experimental methods." (Frank & Saxe, 2004)
"...if students are encouraged to conduct replications as part of an effort to document and archive replications, collected classroom projects could contribute significantly to the field." (Grahe et al., 2012)
"...many academics already have set research agendas and may be unable to engage in the much-needed replication studies. In contrast, undergraduate students lack these prior commitments, creating the perfect opportunity for them to contribute to the scientific field while completing classroom requirements...When undergraduates get involved with research, everyone benefits..." (Grahe, Guillaume, & Rudmann, 2013)
"I am very excited about the recent opportunities that allow students to contribute to "big science" by acting as crowd-sourcing experimenters." ("Opportunities for Collaborative Research"; Grahe, 2013)
Clearly, open science proponents believe in the significance and necessity of undergraduate contributions to the movement. Even when reading between the lines, I take no offense to the implications made above; undergraduates do remain a valuable, blunt-force utility appropriate for open science's "dirty work", particularly when noting our lack of constraints (e.g. preexisting research agendas, reliance on funding, publication quotas, anxiety about tenure) relative to academics. And, though not included above, all of the aforementioned pieces make comparably frequent mentions of the reciprocal benefits to undergraduates when they participate in open science, suggesting that undergraduate involvement--even at the ground-level of replication and crowd-sourcing projects--really is advantageous to all.
Even so, discussions of these benefits are painfully vague and regularly short-ended; they are also, ironically, made by academics rather than undergraduates themselves. Acknowledging these shortcomings, below I briefly recount my own immersion in open science initiatives to firm up the discussion of undergraduate participation within the movement. I seek to frame these numerous open science avenues, both those directed at undergraduates and those open to all, as a critical educational tool, one fit for helping students meet the five "Learning Goals" outlined in the APA Guidelines for the Undergraduate Psychology Major (American Psychological Association, 2013). And while ultimately I vouch for an empirical pretest-posttest evaluation of my opinion--namely, that undergraduate participation in open science-based research projects prepares students to meet these learning goals above and beyond class-bracketed research--my own experiences will have to suffice for now.
Goal 1: Knowledge Base in Psychology
In their first learning goal, the APA calls for students to grasp the historical, theoretical and conceptual underpinnings of psychology and its comprising subfields. They understandably assume that this knowledge will largely come from course-taking, and I certainly don't dispute this assumption, especially when considering the distinct objectives for foundation level students (those who have completed no more than four course in the major) who may have no other extracurriculars (for example, Psi Chi or Psychology Club) from which to refine their knowledge base.
Yet it's important to remember that undergraduate coursework is generally constrained in its breadth and accessibility. More specifically, both the number of classes and the types of classes at an undergraduate's disposal can be gravely limited. I recall not having space in my schedule to take a well-regarded cognitive psychology class, and consequently, feeling poorly versed in the domain's theoretical framework and popular studies. Or, relatedly, hearing murmurings of human factors psychology and its inspiring applied nature, yet not having access to an elective dedicated to introducing that field.
In response to these limitations, I contend that The Center of Open Science's (COS) Archival Project is a remarkable educational opportunity for circumventing these limitations. This project recruits qualified undergraduates to "objectively assess the research and replication practices" of studies published in three of psychology's high-impact academic journals. Alongside its primary goal of deciphering the actual rate of replication within the discipline, The Archival Project represents a unique and meaningful chance for students to critically engage with literature spanning the array of psychological subfields.
I became involved with The Archival Project as a member of my institution's Psi Chi chapter, which was asked to pilot initial versions of the project's standardized article coding scheme. I concede without bitterness that this free, crowd-sourcing initiative forced me to thoroughly digest more psychological literature than the courses I was paying thousands of dollars to attend; doing so while contributing to groundbreaking science was an added bonus and motivator. Additionally, because the journals in question are broad in scope, I dabbled in biopsychology and neuropsychology readings that I may never have encountered otherwise. With confidence I can thus assert that my discipline-specific knowledge base was significantly bolstered by a project that is virtually open to all.
Goal 2: Scientific Inquiry and Critical Thinking
The second learning outcome specified by the APA involves the "the development of scientific reasoning and problem solving, including effective research methods." I foresee the assumption here being that class-mandated, independent projects are a suitable manner of meeting this learning goal, particularly when noting the substantial number of psychology majors required to participate in undergraduate research (Grahe et al., 2012). Again, I don't disagree with this kind of thinking, yet coupling this requirement with open science platforms makes only more sense.
Arguably, the typical undergraduate research experience, especially as a tool for instruction, contains several notable shortcomings. Even in its planning stage, student-driven research is limited by the depth of student's conceptual knowledge and the resources at her or his disposal. Furthermore, both planning and execution can be inhibited by the length of a quarter or semester, where simply getting IRB approval eats away at weeks of valuable data collection time. And thirdly, often a more definite endpoint exists--a final grade--and beyond that, many students' data seemingly don't matter much.
These potential drawbacks are precisely why I remain grateful for my own participation in a collective research paradigm, an experience so critical to my psychology-specific growth that I may have not pursued the discipline otherwise. Dr. Jon Grahe's Collective Undergraduate Research Project (a precursor to the current Collaborative Replications and Education Project) connected me with the project proposal of Dr. Fabian Ramseyer, a Swiss researcher seeking additional samples to test his objective gauge for dyadic movement coordination. (A brief overview of our work is viewable here.) I smile thinking back to the beginning days of our project, when--as a sophomore attempting to better understand Dr. Ramseyer's protocol and its underlying theory--I kept pronouncing "rapport" with a hard, annunciated "t" (thus "report"). And in a way, that's exactly the point. I knew absolutely nothing about the rapport construct and its behavioral correlates before this investigation, and class material never once spotlighted the topic. Just as I came to understand the ins and outs of dyadic rapport development via this open science opportunity, calls for undergraduate replication expose students to research concepts that are otherwise inconceivable, thereby broadening students' psychological knowledge. My collaboration with Dr. Ramseyer also allowed access to methodological tools (e.g. Motion Energy Analysis, the "objective gauge" mentioned above) literally unavailable elsewhere. When acknowledging the limited resources of a small, liberal arts university like my own, such accessibility can be especially empowering.
Also noteworthy is how the potential for "authentic research" (Grahe, "Opportunities for Collaborative Research" can inspire learning far beyond that of research conducted for coursework alone. Knowing that one's data matters--whether it will be collapsed into a larger, crowd-sourced dataset, used to validate previous findings, or employed to evaluate the reliability of a novel methodology--encourages meticulousness. And to be meticulous requires the cultivation of problem-solving and critical-thinking skills central to the APA's second learning goal. A humorous example of this is my own consideration of how swivel chairs (versus stationary desk chairs) might confound the type and frequency of movement synchronization between dyad members. When bringing my question to Dr. Ramseyer's attention, he exclaimed: "Your questions are proof that you have reached a good understanding of what is going on with MEA and video-analysis – congratulations!"
Goal 3: Ethical and Social Responsibility in a Diverse World
The APA's third undergraduate learning goal emphasizes student's awareness of their social and ethical responsibilities. As it applies to students completing their baccalaureate degree, this objective further encourages students to manifest these responsibilities in ways that "optimize" or "strengthen" their professional contributions. I commend the APA's inclusion of this criterion, specifically when noting the recent string of ethical breaches in psychology and beyond. The transparency, impartiality, and general foundation of open science makes it a special, yet underutilized option to hone undergraduates' understanding of scientific ethics.
That said, class-bracketed research might then be counterproductive. While students conduct research, they are concurrently refining their professional values and understanding of ethical science; doing so in a format that limits data-sharing and possible dissemination modes seems to then be sending the wrong message. Could it even be teaching bad science? Without doubt, it is minimizing students' potential contributions to the field.
I can speak from experience when saying that students quickly latch onto the drama of running their painstakingly-collected data through SPSS, crossing their fingers, and praying for results that support their hypotheses. Far too many, in consequence, are greeted with a lack of significance or something discouraging...and that momentary passion is indefinitely quelled. My head hung pretty low the countless times Dr. Ramseyer and I's data yielded nothing worthy of much excitement. With persistent encouragement from my advisor, however, I've shared our robust, vastly underexplored data on the Open Science Framework and via a data paper published in The Journal for Open Psychology Data.
Without hyperbole, I believe that the process of contributing to these open science platforms has forever shaped my values as a psychologist and my ethical commitment as a researcher. Firstly, I've come to frame my efforts in publicizing these data as, indeed, maximizing my contribution (given my resources and my data) to the field, a contribution that would be nearly impossible without the above-mentioned open science opportunities. Secondly, in sharing these data, I've had to also disseminate contextual and procedural information necessary for replication, potential collaboration, or data-checking. I now understand scientific transparency (alluded to in section 8.14 of the APA's Ethics Code) and encouraged replication to be paramount to good science. And most generally, along the way, I've accumulated knowledge about the current replication crisis, data falsification scandals, and other trends that verify the need for an open science network. As an example, choosing to post these data comes from my awareness of (and push back against) the "file drawer problem" (Rosenthal, 1979) plaguing modern science. I can almost guarantee that these current happenings in psychological science--and their interconnection with social and ethical responsibilities--are no more than alluded to in undergraduate psychology classes. This is why teaching open science is so crucial to plugging this educational hole and satisfying the APA's third undergraduate learning objective.
Goal 4: Communication
With their fourth learning goal, the APA insists that psychology majors should be able to effectively communicate through writing, presentations, and interpersonal discussion. My undergraduate experience dedicated a significant amount of time to sharpening my written communication and oral presentation skills, most often through in-class exercises or feedback on assignments. Yet, in learning other types of information exchange, students were left to fend for themselves. The point I hope to make below is that open science requires contributors to communicate via nontraditional mediums that are significant but seldom covered in class. Because these mediums ensure that students can maximize their scientific potential (through collaboration, data-sharing, and the like), they should be embraced and taught across all levels of higher education.
Primarily, I argue that open science is crucial to cushioning the otherwise intimidating prospect of undergraduate-initiated dialogue with graduate students or faculty. An indisputable point is that most undergraduates don't even know how to initiate these conversations, let alone how to sustain them without the fear of asking stupid questions or appearing ignorant. The neat thing about many open science opportunities is that they give students a figurative seat at the academic table, where the student-professor relationship can evolve into one that feels more collegial.
In my own journey, I distinctly recall finding my voice in the midst of my partnership with Dr. Ramseyer. Long-distance collaborations, particularly when using someone else's detailed protocol and associated software package, requires a heap of emails, all of which needed to be professional, clear, and concise. This partnership forced me to become a proficient communicator over email (something that many students could improve on), and additionally, boosted my confidence when talking with academic superiors. Relatedly, Dr. Grahe provided onsite supervision of this project, and our weekly meetings provided ample time to feel better about expressing my own opinion to--and even debating with--faculty. A fitting test for these developing skills occurred during a study abroad experience, when I took a side trip and visited Dr. Ramseyer in Bern. Amazingly, it felt as if we were able to pick up right where we left off over email, discussing potential updates for movement coordination software and his newfound interest in vocal pitch synchronization. Certainly I was nervous for that meeting in Switzerland, and I still get anxious before chats with faculty, however I attribute these communication improvements to my participation in open science projects.
And briefly, while the writing necessary to develop a project page with the Open Science Framework or draft a data paper with The Journal of Open Psychology Data remains unfamiliar to most undergraduates, it also represents a terrific opportunity for student writing to move beyond the generic "APA style manuscript" guidelines often imposed on it. Sure, I found these modes challenging to adapt to at first, yet I was reminded that proficient communication requires exactly that: adaptation to its setting. As we watch the tides of science change, it is important to expose students to mediums that are gaining traction and popularity within the discipline. Only then will students overwhelming meet the criteria included in this fourth goal.
Goal 5: Professional Development
In culmination, the APA's fifth learning objective asks programs to best equip their students with skills increasing their competitiveness for post-college employment or graduate school. Specifications of these abilities include the maturation of "self-efficacy and self-regulation," "project-management skills," enhancing one's "teamwork capacity," and the application of "psychology content and skills to career goals." While I understand the value in these skills for postbaccalaureate success, I also must note that undergraduate coursework (including research) usually serves as the end-goal through which these skills are taught. Few instructors devote intentional lesson plans to improving students' ability to work in a team or develop self-efficacy; instead, these abilities are learned along the way.
Consequently, the content of this fifth goal seems almost circular, especially to those aiming for graduate school or psychology-related employment. Consider the previous four objectives and my arguments for how open science opportunities might benefit learning above and beyond course-bracketed research projects. As students learn how to learn by building a psychology-specific knowledge base, boost their critical thinking skills through intensive scientific inquiry, augment their learning with the awareness of social and science-specific ethics, and polish their communication skills, they are developing abilities paramount to their professional development. And if their participation in open science only betters their development of such skills, then it seems almost imperative that students be exposed to these projects in a setting that, above all else, exists to ready students for their future careers.
I'd like to conclude by acknowledging the disagreements swirling around open science implementation. Debate is inherent to changing the status quo, and ultimately, I don't want to blame either side for their passions or viewpoints. Nonetheless, there's an underlying point here that seems far less disputable. Improving the quality of undergraduate education should be a priority to all, particularly to those same academics engaged in this debate. Can't we all agree on the educational capacity of a movement (call it "open science" if you wish) that stimulates undergraduate learning, in ways previously overlooked or inconceivable? The movement is ready. Now it's simply a matter of embracing it.
Works Cited
American Psychological Association. (2013). APA Guidelines for the Undergraduate Psychology Major. Retrieved from http://www.apa.org/ed/precollege/about/psymajor-guidelines.pdf.
Frank, M. C., & Saxe, R. (2012). Teaching replication. Perspectives on Psychological Science, 7(6), 600-604. doi:10.1177/1745691612460686
Grahe. J. E., Gullaume-Hanes, E., & Rudmann, J. (2013). Students collaborate to advance science: The International Situations Project. Council for Undergraduate Research Quarterly, 34(2), 4-9. http://www.cur.org/publications/curq_on_the_web/
Grahe, J. E., Reifman, A., Hermann, A. D., Walker, M., Oleson, K. C., Nario-Redmond, M., & Wiebe, R. P. (2012). Harnessing the undiscovered resource of student research projects. Perspectives on Psychological Science, 7(6), 605-607. doi:10.1177/1745691612459057
Rosenthal, R. (1979). The file drawer problem and tolerance for null results. Psychological Bulletin, 86(3), 638-641.
Jul 30, 2014
by
Sebastiaan Mathôt
A little more than three years ago I started working on OpenSesame, a free program for the easy development of experiments, mostly oriented at psychologists and neuroscientists. The first version of OpenSesame was the result of a weekend-long hacking sprint. By now, OpenSesame has grown into a substantial project, with a small team of core developers, tens of occasional contributors, and about 2500 active users.
Because of my work on OpenSesame, I've become increasingly interested in open-source software in general. How is it used? Who makes it? Who is crazy enough to invest time in developing a program, only to give it away for free? Well ... quite a few people, because open source is everywhere. Browsers like Firefox and Chrome. Operating systems like Ubuntu and Android. Programming languages like Python and R. Media players like VLC. These are all examples of open-source programs that many people use on a daily basis.
But what about specialized scientific software? More specifically: Which programs do experimental psychologists and neuroscientists use? Although this varies from person to person, a number of expensive, closed-source programs come to mind first: E-Prime, SPSS, MATLAB, Presentation, Brainvoyager, etc. Le psychonomist moyen is not really into open source.
In principle, there are open-source alternatives to all of the above programs. Think of PsychoPy, R, Python, or FSL. But I can imagine the frown on the reader's face: Come on, really? These freebies are not nearly as good as 'the real thing', are they? But this, although true to some extent, merely raises another question: Why doesn't the scientific community invest more effort in the development of open-source alternatives? Why do we keep accepting inconvenient licenses (no SPSS license at home?), high costs ($995 for E-Prime 2 professional), and scripts written in proprietary languages that cannot easily be shared between labs. This last point has become particularly relevant with the recent focus on replication and transparency. How do you perform a direct replication of an experiment if you do not have the required software? And what does transparency even mean if we cannot run each other's scripts?
Despite widespread skepticism, I suspect that most scientists feel that open source is ideologically preferable over proprietary scientific software. But open source suffers from an image problem. For example, a widely shared misconception is that open-source software is buggy, whereas proprietary software is solid and reliable. But even though quality is subjective--and due to cognitive dissonance strongly biased in favor of expensive software!--this belief is not consistent with reality: Reports have shown that open-source software contains about half as many errors per line of code as proprietary software.
Another misconception is that developing (in-house) open-source software is expensive and inefficient. This is essentially a prisoners dilemma. Of course, for an individual organization it is often more expensive to develop software than to purchase a commercial license. But what if scientific organizations would work together to develop the software that they all need: You write this for me, I write this for you? Would open source still be inefficient then?
Let's consider this by first comparing a few commercial packages: E-Prime, Presentation, and Inquisit. These are all programs for developing experiments. Yet the wheel has been re-invented for each program. All overlapping functionality has been re-designed and re-implemented anew, because vendors of proprietary software dislike few things as much as sharing code and ideas. (This is made painfully clear by numerous patent wars.) Now, let's compare a few open-source programs: Expyriment, OpenSesame, and PsychoPy. These too are all programs for developing experiments. And these too have overlapping functionality. But you can use these programs together. Moreover, they build on each other's functionality, because open-source licenses allow developers to modify and re-use each other's code. The point that I'm trying to make is not that open-source programs are better than their proprietary counterparts. Everyone can decide that for him or herself. The crucial point is that the development process of open-source software is collaborative and therefore efficient. Certainly in theory, but often in practice as well.
So it is clear that open-source software has many advantages, also--maybe even especially so--for science. Therefore, development of open-source software should be encouraged. How could universities and other academic organizations contribute to this?
A necessary first step is to acknowledge that software needs time to mature. There are plenty of young researchers, technically skilled and brimming with enthusiasm, who start a software project. Typically, this is software that they developed for their own research, and subsequently made freely available. If you are lucky, your boss allows this type of frivolous fun, as long the 'real' work doesn't suffer. And maybe you can even get a paper out of it, for example in Behavior Research Methods, Journal of Neuroscience Methods, or Frontiers in Neuroinformatics. But it is often forgotten that software needs to be maintained. Bugs need to be fixed. Changes in computers and operating systems require software updates. Unmaintained software spoils like an open carton of milk.
And this is where things get awkward, because universities don't like maintenance. Developing new software is one thing. That's innovation, and somewhat resembles doing research. But maintaining software after the initial development stage is over is not interesting at all. You cannot write papers about maintenance, and maintenance does not make an attractive grant proposal. Therefore, a lot of software ends up 'abandonware', unmaintained ghost pages on development sites like GitHub, SourceForge, or Google Code.
Ideally, universities would encourage maintenance of open-source scientific software. The message should be: Once you start something, go through with it. They should recognize that the development of high-quality software requires stamina. This would be an attitude change, and would require that universities get over their publication fetish. Because the value of a program is not in the papers that have been written about it, but in the scientists that use it. Open-source scientific software has a very concrete and self-evident impact for which developers should be rewarded. Without incentives, they won't make the high-quality software that we all need!
In other words, developers could use a bit of encouragement and support, and this is currently lacking. I recently attended the APS convention, where I met Jeffrey Spies, one of the founders of the Center for Open Science (COS). As readers of this blog probably know, the COS is an American organization that (among many other things) facilitates development of open-source scientific software. They provide advice, support promising projects, and build networks. (Social, digital, and a mix of both, like this blog!) A related organization that focuses more specifically on software development is the Mozilla Science Lab (MSL). I think that the COS and MSL do great work, and provide models that could be adopted by other organizations. For example, I currently work for the CNRS, the French organization for fundamental research. The CNRS is very large, and could easily provide sustained support for the development of high-quality open-source projects. And the European Research Council could easily do so as well. However, these large research organization do not appear to recognize the importance of software development. They prefer to invest all of their budget in individual research projects, rather than invest a small part of it in the development and maintenance of the software that these research projects need.
In summary, a little systematic support would do wonders for the quality and availability of open-source scientific software. Investing in the future, is that not what science is about?
A Dutch version of this article initially appeared in De Psychonoom, the magazine of the Dutch psychonomic society. This article has been translated and updated for the OSC blog.
Jul 16, 2014
by
Sara Bowman, Mallory Kidwell, and Erin Braswell
Scientific domains vary by the tools and instruments used, the way data are collected and managed, and even how results are analyzed and presented. As advocates of open science practices, it’s important that we understand the common obstacles to scientific workflow across many domains. The COS team visits scientists in their labs and out in the field to discuss and experience their research processes first-hand. We experience the day-to-day of researchers and do our own investigating. We find where data loss occurs, where there are inefficiencies in workflow, and what interferes with reproducibility. These field trips inspire new tools and features for the Open Science Framework to support openness and reproducibility across scientific domains.
Last week, the team visited the Monticello Department of Archaeology to dig a little deeper (bad pun) into the workflow of archaeologists, as well as learn about the Digital Archaeological Archive of Comparative Slavery (DAACS). Derek Wheeler, Research Archaeologist at Monticello, gave us a nice overview of how the Archaeology Department surveys land for artifacts. Shovel test pits, approximately 1 foot square, are dug every 40 feet on center as deep as anyone has dug in the past (i.e., down to undisturbed clay). If artifacts are found, the shovel test pits are dug every 20 feet on center. At Monticello, artifacts are primarily man-made items like nails, bricks or pottery. The first 300 acres surveyed contained 12,000 shovel test pits -- and that’s just 10% of the total planned survey area. That’s a whole lot of holes, and even more data.
Fraser Neiman, Director of Archaeology at Monticello, describes the work being done to excavate on Mulberry Row - the industrial hub of Jefferson’s agricultural industry.
At the Mulberry Row excavation site, Fraser Neiman, Director of Archaeology, explained the meticulous and painstaking process of excavating quadrats, small plots of land isolated for study. Within a quadrat, there exist contexts - stratigraphic units. Any artifacts found within a context are carefully recorded on a context sheet - what the artifact is, its location within the quadrat, along with information about the fill (dirt, clay, etc.) in the context. The fill itself is screened to pull out smaller artifacts the eye may not catch. All of the excavation and data collection at the Mulberry Row Reassessment is conducted following the standards of the Digital Archaeological Archive of Comparative Slavery (DAACS). Standards developed by DAACS help archaeologists in the Chesapeake region to generate, report, and compare data from 20 different sites across the region in a systematic way. Without these standards, archiving and comparing artifacts from different sites would be extremely difficult.
Researchers make careful measurements at the Monticello Mulberry Row excavation site, while recording data on a context sheet.
The artifacts, often sherds, are collected by context and taken to the lab for washing, labeling, analysis and storage. After washing, every sherd within a particular context is labeled with the same number and stored together. All of the data from the context sheets, as well as photos of the quadrants and sherds, are carefully input into DAACS following the standards set out in the DAACS Cataloging Manual. There is an enormous amount of manual labor associated with preparing and curating each artifact. Jillian Galle, Project Manager of DAACS, described the extensive training users must undergo in order to deposit their data in the archive to ensure the standards outlined by the Cataloging Manual are kept. This regimented process ensures the quality and consistency of the data- and thus its utility. The result is a publicly available dataset of the history of Monticello for researchers of all kinds to examine this important site in America’s history.
These sherds have been washed and numbered to denote their context.
Our trip to Monticello Archaeology was eye-opening, as none of us had any practical experience with archaeological research or data. The impressive DAACS protocols and standards represent an important aspect of all scientific research - the ability to accurately capture large amounts of data in a systematic, thoughtful way - and then share it freely with others.
Jul 10, 2014
by
Tom Stafford
Jason Mitchell's essay 'On the emptiness of failed replications' is notable for being against the current effort to publish replication attempts. Commentary on the essay that I saw was pretty negative (e.g. "awe-inspiringly clueless", “defensive pseudo-scientific, anti-Bayesian academic ass-covering”, "Do you get points in social psychology for publicly declaring you have no idea how science works?").
Although I reject his premises, and disagree with his conclusion, I don't think Mitchell's arguments are incomprehensibly mad. This seems to put me in a minority, so I thought I'd try and explain the value in what he's saying. I'd like to walk through his essay assuming he is a thoughtful rational person. Why would a smart guy come to the views he has? What is he really trying to say, and what are his assumptions about the world of psychology that might, perhaps, illuminate our own assumptions?
Experiments as artefacts, not samples
First off, key to Mitchell's argument is a view that experiments are complex artefacts, in the construction of which errors are very likely. Effects, in this view, are hard won, eventually teased out via a difficult process of refinement and validation. The value of replication is self-evident to anyone who thinks statistically: sampling error and publication bias will produce lots of false positives, you improve your estimate of the true effect by independent samples (= replications). Mitchell seems to be saying that the experiments are so complex that replications by other labs aren't independent samples of the same effect. Although they are called replications there are, he claims, most likely to be botched, and so informative of nothing more than the incompetence of the replicators.
When teaching our students many of us will have deployed the saying "The plural of anecdote is not data". What we mean by this is that many weak observations - of ghosts, aliens or psychic powers - do not combine multiplicatively to make strong evidence in favour of these phenomena. If I've read him right, Mitchell is saying the same thing about replication experiments - many weak experiments are uninformative about real effects.
Tacit practical knowledge
Part of Mitchell's argument rests on the importance of tacit knowledge in running experiments (see his section "The problem with recipe-following"). We all know that tacit knowledge about experimental procedures exists in science. Mitchell puts a heavy weight on the importance of this. This is a position which presumably would have lots of sympathy from Daniel Kahneman, who suggested that all replication attempts should involve the original authors.
There's a tension here between how science should be and how it is. Obviously our job is to make things explicit, to explain how to successfully run experiments so that anyone can run them but the truth is, full explanations aren't always possible. Sure, anyone can try and replicate based on a methods section, but - says Mitchell - you will probably be wasting your time generating noise rather than data, and shouldn't be allowed to commit this to the scientific record.
Most of us would be comfortable with the idea that if a non-psychologist ran our experiments they might make some serious errors (one thinks of the hash some physical scientists made of psi-experiments, failing completely to account for things like demand effects, for example). Mitchell's line of thought here seems to take this one step further, you can't run a social psychologist's experiments without special training in social psychology. Or even, maybe, you can't successfully run another lab's experiment without training from that lab.
I think happen to think he's wrong on this, and that he neglects to mention the harm of assuming that successful experiments have a "special sauce" which cannot be easily communicated (it seems to be a road to elitism and mysticism to me, completely contrary to the goals science should have). Nonetheless, there's definitely some truth to the idea, and I think it is useful to consider the errors we will make if we assume the contrary, that methods sections are complete records and no special background is required to run experiments.
Innuendo
Mitchell makes the claim that targeting an effect for replication amounts to the innuendo that the effects under inspection are unreliable, which is a slur on the scientists who originally published them. Isn't this correct? Several people on twitter admitted, or tacitly admitted, that their prior beliefs were that many of these effects aren't real. There is something disingenuous about claiming, on the one hand, that all effects should be replicated, but, on the other, targeting particular effects for attention. If you bought Mitchell's view that experiments are delicate artefacts which render most replications uninformative, you can see how the result is a situation which isn't just uninformative but actively harmful to the hard-working psychologists whose work is impugned. Even if you don't buy that view, you might think that selection of which effects should be the focus of something like the Many Labs project is an active decision made by a small number of people, and which targets particular individuals. How this processes works out in practice deserves careful consideration, even if everyone agrees that it is a Good Thing overall.
Caveats
There are a number of issues in Mitchell's essay I haven't touched on - this isn't meant to be a complete treatment, just an explanation of some of the reasonable arguments I think he makes. Even if I disagree with them, I think they are reasonable; they aren't as obviously wrong as some have suggested and should be countered rather than dismissed.
Stepping back, my take on the 'replication crisis' in psychology is that it really isn't about replication. Instead, this is what digital disruption looks like in a culture organised around scholarly kudos rather than profit. We now have the software tools to coordinate data collection, share methods and data, analyse data, and interact with non-psychologists, both directly and via the media, in unprecedented ways and at an unprecedented rate. Established scholarly communities are threatened as "the way things are done" is challenged. Witness John Bargh's incredulous reaction to having his work challenged (and note that this was 'a replicate and explain via alternate mechanism' type study that Mitchell says is a valid way of doing replication). Witness the recent complaint of medical researcher Jonathan S. Nguyen-Van-Tam when a journalist included critique of his analysis technique in a report on his work. These guys obviously believe in a set of rules concerning academic publishing which many of us aren't fully aware of or believe no longer apply.
By looking at other disrupted industries, such as music or publishing, we can discern morals for both sides. Those who can see the value in the old way of doing things, like Mitchell, need to articulate that value and fast. There's no way of going back, but we need to salvage the good things about tight-knit, slow moving, scholarly communities. The moral for the progressives is that we shouldn't let the romance of change blind us to the way that the same old evils will reassert themselves in new forms, by hiding behind a facade of being new, improved and more equitable.
Jul 9, 2014
by
Sean Mackinnon
Jason Mitchell recently wrote an article entitled “On the Emptiness of Failed Replications.”. In this article, Dr. Mitchell takes an unconventional and extremely strong stance against replication, arguing that: “… studies that produce null results -- including preregistered studies -- should not be published.” The crux of the argument seems to be that "scientists who get p > .05 are just incompetent." It completely ignores the possibility that a positive result could also (maybe even equally) be due to experimenter error. Dr. Mitchell also appears to ignore the possibility of simply getting a false positive (which is expected to happen under the null in 5% of cases).
More importantly, it ignores issues of effect size and treats the outcome of research as a dichotomous "success or fail.” The advantages of examining effect sizes over simple directional hypotheses using null hypothesis significance testing are beyond the scope of this short post, but you might check out Sullivan and Feinn (2012) as an open-access starting point. Generally speaking, the problem is that sampling variation means that some experiments will find null results even when the experimenter does everything right. As an illustration, below is 1000 simulated correlations, assuming that r = .30 in the population, and a sample size of 100 (I used a monte carlo method).
In this picture, the units of analysis are individual correlations obtained in 1 of 1000 hypothetical research studies. The x-axis is the value of the correlation coefficient found, and the y-axis is the number of studies reporting that value. The red line is the critical value for significant results at p < .05 assuming a sample size of 100. As you can see from this picture, the majority of studies are supportive of an effect that is greater than zero. However (simply due to chance) all the studies to the left of the red line turned out non-significant. If we suppressed all the null results (i.e., all those unlucky scientists to the left of the red line) as Dr. Mitchell suggests, then our estimate of the effect size in the population would be inaccurate; specifically, it would appear to be larger than it really is, because certain aspects of random variation (i.e., null results) are being selectively suppressed. Without the minority of null findings (in addition to the majority of positive findings) the overall estimate of the effect cannot be correctly estimated.
The situation is even more grim if there really is no effect in the population.
In this case, a small proportion of studies will produce false positives, with a roughly equal chance of an effect in either direction. If we fail to report null results, false positives may be reified as substantive effects. The reversal of signs across repeated studies might be a warning sign that the effect doesn’t really exist, but without replication, a single false positive could define a field if it happens (by chance) to be in line with prior theory.
With this in mind, I also disagree that replications are “publicly impugning the scientific integrity of their colleagues.” Some people feel threatened or attacked by replication. The ideas we produce as scientists are close to our hearts, and we tend to get defensive when they’re challenged. If we focus on effect sizes, rather than the “success or fail” logic of null hypothesis significance testing, then I don’t believe that “failed” replications damage the integrity of the original author, but rather simply suggests that we should modulate the estimate of the effect size downwards. In this framework, replication is less about “proving someone wrong” and more about centering on the magnitude of an effect size.
Something that is often missed in discussion of replication is that the very nature of randomness inherent in the statistical procedures scientists use means that any individual study (even if perfectly conducted) will probably generate an effect size that is a bit larger or smaller than it is in the population. It is only through repeated experiments that we are able to center on an accurate estimate of the effect size. This issue is independent of researcher competence, and means that even the most competent researchers will come to the wrong conclusions occasionally because of the statistical procedures and cutoffs we’ve chosen to rely on. With this in mind, people should be aware that a failed replication does not necessarily mean that one of the two researchers is incorrect or incompetent – instead, it is assumed (until further evidence is collected) that the best estimate is a weighted average of the effect size from each research study.
For some more commentary from other bloggers, you might check out the following links:
"Are replication efforts pointless?" by Richard Tomsett
"Being as wrong as can be on the so-called replication crisis of science" by drugmonkey at Scientopia
"Are replication efforts useless?" by Jan Moren
"Jason Mitchell’s essay" by Chris Said
"#MethodsWeDontReport – brief thought on Jason Mitchell versus the replicators" by Micah Allen
"On 'On the emptiness of failed replications'" by Neuroskeptic
Jul 2, 2014
by
Ryne Sherman
This article was originally posted in the author's personal blog.
Imagine you have a two group between-S study with N=30 in each group. You compute a two-sample t-test and the result is p = .09, not statistically significant with an effect size r = .17. Unbeknownst to you there is really no relationship between the IV and the DV. But, because you believe there is a relationship (you decided to run the study after all!), you think maybe adding five more subjects to each condition will help clarify things. So now you have N=35 in each group and you compute your t-test again. Now p = .04 with r = .21.
If you are reading this blog you might recognize what happened here as an instance of p-hacking. This particular form (testing periodically as you increase N) of p-hacking was one of the many data analytic flexibility issues exposed by Simmons, Nelson, and Simonshon (2011). But what are the real consequences of p-hacking? How often will p-hacking turn a null result into a positive result? What is the impact of p-hacking on effect size?
These were the kinds of questions that I had. So I wrote a little R function that simulates this type of p-hacking. The function – called phack – is designed to be flexible, although right now it only works for two-group between-S designs. The user is allowed to input and manipulate the following factors (argument name in parentheses):
- Initial Sample Size (initialN): The initial sample size (for each group) one had in mind when beginning the study (default = 30).
- Hack Rate (hackrate): The number of subjects to add to each group if the p-value is not statistically significant before testing again (default = 5).
- Population Means (grp1M, grp2M): The population means (Mu) for each group (default 0 for both).
- Population SDs (grp1SD, grp2SD): The population standard deviations (Sigmas) for each group (default = 1 for both).
- Maximum Sample Size (maxN): You weren’t really going to run the study forever right? This is the sample size (for each group) at which you will give up the endeavor and go run another study (default = 200).
- Type I Error Rate (alpha): The value (or lower) at which you will declare a result statistically significant (default = .05).
- Hypothesis Direction (alternative): Did your study have a directional hypothesis? Two-group studies often do (i.e., this group will have a higher mean than that group). You can choose from “greater” (Group 1 mean is higher), “less” (Group 2 mean is higher), or “two.sided” (any difference at all will work for me, thank you very much!). The default is “greater.”
- Display p-curve graph (graph)?: The function will output a figure displaying the p-curve for the results based on the initial study and the results for just those studies that (eventually) reached statistical significance (default = TRUE). More on this below.
- How many simulations do you want (sims). The number of times you want to simulate your p-hacking experiment.
To make this concrete, consider the following R code:
res <- phack(initialN=30, hackrate=5, grp1M=0, grp2M=0, grp1SD=1,
grp2SD=1, maxN=200, alpha=.05, alternative="greater", graph=TRUE, sims=1000)
This says you have planned a two-group study with N=30 (initialN=30) in each group. You are going to compute your t-test on that initial sample. If that is not statistically significant you are going to add 5 more (hackrate=5) to each group and repeat that process until it is statistically significant or you reach 200 subjects in each group (maxN=200). You have set the population Ms to both be 0 (grp1M=0; grp2M=0) with SDs of 1 (grp1SD=1; grp2SD=1). You have set your nominal alpha level to .05 (alpha=.05), specified a direction hypothesis where group 1 should be higher than group 2 (alternative=“greater”), and asked for graphical output (graph=TRUE). Finally, you have requested to run this simulation 1000 times (sims=1000).
So what happens if we run this experiment?1 So we can get the same thing, I have set the random seed in the code below.
source("http://rynesherman.com/phack.r") # read in the p-hack function
set.seed(3)
res <- phack(initialN=30, hackrate=5, grp1M=0, grp2M=0, grp1SD=1, grp2SD=1,
maxN=200, alpha=.05, alternative="greater", graph=TRUE, sims=1000)
The following output appears in R:
Proportion of Original Samples Statistically Significant = 0.054
Proportion of Samples Statistically Significant After Hacking = 0.196
Probability of Stopping Before Reaching Significance = 0.805
Average Number of Hacks Before Significant/Stopping = 28.871
Average N Added Before Significant/Stopping = 144.355
Average Total N 174.355
Estimated r without hacking 0
Estimated r with hacking 0.03
Estimated r with hacking 0.19 (non-significant results not included)
The first line tells us how many (out of the 1000 simulations) of the originally planned (N=30 in each group) studies had a p-value that was .05 or less. Because there was no true effect (grp1M = grp2M) this at just about the nominal rate of .05. But what if we had used our p-hacking scheme (testing every 5 subjects per condition until significant or N=200)? That result is in the next line. It shows that just about 20% of the time we would have gotten a statistically significant result. So this type of hacking has inflated our Type I error rate from 5% to 20%. How often would we have given up (i.e., N=200) before reaching statistical significance? That is about 80% of the time. We also averaged 28.87 “hacks” before reaching significance/stopping, averaged having to add N=144 (per condition) before significance/stopping, and had an average total N of 174 (per condition) before significance/stopping.
What about effect sizes? Naturally the estimated effect size (r) was .00 if we just used our original N=30 in each group design. If we include the results of all 1000 completed simulations that effect size averages out to be r = .03. Most importantly, if we exclude those studies that never reached statistical significance, our average effect size r = .19.
This is pretty telling. But there is more. We also get this nice picture:
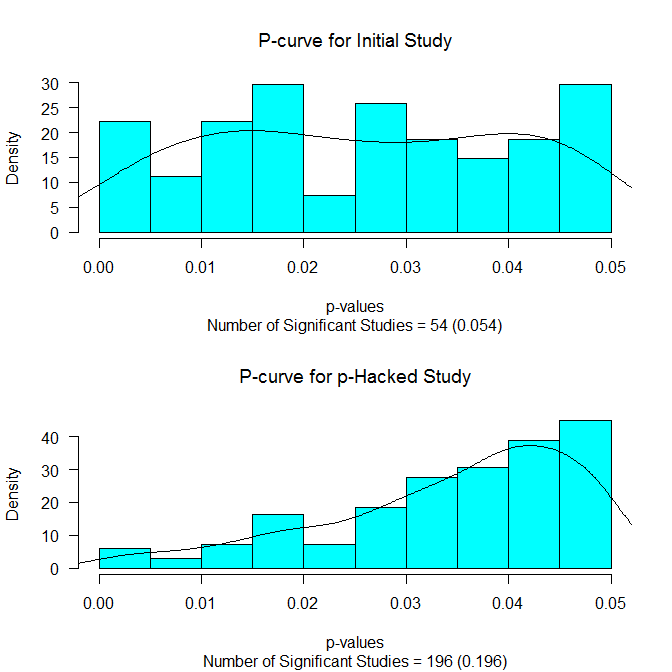
It shows the distribution of the p-values below .05 for the initial study (upper panel) and for those p-values below .05 for those reaching statistical significance. The p-curves (see Simonsohn, Nelson, & Simmons, 2013) are also drawn on. If there is really no effect, we should see a flat p-curve (as we do in the upper panel). And if there is no effect and p-hacking has occurred, we should see a p-curve that slopes up towards the critical value (as we do in the lower panel).
Finally, the function provides us with more detailed output that is summarized above. We can get a glimpse of this by running the following code:
This generates the following output:
Initial.p Hackcount Final.p NAdded Initial.r Final.r
0.86410908 34 0.45176972 170 -0.14422580 0.006078565
0.28870264 34 0.56397332 170 0.07339944 -0.008077691
0.69915219 27 0.04164525 135 -0.06878039 0.095492249
0.84974744 34 0.30702946 170 -0.13594941 0.025289555
0.28048754 34 0.87849707 170 0.07656582 -0.058508736
0.07712726 34 0.58909693 170 0.18669338 -0.011296131
The object res contains the key results from each simulation including the p-value for the initial study (Initial.p), the number of times we had to hack (Hackcount), the p-value for the last study run (Final.p), the total N added to each condition (NAdded), the effect size r for the initial study (Initial.r), and the effect size r for the last study run (Final.r).
So what can we do with this? I see lots of possibilities and quite frankly I don’t have the time or energy to do them. Here are some quick ideas:
- What would happen if there were a true effect?
- What would happen if there were a true (but small) effect?
- What would happen if we checked for significance after each subject (hackrate=1)?
- What would happen if the maxN were lower?
- What would happen if the initial sample size was larger/smaller?
- What happens if we set the alpha = .10?
- What happens if we try various combinations of these things?
I’ll admit I have tried out a few of these ideas myself, but I haven’t really done anything systematic. I just thought other people might find this function interesting and fun to play with.
1 By the way, all of these arguments are set to their default, so you can do the same thing by simply running:
Jun 25, 2014
by
EJ Wagenmakers
James Randi, magician and scientific skeptic, has compared those who believe in the paranormal to “unsinkable rubber ducks”: after a particular claim has been thoroughly debunked, the ducks submerge, only to resurface again a little later to put forward similar claims.
In light of this analogy, it comes as no surprise that Bem and colleagues have produced a new paper claiming that people can look into the future. The paper is titled “Feeling the Future: A Meta-Analysis of 90 Experiments on the Anomalous Anticipation of Random Future Events” and it is authored by Bem, Tressoldi, Rabeyron, and Duggan.
Several of my colleagues have browsed Bem's meta-analysis and have asked for my opinion. Surely, they say, the statistical evidence is overwhelming, regardless of whether you compute a p-value or a Bayes factor. Have you not changed your opinion? This is a legitimate question, one which I will try and answer below by showing you my review of an earlier version of the Bem et al. manuscript.
I agree with the proponents of precognition on one crucial point: their work is important and should not be ignored. In my opinion, the work on precognition shows in dramatic fashion that our current methods for quantifying empirical knowledge are insufficiently strict. If Bem and colleagues can use a meta-analysis to demonstrate the presence of precognition, what should we conclude from a meta-analysis on other, more plausible phenomena?
Disclaimer: the authors have revised their manuscript since I reviewed it, and they are likely to revise their manuscript again in the future. However, my main worries call into question the validity of the enterprise as a whole.
To keep this blog post self-contained, I have added annotations in italics to provide context for those who have not read the Bem et al. manuscript in detail.
My review of Bem, Tressoldi, Rabeyron, and Duggan
Read more...
Jun 18, 2014
by
Jon Grahe
As I follow the recent social media ruckus centered on replication science questioning motives and methods, it becomes clear that the open science discussion needs to consider the point made by the title of this blog; maybe repeatedly. For readers who weren’t following this, this blog by a political scientist and another post from the SPSP Blog might be of interest. I invite you to join me in evaluating this argument as the discussion progresses. I contend that “Open Science Initiatives promote Diversity, Social Justice, and Sustainability.” Replication science and registered reports are two Open Science Initiatives and by extension should also promote these ideals. If this is not true, I will abandon this revolution and go back to the status quo. However, I am confident that when considering all the evidence, you will agree with me that these idealistic principles benefit from openness generally and by open science specifically.
Before suggesting specific mechanisms by which this occurs, I will briefly note that the definitions of Open Science, Diversity, Social Justice, and Sustainability that are listed on Wikipedia are sufficient for this discussion since Wikipedia itself is an Open Science initiative. Also, I would like to convey the challenge of advancing each of these simultaneously. My own institution, Pacific Lutheran University (PLU), in our recent long range plan, PLU2020, highlighted the importance of uplifting each of these at our own institution as introduced on page 11, “As we discern our commitments for the future, we reaffirm the ongoing commitments to diversity, sustainability, and justice that already shape our contemporary identity, and we resolve to integrate these values ever more intentionally into our mission and institution.” This is easier said than done because at times the goals of these ideals sometimes conflict. For instance, the environmental costs of feeding billions of people and heating their homes are enormous. Sometimes valuing diversity (such as scholarships targeted for people of color) seems unjust because resources are being assigned unevenly. These tensions can be described with many examples across numerous goals in all three dimensions and highlight the need to make balanced decisions.
PLU has not yet resolved this challenge in uplifting all three simultaneously, but I hope that we succeed as we continue the vigorous discussion. Why each is important should be considered from is a Venn diagram on the sustainability Wikipedia page showing sustainable development as intersections between three pillars of sustainable development, social (people), economic, and environmental because even sustainability itself represents competing interests. Diversity and Social Justice are both core aspects of the social dimension, where uplifting diversity highlights the importance of distinct ideas and cultures and helps us understand why people and their varied ideas, in addition to oceans and forests are important resources of our planet. The ideals of social justice aim to provide mechanisms such that all members of our diverse population receive and contribute our fair share of these resources. Because resources are limited and society complex and flawed, these ideals are often more aspirational rather than practical. However, the basic premise of uplifting all three is that we are better when valuing diversity, providing social justice, and sustainably using the planet’s resources (people, animals, plants, and rocks). Below I provide examples for how OSIs promote each of these principles while illustrating why each is important to science.
Read more...
Jun 11, 2014
by
Betsy Levy Paluck
This article was originally posted on Betsy Levy Paluck's website.
On his terrific blog, Professor Sanjay Srivastava points out that the current (vitriolic) debate about replication in psychology has been "salted with casually sexist language, and historically illiterate" arguments, on both sides. I agree, and thank him for pointing this out.
I'd like to add that I believe academics participating in this debate should be mindful of co-opting powerful terms like bullying and police (e.g., the "replication police") to describe the replication movement. Why? Bullying behavior describes repeated abuse from a person of higher power and influence. Likewise, many people in the US and throughout the world have a well-grounded terror of police abuse. The terror and power inequality that these terms connote is diminished when we use it to describe the experience of academics replicating one another's studies. Let's keep evocative language in reserve so that we can use it to name and change the experience of truly powerless and oppressed people.
Back to replication. Here is the thing: we all believe in the principle of replication. As scientists and as psychologists, we are all here because we wish to contribute to cumulative research that makes progress on important psychological questions. This desire unites us.
So what's up?
It seems to me that some people oppose the current wave of replication efforts because they do not like the tenor of the recent public discussions. As I already mentioned, neither do I. I'm bewildered by the vitriol. Just a few days ago, one of the most prominent modern economists, currently an internationally bestselling author, had his book called into question over alleged data errors in a spreadsheet that he made public. His response was cordial and curious; his colleagues followed up with care, equanimity, and respect.
Are we really being taught a lesson in manners from economists? Is that happening?
As one of my favorite TV characters said recently ...
If we don't like the tenor of the discussion about replication, registration, etc., let's change it.
In this spirit, I offer a brief description of what we are doing in my lab to try to make our social science rigorous, transparent, and replicable. It's one model for your consideration, and we are open to suggestions.
For the past few years we have registered analysis plans for every new project we start. (They can be found here on the EGAP website; this is a group to which I belong. EGAP has had great discussions in partnership with BITSS about transparency.) My lab's analysis registrations are accompanied by a codebook describing each variable in the dataset.
I am happy to say that we are just starting to get better at producing replication code and data & file organization that is sharing-ready as we do the research, rather than trying to reconstruct these things from our messy code files and Dropbox disaster areas following publication (for this, I thank my brilliant students, who surpass me with their coding skills and help me to keep things organized and in place. See also this). What a privilege and a learning experience to have graduate students, right? Note that they are listening to us have this debate.
Margaret Tankard, Rebecca Littman, Graeme Blair, Sherry Wu, Joan Ricart-Huguet, Andreana Kenrick (awesome grad students), and Robin Gomila and David Mackenzie (awesome lab managers) have all been writing analysis registrations, organizing files, checking data codebooks, and writing replication code for the experiments we've done in the past three years, and colleagues Hana Shepherd, Peter Aronow, Debbie Prentice, and Eldar Shafir are doing the same with me. Thank goodness for all these amazing and dedicated collaborators, because one reason I understand replication to be so difficult is that it is a huge challenge to reconstruct what you thought and did over a long period of time, without careful record keeping (note: analysis registration also serves that purpose for us!).
Previously, I posted data at Yale's ISPS archive, and for other datasets made them available on request if I thought I was going to work more on them. But in future we plan to post all published data plus the dataset's codebook. Economist and political scientists friends often post to their personal websites. Another possibility is posting in digital archives (like Yale's, but there are others: I follow @annthegreen for updates on digital archiving).
I owe so much of my appreciation for these practices to my advisor Donald Green. I've also learned a lot from Macartan Humphreys.
I'm interested in how we can be better. I'm listening to the constructive debates and to the suggestions out there. If anyone has questions about our current process, please leave a comment below! I'd be happy to answer questions, provide examples, and to take suggestions.
It costs nothing to do this--but it slows us down. Slowing down is not a bad thing for research (though I recognize that a bad heuristic of quantity = quality still dominates our discipline). During registration, we can stop to think-- are we sure we want to predict this? With this kind of measurement? Should we go back to the drawing board about this particular secondary prediction? I know that if I personally slow down, I can oversee everything more carefully. I'm learning how to say no to new and shiny projects.
I want to end on the following note. I am now tenured. If good health continues, I'll be on hiring committees for years to come. In a hiring capacity, I will appreciate applicants who, though they do not have a ton of publications, can link their projects to an online analysis registration, or have posted data and replication code. Why? I will infer that they were slowing down to do very careful work, that they are doing their best to build a cumulative science. I will also appreciate candidates who have conducted studies that "failed to replicate" and who responded to those replication results with follow up work and with thoughtful engagement and curiosity (I have read about Eugene Caruso's response and thought that he is a great model of this kind of response).
I say this because it's true, and also because some academics report that their graduate students are very nervous about how replication of their lab's studies might ruin their reputations on the job market (see Question 13). I think the concern is understandable, so it's important for those of us in these lucky positions to speak out about what we value and to allay fears of punishment over non-replication (see Funder: SERIOUSLY NOT OK).
In sum, I am excited by efforts to improve the transparency and cumulative power of our social science. I'll try them myself and support newer academics who engage in these practices. Of course, we need to have good ideas as well as good research practices (ugh--this business is not easy. Tell that to your friends who think that you've chosen grad school as a shelter from the bad job market).
I encourage all of my colleagues, and especially colleagues from diverse positions in academia and from underrepresented groups in science, to comment on what they are doing in their own research and how they are affected by these ideas and practices. Feel free to post below, post on (real) blogs, write letters to the editor, have conversations in your lab and department, or tweet. I am listening. Thanks for reading.
*
A collection of comments I've been reading about the replication debate, in case you haven't been keeping up. Please do post more links below, since this isn't comprehensive.
I'm disappointed: a graduate student's perspective
Does the replication debate have a diversity problem?
Replications of Important Results in Social Psychology: Special Issue of Social Psychology
The perilous plight of the (non)-replicator
"Replication Bullying": Who replicates the replicators?
Rejoinder to Schnall (2014) in Social Psychology
Context and Correspondence for Special Issue of Social Psychology
Behavioral Priming: Time to Nut Up or Shut Up
Tweets: